Evaluation of the prediction effectiveness for geochemical mapping using machine learning methods: A case study from northern Guangdong Province in China
Science of The Total Environment(2024)
摘要
This study compares seven machine learning models to investigate whether they improve the accuracy of geochemical mapping compared to ordinary kriging (OK). Arsenic is widely present in soil due to human activities and soil parent material, posing significant toxicity. Predicting the spatial distribution of elements in soil has become a current research hotspot. Lianzhou City in northern Guangdong Province, China, was chosen as the study area, collecting a total of 2908 surface soil samples from 0 to 20 cm depth. Seven machine learning models were chosen: Random Forest (RF), Support Vector Machine (SVM), Ridge Regression (Ridge), Gradient Boosting Decision Tree (GBDT), Artificial Neural Network (ANN), K-Nearest Neighbors (KNN), and Gaussian Process Regression (GPR). Exploring the advantages and disadvantages of machine learning and traditional geological statistical models in predicting the spatial distribution of heavy metal elements, this study also analyzes factors affecting the accuracy of element prediction. The two best-performing models in the original model, RF (R2 = 0.445) and GBDT (R2 = 0.414), did not outperform OK (R2 = 0.459) in terms of prediction accuracy. Ridge and GPR, the worst-performing methods, have R2 values of only 0.201 and 0.248, respectively. To improve the models' prediction accuracy, a spatial regionalized (SR) covariate index was added. Improvements varied among different methods, with RF and GBDT increasing their R2 values from 0.4 to 0.78 after enhancement. In contrast, the GPR model showed the least significant improvement, with its R2 value only reaching 0.25 in the improved method. This study concluded that choosing the right machine learning model and considering factors that influence prediction accuracy, such as regional variations, the number of sampling points, and their distribution, are crucial for ensuring the accuracy of predictions. This provides valuable insights for future research in this area.
更多查看译文
关键词
Machine learning,Heavy metals,Kriging interpolation,Accurate prediction
AI 理解论文
溯源树
样例
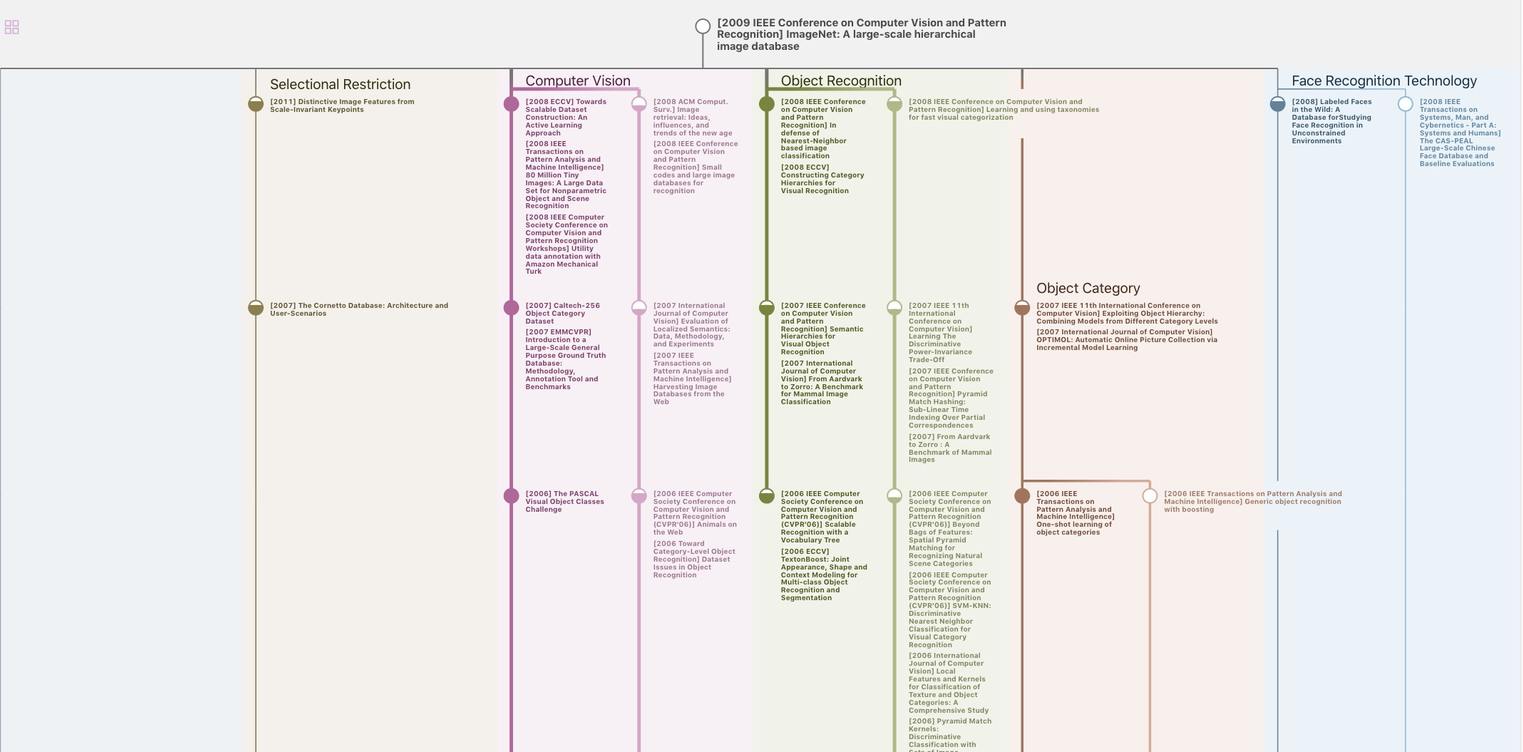
生成溯源树,研究论文发展脉络
Chat Paper
正在生成论文摘要