A Modified Feature Selection Algorithm for Intrusion Detection System Based on Student Psychology-Based Optimization with Explainable AI
2023 IEEE Asia-Pacific Conference on Computer Science and Data Engineering (CSDE)(2023)
摘要
Intrusion Detection Systems (IDS) play a vital role in safeguarding network security by monitoring and identifying both normal and intrusive activities. However, constructing an effective IDS from extensive datasets poses a significant challenge. The process of feature selection from these datasets is crucial, as it not only enhances accuracy but also reduces the time required to build the model. In this paper, we employ a modified version of the Student Psychology-Based Optimization (SPBO) algorithm to identify the most relevant features set for IDS. Following feature selection, various machine learning algorithms are employed for multi-level classification. To evaluate our approach, we utilize the NLS-KDD dataset. Our proposed algorithm surpasses several state-of-the-art feature selection methods in terms of accuracy, precision, recall, Fl-score, and finally faster convergence. Specif-ically, our proposed bio-inspired SPBO algorithm combined with the Random Forest (RF) algorithm achieves a comparable accuracy rate of 99.81 %. To ensure better comprehensibility, we employ two widely used Explainable Artificial Intelligence (XAI) techniques: SHapley Additive exPlanations (SHAP) and Local In-terpretable Model-Agnostic Explanations (LIME). These methods provide insightful explanations for our model's decisions, further enhancing its interpretability.
更多查看译文
关键词
Intrusion Detection System,Security,Machine Learning,Feature Selection,Optimization,Artificial intelligence
AI 理解论文
溯源树
样例
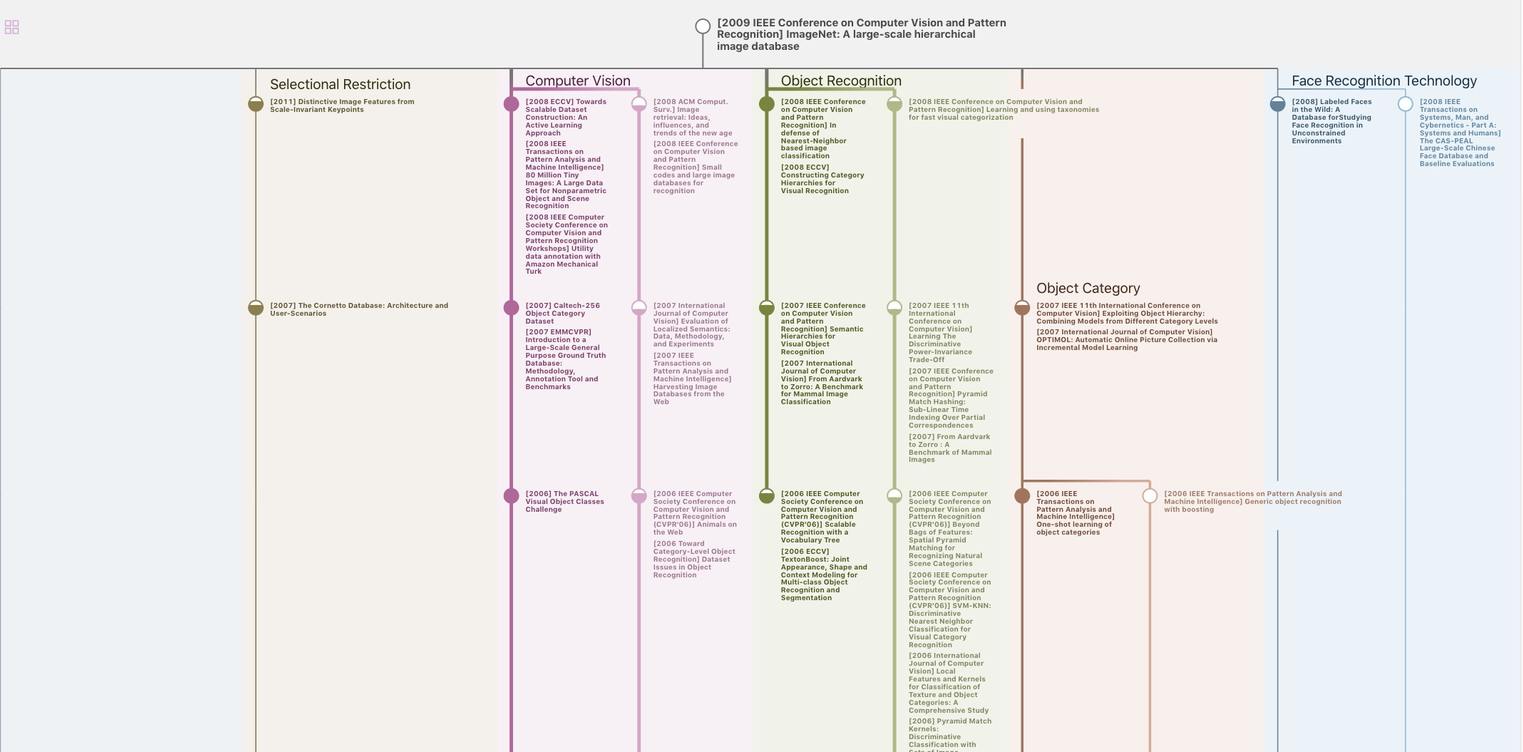
生成溯源树,研究论文发展脉络
Chat Paper
正在生成论文摘要