Global Heterogeneous Graph and Target Interest Denoising for Multi-behavior Sequential Recommendation
PROCEEDINGS OF THE 17TH ACM INTERNATIONAL CONFERENCE ON WEB SEARCH AND DATA MINING, WSDM 2024(2024)
摘要
Multi-behavior sequential recommendation (MBSR) predicts a user's next item of interest based on their interaction history across different behavior types. Although existing studies have proposed capturing the correlation between different types of behavior, two important challenges have not been explored: i) Dealing with heterogeneous item transitions (both global and local perspectives). ii) Mitigating the issue of noise that arises from the incorporation of auxiliary behaviors. To address these issues, we propose a novel solution, Global Heterogeneous Graph and Target Interest Denoising for Multi-behavior Sequential Recommendation (GHTID). In particular, we view the transitions between behavior types of items as different relationships and propose two heterogeneous graphs. By considering the relationship between items under different behavioral types of transformations, we propose two heterogeneous graph convolution modules and explicitly learn heterogeneous item transitions. Moreover, we utilize two attention networks to integrate long-term and short-term interests associated with the target behavior to alleviate the noisy interference of auxiliary behaviors. Extensive experiments on four real-world datasets demonstrate that our method outperforms other state-of-the-art methods.
更多查看译文
关键词
Multi-Behavior Sequential Recommendation,Graph Neural Network,User Interest Denosing
AI 理解论文
溯源树
样例
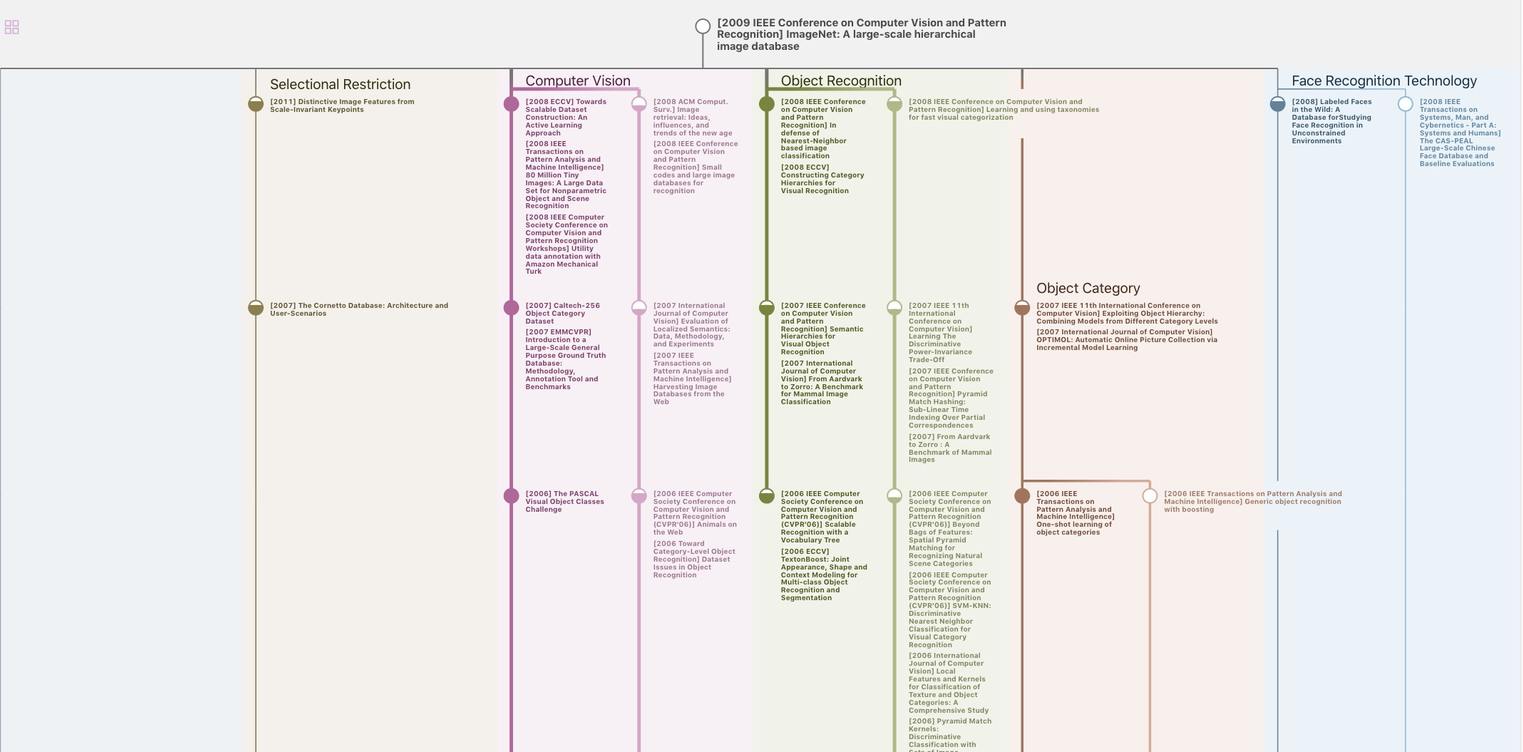
生成溯源树,研究论文发展脉络
Chat Paper
正在生成论文摘要