HVAC energy cost minimization in smart grids: A cloud-based demand side management approach with game theory optimization and deep learning
Energy and AI(2024)
摘要
In this paper, we present a novel cloud-based demand side management (DSM) optimization approach for the cost reduction of energy usage in heating, ventilation and air conditioning (HVAC) systems in residential homes at the district level. The proposed approach achieves optimization through scheduling of HVAC energy usage within permissible bounds set by house users. All houses are assumed to be equipped with smart home energy management (SHEM) or energy consumption scheduling (ECS) devices that are connected to the utility - or aggregator/energy service provider that may operate a virtual power plant (VPP) - via a dedicated communication network that is used to enable DSM. Additionally, each house SHEM has the ability to predict its own HVAC energy usage for the next 24 h using minimalistic deep learning (DL) prediction models. These predictions are communicated to the aggregator, which will then do day ahead optimizations using a game theory (GT) model. The GT model captures the interaction between aggregator and customers and identifies a solution to the GT problem that translates into HVAC energy peak shifting and peak reduction achieved by rescheduling HVAC energy usage. The algorithm that implements the GT based solution is executed once per day by the aggregator, which in turn solves for each house user a local optimization problem. In this algorithm, house users represent virtual game players - digital twins - whose individual objective is to reduce their energy cost without sacrificing much in comfort beyond a fixed threshold. The solution found by the algorithm is a set of customized HVAC schedules that the aggregator will offer or suggest to customers via DSM signals. If customers’ SHEM devices accept the offer energy cost reduction is achieved. We validate the proposed ideas via simulations with a custom simulation tool based on GridLab-D tool - integrated with DL prediction models and optimization libraries - with the capability of simulating an instrumented testcase for an arbitrary number of days. Simulation results show that HVAC energy cost can be reduced by up to 36% while indirectly also reducing the peak-to-average (PAR) as well of the aggregated net load by up to 9.97%.
更多查看译文
关键词
Day ahead demand side management (DSM),Appliance energy usage prediction,Residential energy usage scheduling flexibility,Market incentives,Non-cooperative game theory (GT),Dynamic price (DP),Energy cost minimization,Electricity cost minimization,Peak-to-average ratio (PAR) minimization,Machine learning (ML),Long short-term memory (LSTM),Smart Home Energy Management (SHEM),Load shifting,Internet of Things (ioT) applications,Smart grid,Heating,Ventilation and air conditioning (HVAC)
AI 理解论文
溯源树
样例
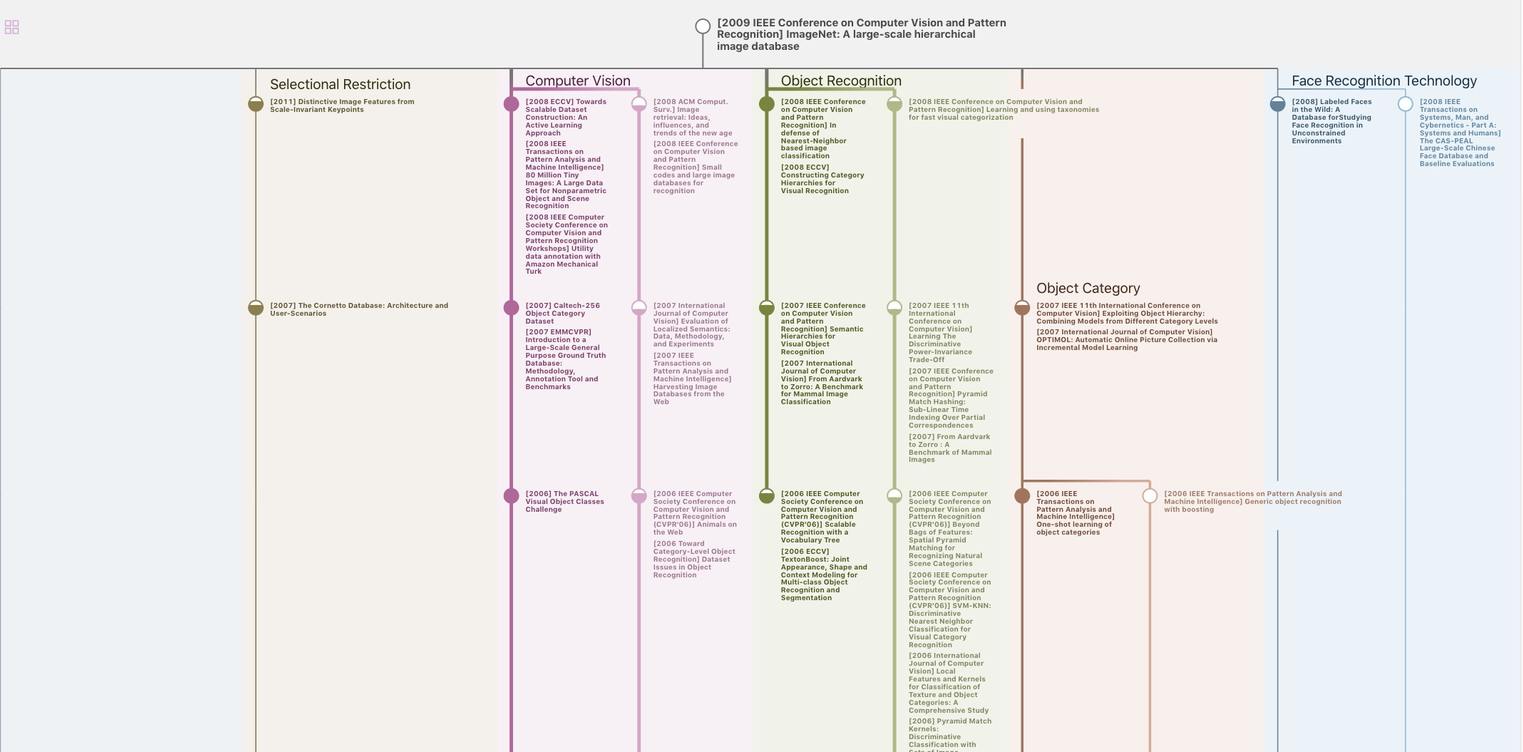
生成溯源树,研究论文发展脉络
Chat Paper
正在生成论文摘要