Temporal Super-Resolution, Ground Adjustment, and Advection Correction of Radar Rainfall Using 3-D-Convolutional Neural Networks
IEEE TRANSACTIONS ON GEOSCIENCE AND REMOTE SENSING(2024)
摘要
Weather radars are highly sophisticated tools for quantitative precipitation estimation (QPE) and provide observations with unmatched spatial representativeness. However, their indirect measurement of precipitation high above ground leads to strong systematic errors compared to direct rain gauge measurements. Additionally, the temporal undersampling from 5-min instantaneous radar measurements requires advection correction. We present ResRadNet, a 3-D-convolutional residual neural network approach, to reduce these errors and, at the same time, increase the temporal resolution of the radar rainfall fields by a 5-min short-range prediction of 1-min time-steps. The network is trained to process spatiotemporal sequences of radar rainfall estimates from a composite product derived from 17 C-band weather radars in Germany. In contrast to previous approaches, we present a method that emphasizes the generation of spatiotemporally consistent and advection-corrected country-wide rainfall maps. Our approach significantly increased the Pearson correlation coefficient (PCC) of the radar product (from 0.63 to 0.74) and decreased the root mean squared error (mse) by 22% when compared to 247 rain gauges at a 5-min resolution. An additional large-scale comparison to eight years of data from 1138 independent manual daily gauges confirmed that the improvement is robust and transferable to new locations. Overall, our study shows the benefits of using 3-D convolutional neural networks (CNNs) for weather radar rainfall estimation to provide 1-min, ground-adjusted, that is, bias-corrected with respect to on-ground sensors, and advection-corrected radar rainfall estimates.
更多查看译文
关键词
Convolutional neural network (CNN),deep learning,precipitation,residual neural network,weather radar
AI 理解论文
溯源树
样例
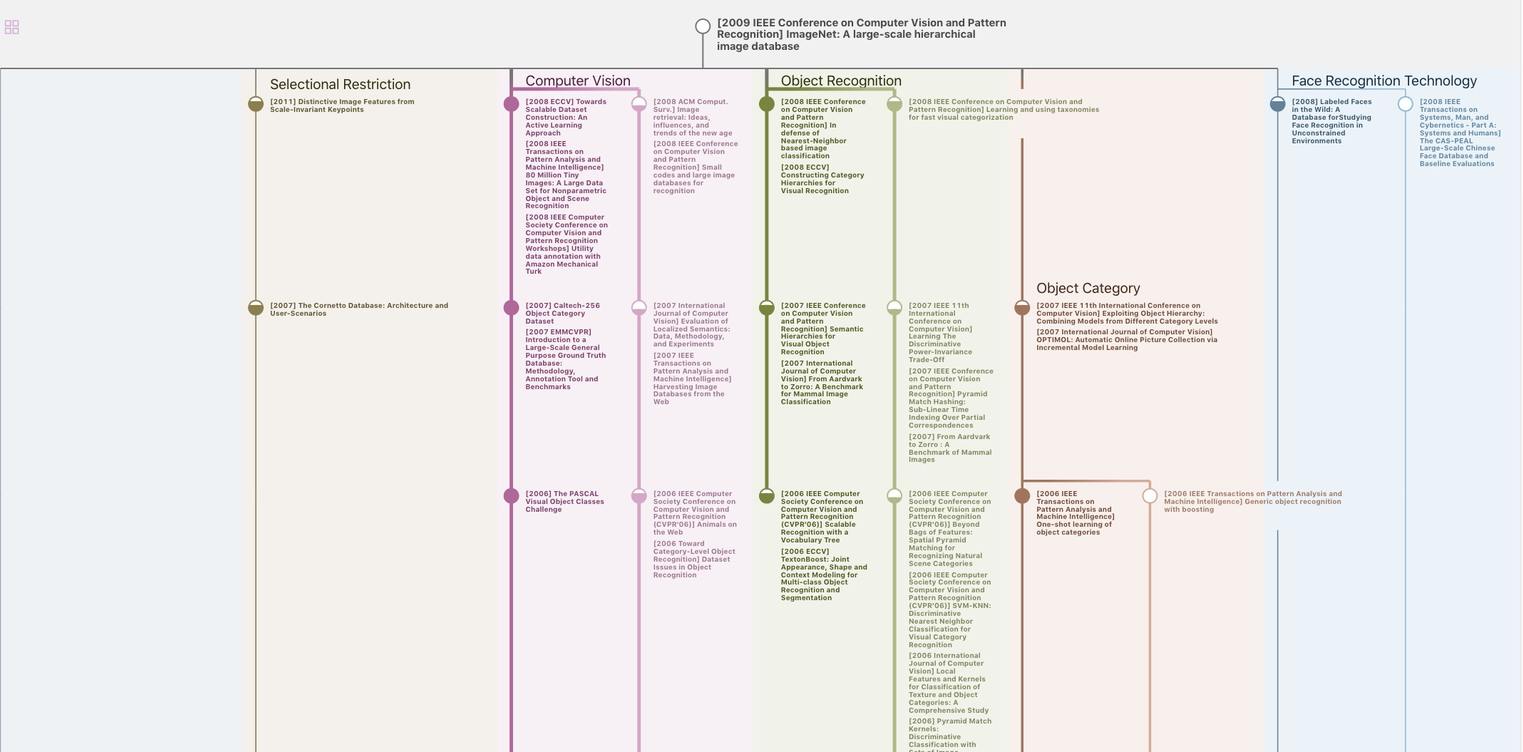
生成溯源树,研究论文发展脉络
Chat Paper
正在生成论文摘要