STRmt: A state transition based model for real‐time crowd counting in a metro system
Concurrency and Computation: Practice and Experience(2024)
摘要
SummaryReal‐time estimation of crowd counting in underground metro systems, constrained by limited space, is crucial for managing heightened pedestrian volumes and responding promptly to emergencies. To address this challenge, we propose a passenger state transition‐based model, called STRmt, designed for the seamless and continuous monitoring of real‐time crowd movement within service areas of stations and trains, leveraging auto fare collection systems (AFC) as a comprehensive sensor network. Our innovation lies in modeling the dynamic movement of passengers within a metro system over time as a state transition process aligned with the train schedule. To achieve this, we introduce a spatio‐temporal deep learning framework, denoted as STnet, designed to dynamically predict these state transitions. The performance of our method is rigorously assessed through extensive experiments conducted spanning 2 years in Shenzhen, China, utilizing AFC data, train schedule data, and weather data. The results demonstrate that the proposed method surpasses baseline methods, achieving an estimation precision of 0.92.
更多查看译文
AI 理解论文
溯源树
样例
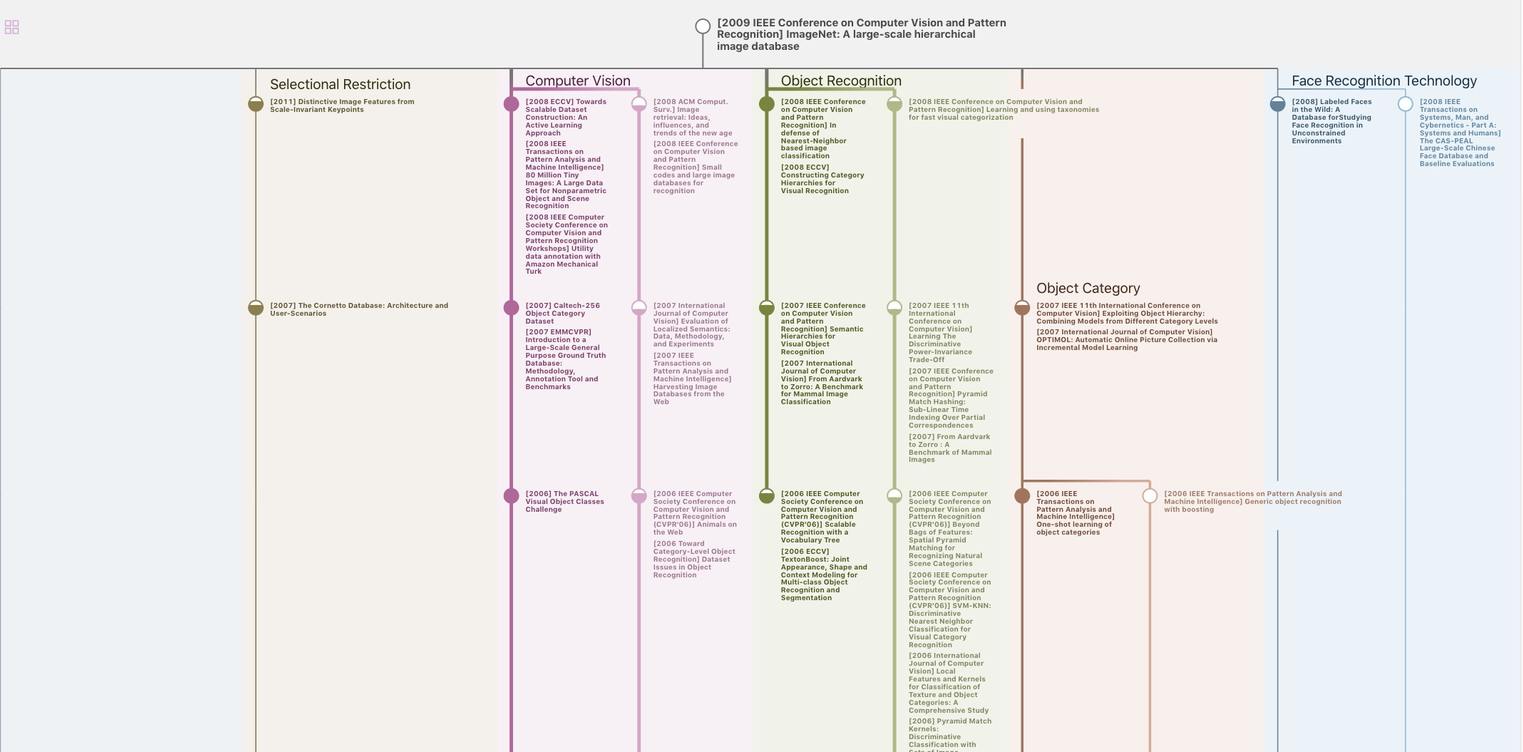
生成溯源树,研究论文发展脉络
Chat Paper
正在生成论文摘要