A novel framework for high resolution air quality index prediction with interpretable artificial intelligence and uncertainties estimation
Journal of Environmental Management(2024)
摘要
Accurate air quality index (AQI) prediction is essential in environmental monitoring and management. Given that previous studies neglect the importance of uncertainty estimation and the necessity of constraining the output during prediction, we proposed a new hybrid model, namely TMSSICX, to forecast the AQI of multiple cities. Firstly, time-varying filtered based empirical mode decomposition (TVFEMD) was adopted to decompose the AQI sequence into multiple internal mode functions (IMF) components. Secondly, multi-scale fuzzy entropy (MFE) was applied to evaluate the complexity of each IMF component and clustered them into high and low-frequency portions. In addition, the high-frequency portion was secondarily decomposed by successive variational mode decomposition (SVMD) to reduce volatility. Then, six air pollutant concentrations, namely CO, SO2, PM2.5, PM10, O3, and NO2, were used as inputs. The secondary decomposition and preliminary portion were employed as the outputs for the bidirectional long short-term memory network optimized by the snake optimization algorithm (SOABiLSTM) and improved Catboost (ICatboost), respectively. Furthermore, extreme gradient boosting (XGBoost) was applied to ensemble each predicted sub-model to acquire the consequence. Ultimately, we introduced adaptive kernel density estimation (AKDE) for interval estimation. The empirical outcome indicated the TMSSICX model achieved the best performance among the other 23 models across all datasets. Moreover, implementing the XGBoost to ensemble each predicted sub-model led to an 8.73%, 8.94%, and 0.19% reduction in RMSE, compared to SVM. Additionally, by utilizing SHapley Additive exPlanations (SHAP) to assess the impact of the six pollutant concentrations on AQI, the results reveal that PM2.5 and PM10 had the most notable positive effects on the long-term trend of AQI. We hope this model can provide guidance for air quality management.
更多查看译文
关键词
AQI prediction,Improved catboost,Time-varying filtered based empirical mode decomposition,XGBoost,SHAP
AI 理解论文
溯源树
样例
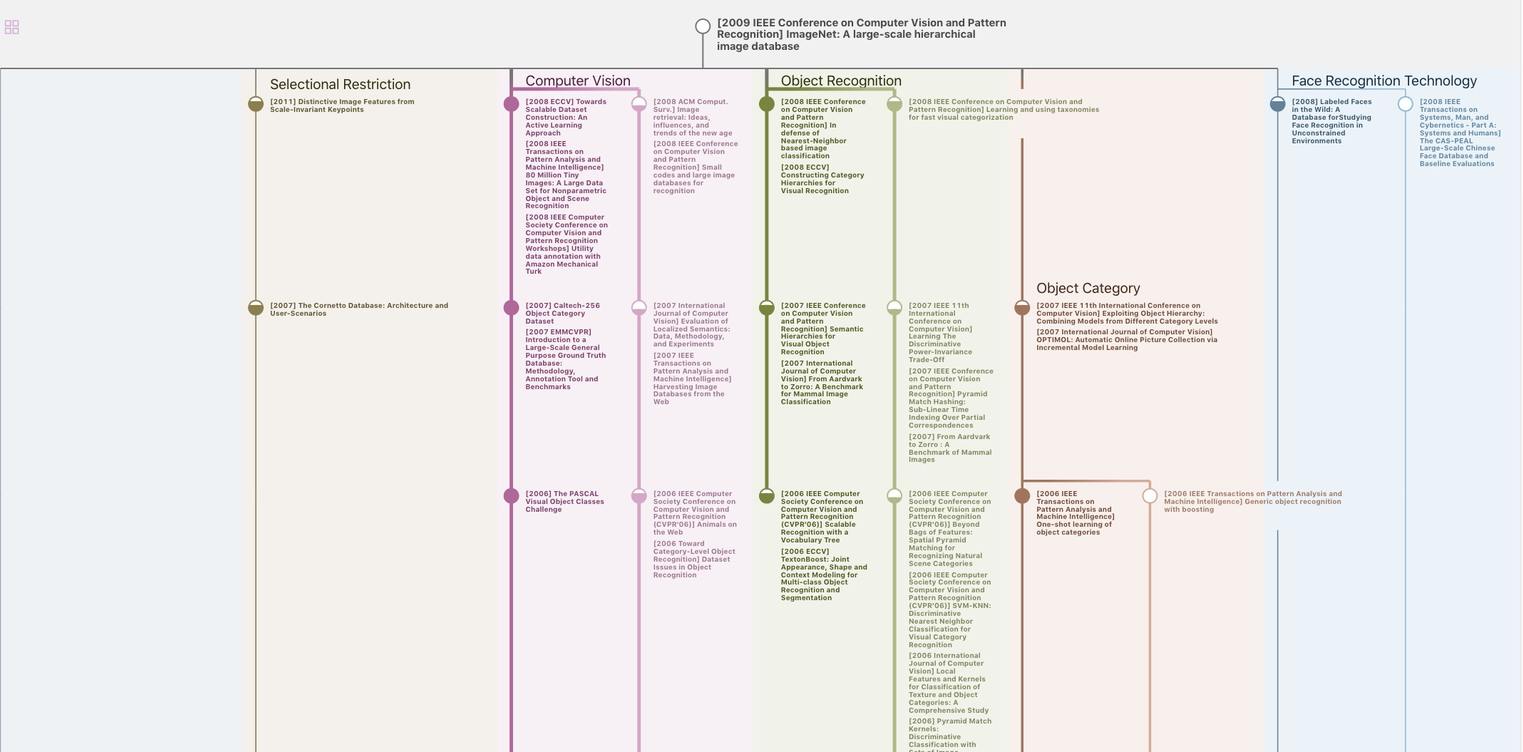
生成溯源树,研究论文发展脉络
Chat Paper
正在生成论文摘要