Inverse design of electromagnetic metamaterials: from iterative to deep learning-based methods
JOURNAL OF MICROMECHANICS AND MICROENGINEERING(2024)
摘要
In recent years, considerable research advancements have emerged in the application of inverse design methods to enhance the performance of electromagnetic (EM) metamaterials. Notably, the integration of deep learning (DL) technologies, with their robust capabilities in data analysis, categorization, and interpretation, has demonstrated revolutionary potential in optimization algorithms for improved efficiency. In this review, current inverse design methods for EM metamaterials are presented, including topology optimization (TO), evolutionary algorithms (EAs), and DL-based methods. Their application scopes, advantages and limitations, as well as the latest research developments are respectively discussed. The classical iterative inverse design methods categorized TO and EAs are discussed separately, for their fundamental role in solving inverse design problems. Also, attention is given on categories of DL-based inverse design methods, i.e. classifying into DL-assisted, direct DL, and physics-informed neural network methods. A variety of neural network architectures together accompanied by relevant application examples are highlighted, as well as the practical utility of these overviewed methods. Finally, this review provides perspectives on potential future research directions of EM metamaterials inverse design and integrated artificial intelligence methodologies.
更多查看译文
关键词
inverse design,electromagnetic metamaterials,topology optimization,evolutionary algorithms,surrogate modeling,inverse networks,physics-informed neural networks
AI 理解论文
溯源树
样例
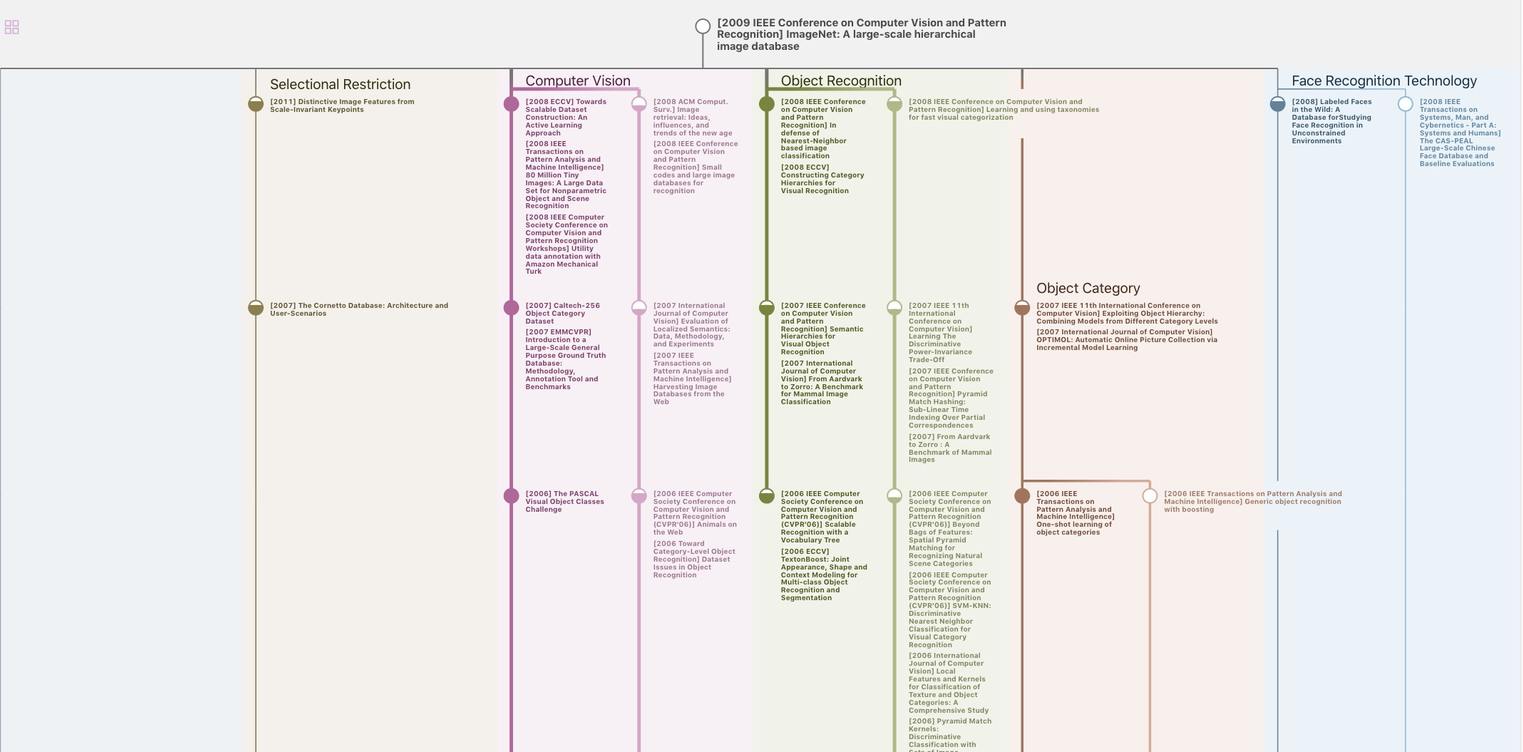
生成溯源树,研究论文发展脉络
Chat Paper
正在生成论文摘要