X2V: 3D Organ Volume Reconstruction from a Planar X-ray Image with Neural Implicit Methods
IEEE Access(2024)
摘要
In this work, an innovative approach is proposed for three-dimensional (3D) organ volume reconstruction from a single planar X-ray, namely X2V network. Such capability holds pivotal clinical potential, especially in real-time image-guided radiotherapy, computer-aided surgery, and patient follow-up sessions. Traditional methods for 3D volume reconstruction from X-rays often require the utilization of statistical 3D organ templates, which are employed in 2D/3D registration. However, these methods may not accurately account for the variation in organ shapes across different subjects. Our X2V model overcomes this problem by leveraging neural implicit representation. A vision transformer model is integrated as an encoder network, specifically designed to direct and enhance attention to particular regions within the X-ray image. The reconstructed meshes exhibit a similar topology to the ground truth organ volume, demonstrating the ability of X2V in accurately capturing the 3D structure from a 2D image. The effectiveness of X2V is evaluated on lung X-rays using several metrics, including volumetric Intersection over Union (IoU). X2V outperforms the state-of-the-art method in the literature for lungs (DeepOrganNet) by about 7-9% achieving IoU’s between 0.892-0.942 versus DeepOrganNet’s IoU of 0.815-0.888.
更多查看译文
关键词
3D reconstruction,X-ray,3D organ topology,neural implicit methods,vision transformers
AI 理解论文
溯源树
样例
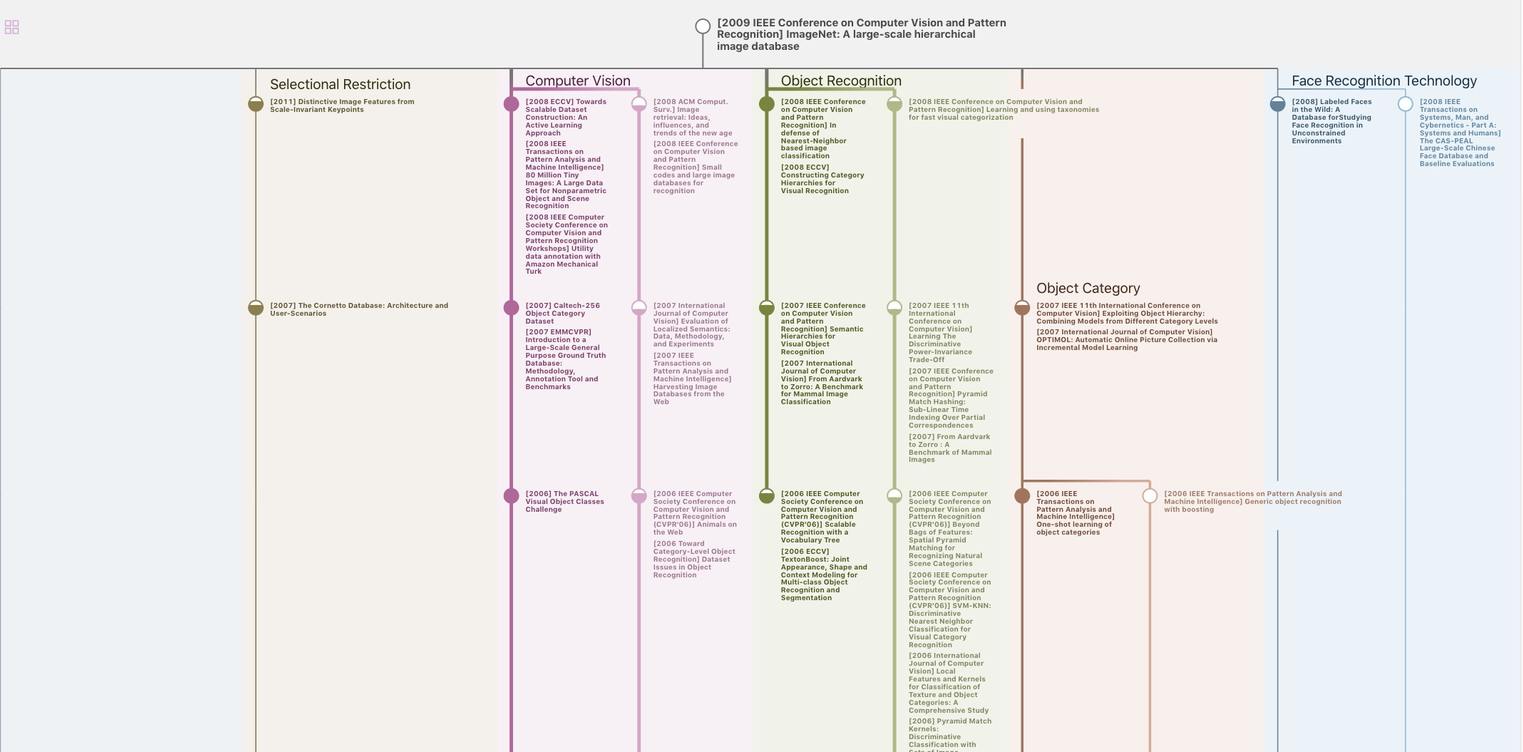
生成溯源树,研究论文发展脉络
Chat Paper
正在生成论文摘要