Enhancing smart road safety with federated learning for Near Crash Detection to advance the development of the Internet of Vehicles
Engineering Applications of Artificial Intelligence(2024)
摘要
We introduce an innovative methodology for the identification of vehicular collisions within Internet of Vehicles (IoV) applications. This approach combines a knowledge base system with deep learning for model selection in an ensemble learning setting. It is designed to provide a general near-crash detection capability without relying on domain-specific knowledge, enabling the development of generic deep learning models. Our proposed methodology employs a novel deep learning approach, wherein multiple learning models are individually trained for each image. Subsequently, visual features are computed and stored for each trained image, along with the associated loss values from the training phase. This stored information is utilized to select the most suitable models for processing new image data during the testing phase. To facilitate efficient model selection, we employ a kNN (k Nearest Neighbors) strategy. To enhance both data and model security in IoV environments, we implement an intelligent federated learning (FL) strategy. Users are organized into clusters, and we employ two distinct aggregation methods, departing from conventional federated learning approaches. In the initial stage, we aggregate model data from all users to create a global model representing collective knowledge. In the subsequent stage, we aggregate models from each cluster to generate customized local models. Users are provided with both global and local models, allowing them to select the most suitable model for their specific crash detection needs. We test our approach, that we call Knowledge Guided Deep Learning for Near Crash Detection (KGDL-NCD), on well-known NCD benchmarks. The results demonstrate that KGDL-NCD surpasses baseline solutions, achieving an AUC (Area Under Curve) metric of 0.95.
更多查看译文
关键词
Smart road safety,Federated learning,Guided deep learning,Internet of Vehicles
AI 理解论文
溯源树
样例
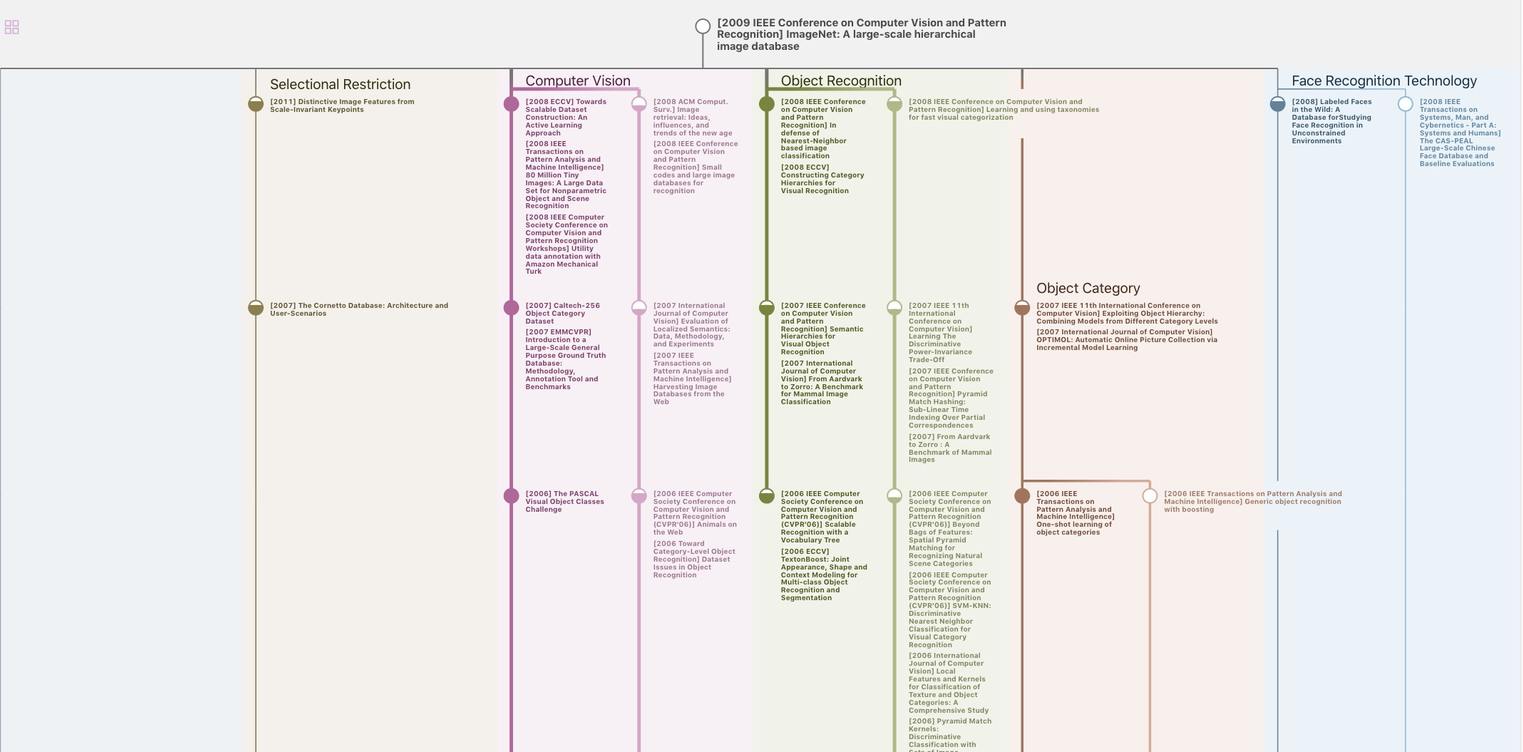
生成溯源树,研究论文发展脉络
Chat Paper
正在生成论文摘要