Adaptive Iterative Learning Control for Nonsquare Nonlinear Systems With Various Nonrepetitive Uncertainties: A Unified Approach
IEEE TRANSACTIONS ON AUTOMATIC CONTROL(2024)
摘要
This work concerns adaptive iterative learning control (AILC) for nonsquare multiple-input--multiple-output (MIMO) nonlinear systems. With newly introduced techniques in dealing with the nonsquare input distribution matrix, a novel robust AILC (RAILC) approach is proposed. The method is not only effective to address the perfect trajectory tracking problem for both underactuated or overactuated systems, but also shows impressive robustness and adaptiveness to various system uncertainties and external disturbances, including matched and mismatched, parametric and nonparametric as well as input distribution uncertainties. Inspired by the proposed RAILC design framework, a new unified AILC algorithm is then developed for a generic class of uncertain MIMO nonlinear systems with either square or nonsquare input distribution matrices. The work is novel, which contributes significantly not only to refine the theory of AILC but also to widen the application scope. The convergence properties of the proposed control algorithms are analyzed rigorously under the framework of composite energy function methodology, and their effectiveness have been verified through two illustrated examples.
更多查看译文
关键词
Uncertainty,Nonlinear systems,MIMO communication,Convergence,Trajectory tracking,Iterative learning control,Adaptive control,Adaptive iterative learning control (AILC),composite energy function (CEF),convergence analysis,nonsquare systems,trajectory tracking
AI 理解论文
溯源树
样例
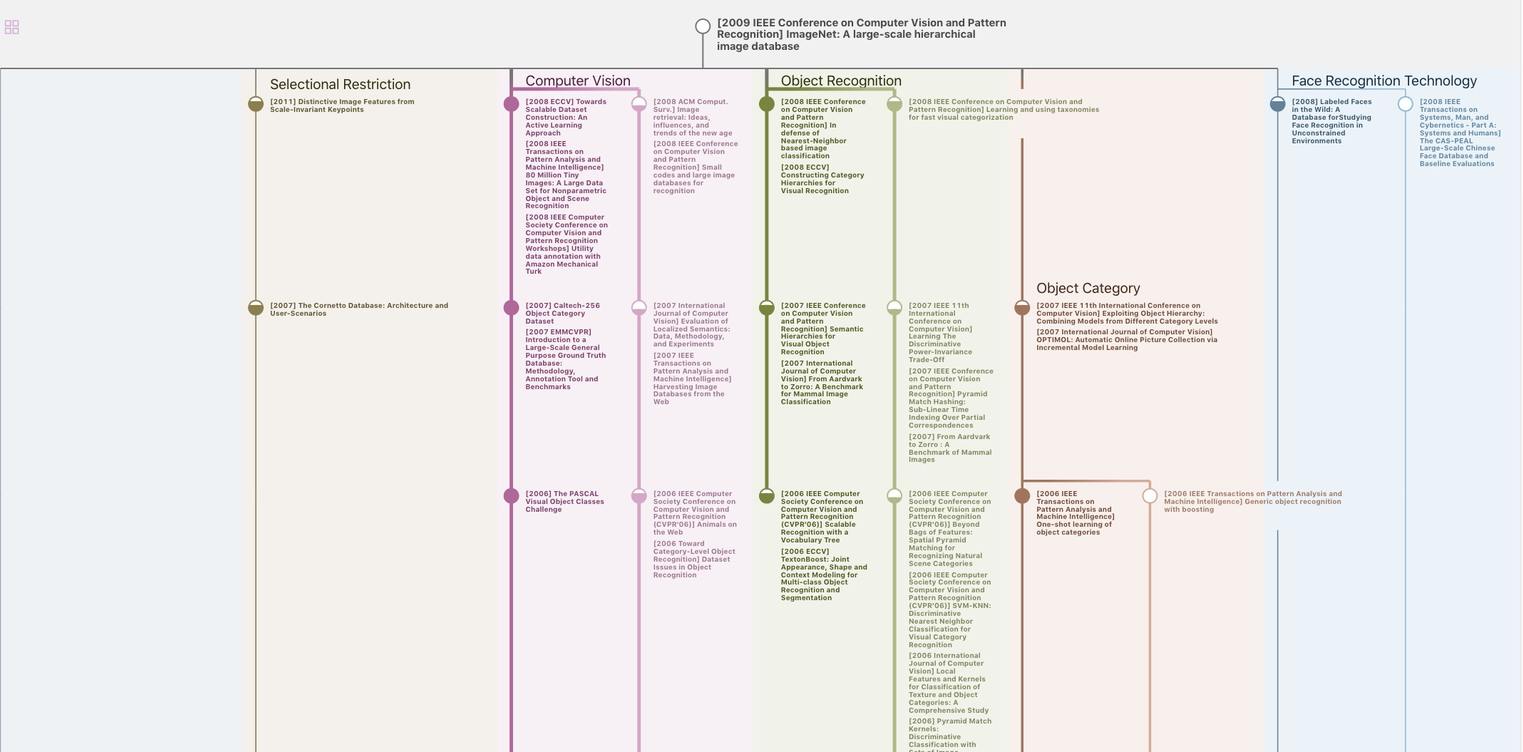
生成溯源树,研究论文发展脉络
Chat Paper
正在生成论文摘要