Input Agnostic Trojan Attack for Deep Learning-Based Wireless Signal Classification.
National Conference on Communications(2024)
摘要
Deep learning (DL) is gaining prominence in various wireless communications applications, including modulation classification, channel estimation, optimal power allocation, etc. Nevertheless, DL is susceptible to data poisoning-based attacks (Trojan/backdoor attacks), where carefully designed imperceptible triggers are introduced to the training dataset, causing models to make erroneous predictions. We propose a novel Trojan attack technique for wireless modulation classification, where the adversary finds linearly independent and orthonormal triggers using the Gram-Schmidt orthogonalization method. The triggers are also input agnostic, making them generalizable across different signals. During the training phase, a small amount of clean samples are poisoned by adding these triggers and changing the labels to a desired target label. We show that in the deployment stage, the DL-based classifier (poisoned) at the receiver correctly classifies the clean (trigger-free) signals with high accuracy. However, it misclassifies the wireless signals with the trigger to the desired target label, which differs from the true label with a high attack success rate. Finally, we propose an activation clustering-based detection technique to determine the presence of poisoned samples in the dataset and found to be effective against the proposed Trojan attack method.
更多查看译文
关键词
Adversarial machine learning,deep learning,modulation classification,Trojan attack,wireless security
AI 理解论文
溯源树
样例
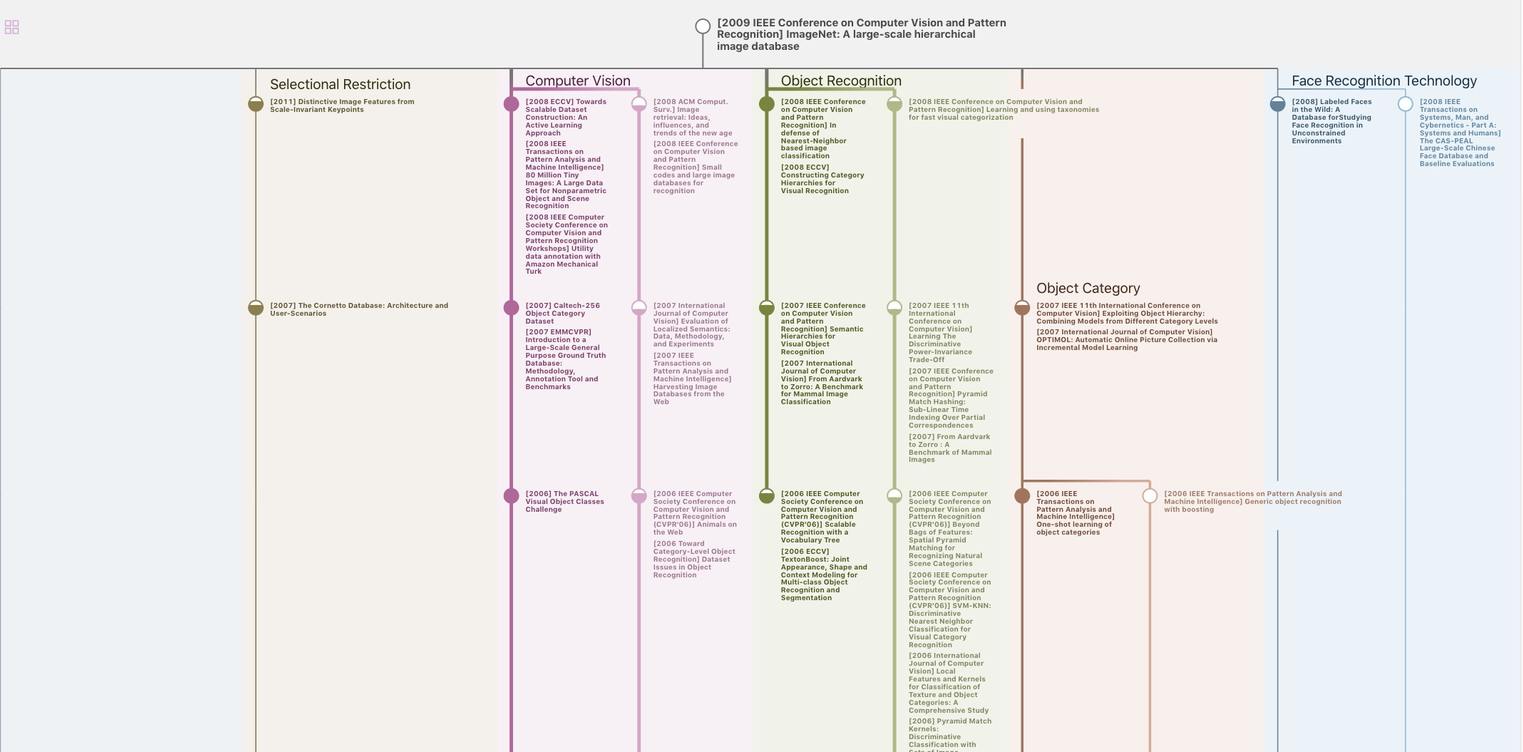
生成溯源树,研究论文发展脉络
Chat Paper
正在生成论文摘要