UGLS: an uncertainty guided deep learning strategy for accurate image segmentation
FRONTIERS IN PHYSIOLOGY(2024)
摘要
Accurate image segmentation plays a crucial role in computer vision and medical image analysis. In this study, we developed a novel uncertainty guided deep learning strategy (UGLS) to enhance the performance of an existing neural network (i.e., U-Net) in segmenting multiple objects of interest from images with varying modalities. In the developed UGLS, a boundary uncertainty map was introduced for each object based on its coarse segmentation (obtained by the U-Net) and then combined with input images for the fine segmentation of the objects. We validated the developed method by segmenting optic cup (OC) regions from color fundus images and left and right lung regions from Xray images. Experiments on public fundus and Xray image datasets showed that the developed method achieved a average Dice Score (DS) of 0.8791 and a sensitivity (SEN) of 0.8858 for the OC segmentation, and 0.9605, 0.9607, 0.9621, and 0.9668 for the left and right lung segmentation, respectively. Our method significantly improved the segmentation performance of the U-Net, making it comparable or superior to five sophisticated networks (i.e., AU-Net, BiO-Net, AS-Net, Swin-Unet, and TransUNet).
更多查看译文
关键词
deep learning,training strategy,image segmentation,fundus image,optic cup deep learning,optic cup
AI 理解论文
溯源树
样例
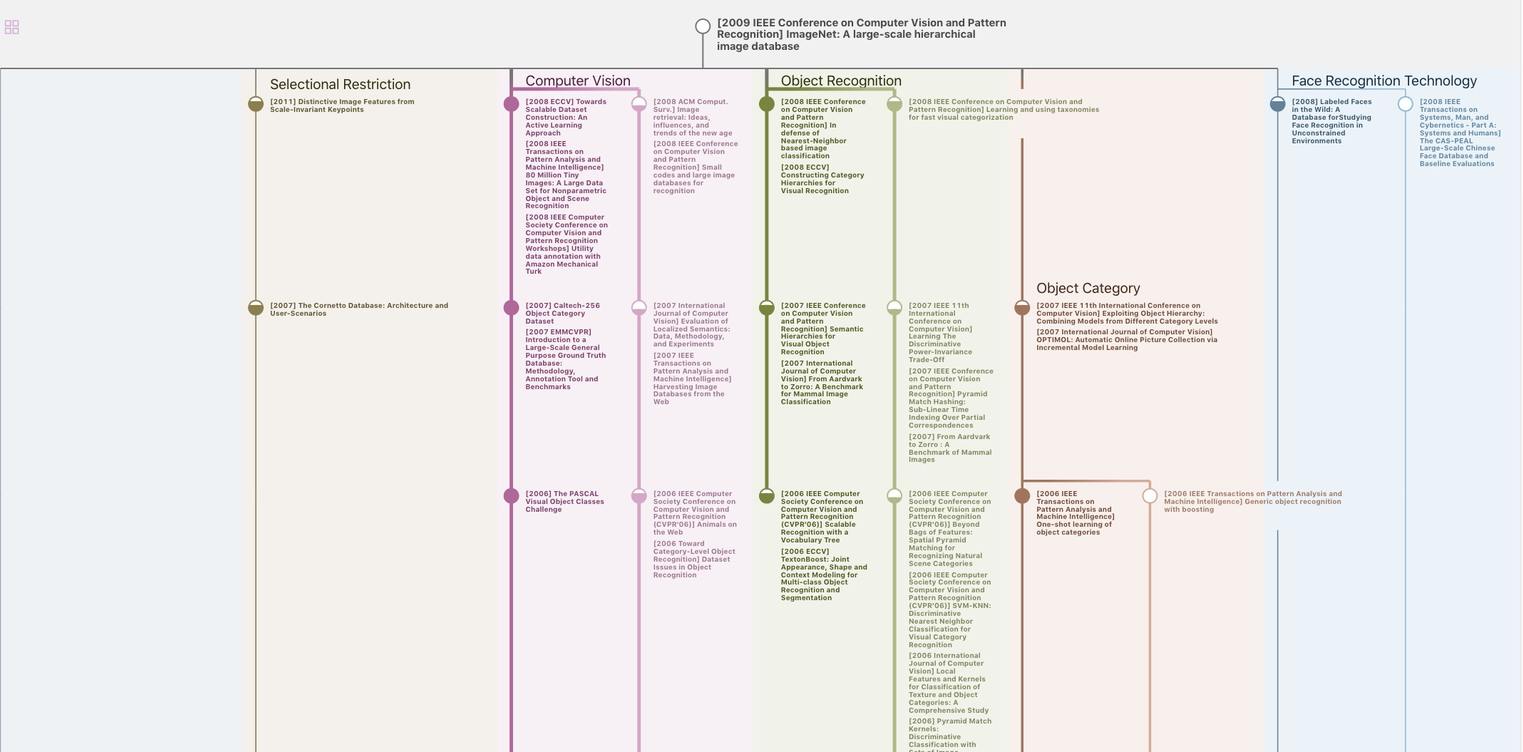
生成溯源树,研究论文发展脉络
Chat Paper
正在生成论文摘要