Contrastive Learning for Whole Slide Image Representation: A Self-Supervised Approach in Digital Pathology
European Journal of Applied Science, Engineering and Technology(2024)
摘要
Image analysis in digital pathology is identified as a challenging field, particularly for AI-driven classification and search tasks. The high-resolution and large-scale nature of whole slide images (WSIs) present significant computational challenges in representing and analyzing these images effectively. The research endeavors to tackle these hurdles by presenting an innovative methodology grounded in self-supervised learning (SSL). Unlike prior SSL approaches that depend on augmenting at the patch level, the novel framework capitalizes on existing primary site information to directly glean effective representations from Whole Slide Images (WSIs). Moreover, the investigation integrates fully supervised contrastive learning to bolster the resilience of these representations for both classification and search endeavors. For experimentation, the study drew upon a dataset encompassing over 6,000 WSIs sourced from The Cancer Genome Atlas (TCGA) repository facilitated by the National Cancer Institute. The proposed architecture underwent training and assessment using this dataset. Evaluation primarily focused on scrutinizing performance across diverse primary sites and cancer subtypes, with particular attention dedicated to lung cancer classification. Impressively, the proposed architecture yielded outstanding outcomes, showcasing robust performance across the majority of primary sites and cancer subtypes. Furthermore, the study garnered the top position in validation for a lung cancer classification task.
更多查看译文
AI 理解论文
溯源树
样例
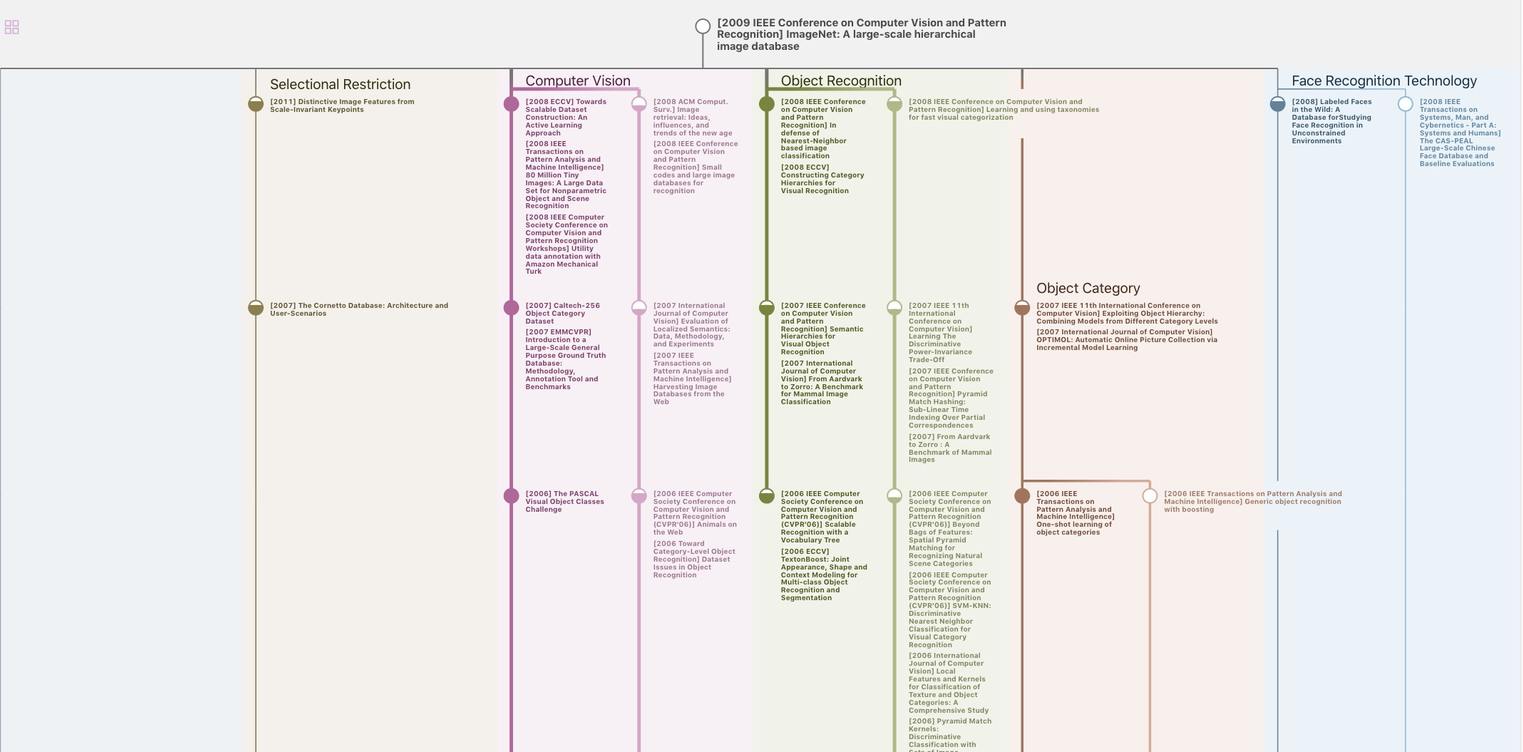
生成溯源树,研究论文发展脉络
Chat Paper
正在生成论文摘要