Cross-modal Recipe Retrieval with Fine-grained Prompting Alignment and Evidential Semantic Consistency
IEEE Transactions on Multimedia(2024)
摘要
Alignment between the food images and the corresponding recipes is an emerging cross-modal representation learning task. In this task, the recipes are composed of three components, i.e., food title, ingredient lists, and cooking instructions, which require a fine-grained alignment between the features of the two modalities. Existing methods usually aggregate the recipes into global embeddings and then align them with the global image embeddings. Meanwhile, semantic classification is frequently used in these methods to regularize the embeddings of the two modalities. While these methods are efficient, there remain two problems: (1) Forcing the alignment between the global images and recipes embeddings may result in losing the component-specific information. (2) The high diversity of food appearance leads to high uncertainty in the semantic classification of food images and recipes. To solve these problems, we propose a Fine-grained Prompting and Alignment (FPA) model to enhance the feature extraction and bring more component-specific information for fine-grained alignment. Furthermore, to regularize the semantic information contained in the cross-modal features, we design an Evidential Semantic Consistency (ESC) loss to keep the cross-modal semantic consistency. We have conducted comprehensive experiments on the benchmark dataset Recipe1M and the state-of-the-art results on the cross-modal recipe retrieval task demonstrate the effectiveness of our method.
更多查看译文
关键词
Cross-modal recipe retrieval,Prompt learning,Evidential deep learning
AI 理解论文
溯源树
样例
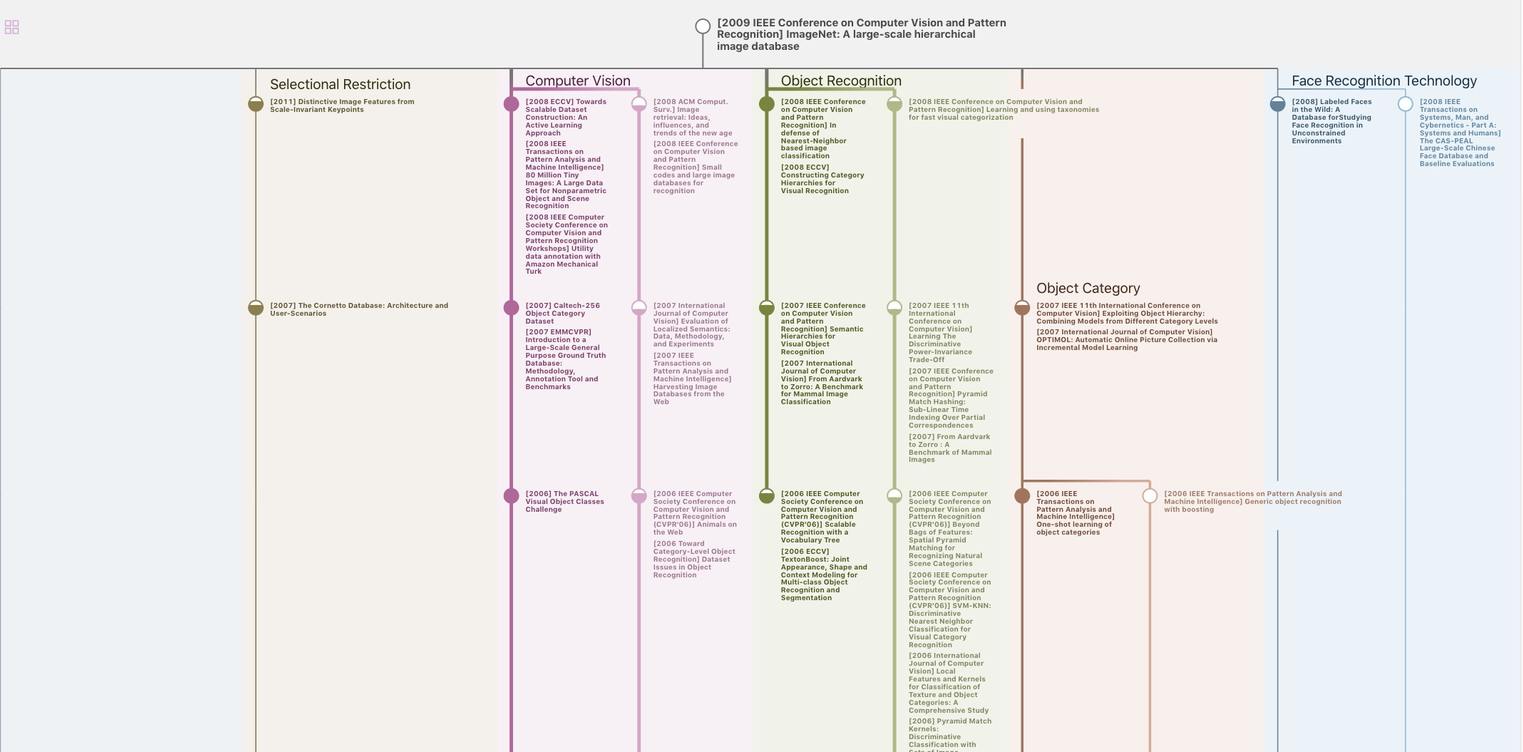
生成溯源树,研究论文发展脉络
Chat Paper
正在生成论文摘要