Machine learning based on optimal VOI of multi-sequence MR images to predict lymphovascular invasion in invasive breast cancer
Heliyon(2024)
摘要
Objectives
Lymphovascular invasion serves as a crucial prognostic indicator in invasive breast cancer, influencing treatment decisions. We aimed to develop a machine learning model utilizing optimal volumes of interest extracted from multisequence magnetic resonance images to predict lymphovascular invasion in patients with invasive breast cancer.
Materials and methods
This study comprised 191 patients postoperatively diagnosed with invasive breast cancer through multi-sequence magnetic resonance imaging. Independent predictors were identified through univariate and multivariate logistic regression analyses, culminating in the construction of a clinical model. Radiomic features were extracted from multi-sequence magnetic resonance imaging images across various volume of interest scales (−2 mm, entire, +2 mm, +4 mm, and +6 mm). Subsequently, various radiomic models were developed using machine learning model algorithms, including logistic regression, support vector machine, k-nearest neighbor, gradient boosting machine, classification and regression tree, and random forest. A hybrid model was then formulated, amalgamating optimal radiomic and clinical models.
Results
The area under the curve of the clinical model was 0.757. Among the radiomic models, the most efficient diagnosis was achieved by the k-nearest neighbor-based radiomics-volume of interest (+2 mm), resulting in an area under the curve of 0.780. The hybrid model, integrating the k-nearest neighbor-based radiomics-volume of interest (+2 mm), and the clinical model surpassed the individual clinical and radiomics models, exhibiting a superior area under the curve of 0.864.
Conclusion
Utilizing a hybrid approach integrating clinical data and multi-sequence magnetic resonance imaging-derived radiomics models based on the multiscale tumor region volume of interest (+2 mm) proved effective in determining lymphovascular invasion status in patients with invasive breast cancer. This innovative methodology may offer valuable insights for treatment planning and disease management.
更多查看译文
关键词
Invasive breast cancer,Lymphovascular invasion,Multi-sequence MRI,Machine learning,Volumes of interest
AI 理解论文
溯源树
样例
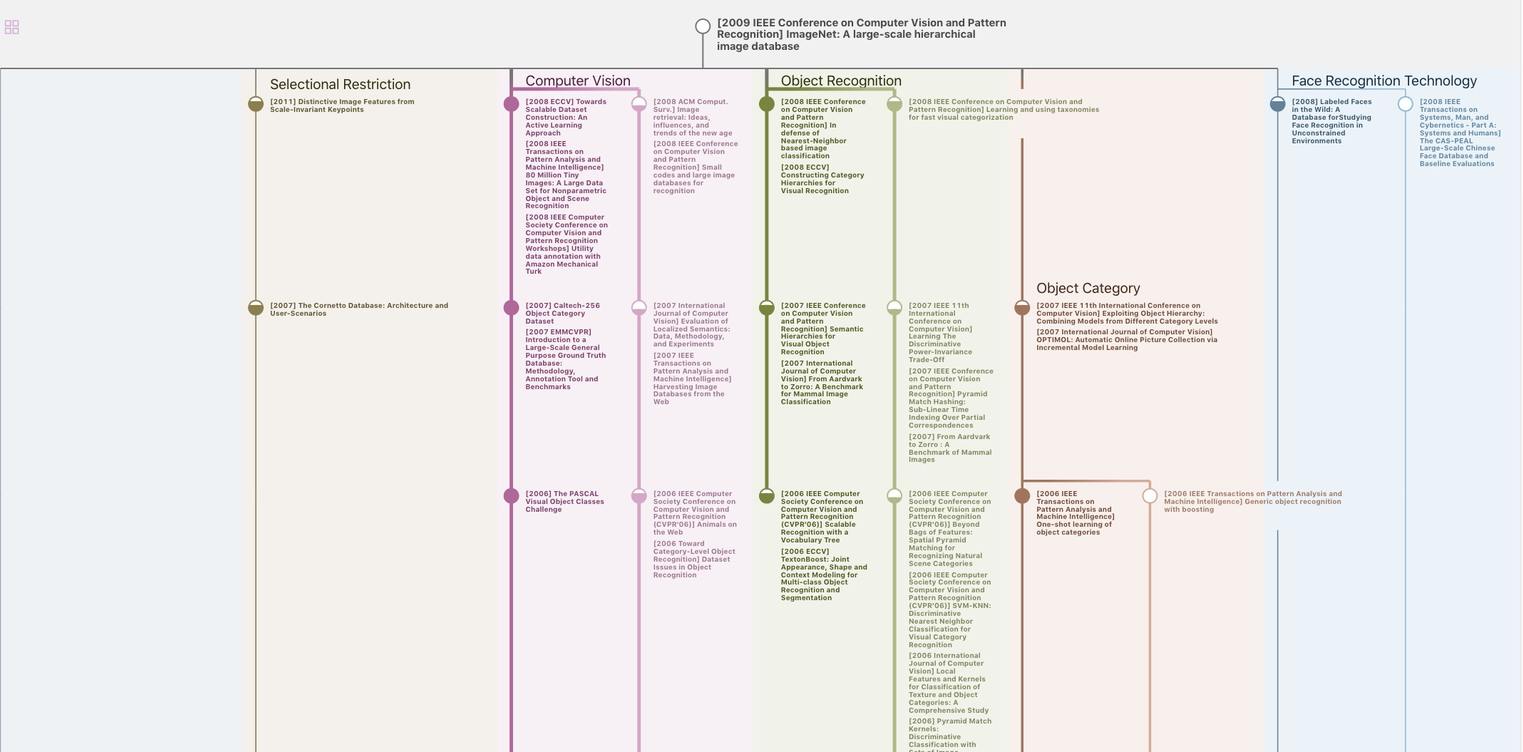
生成溯源树,研究论文发展脉络
Chat Paper
正在生成论文摘要