Major depressive disorder recognition by quantifying EEG signal complexity using proposed APLZC and AWPLZC
Journal of Affective Disorders(2024)
摘要
Background
Seeking objective quantitative indicators is important for accurately recognizing major depressive disorder (MDD). Lempel-Ziv complexity (LZC), employed to characterize neurological disorders, faces limitations in tracking dynamic changes in EEG signals due to defects in the coarse-graining process, hindering its precision for MDD objective quantitative indicators.
Methods
This work proposed Adaptive Permutation Lempel-Ziv Complexity (APLZC) and Adaptive Weighted Permutation Lempel-Ziv Complexity (AWPLZC) algorithms by refining the coarse-graining process and introducing weight factors to effectively improve the precision of LZC in characterizing EEGs and further distinguish MDD patients better. APLZC incorporated the ordinal pattern, while False Nearest Neighbor and Mutual Information algorithms were introduced to determine and adjust key parameters adaptively. Furthermore, we proposed AWPLZC by assigning different weights to each pattern based on APLZC. Thirty MDD patients and 30 healthy controls (HCs) were recruited and their 64-channel resting EEG signals were collected. The complexities of gamma oscillations were then separately computed using LZC, APLZC, and AWPLZC algorithms. Subsequently, a multi-channel adaptive K-nearest neighbor model was constructed for identifying MDD patients and HCs.
Results
LZC, APLZC, and AWPLZC algorithms achieved accuracy rates of 78.29 %, 90.32 %, and 95.13 %, respectively. Sensitivities reached 67.96 %, 85.04 %, and 98.86 %, while specificities were 88.62 %, 95.35 %, and 89.92 %, respectively. Notably, AWPLZC achieved the best performance in accuracy and sensitivity, with a specificity limitation.
Limitation
The sample size is relatively small.
Conclusion
APLZC and AWPLZC algorithms, particularly AWPLZC, demonstrate superior effectiveness in differentiating MDD patients from HCs compared with LZC. These findings hold significant clinical implications for MDD diagnosis.
更多查看译文
关键词
Electroencephalogram,Gamma oscillations,Lempel-Ziv complexity,Major depressive disorder
AI 理解论文
溯源树
样例
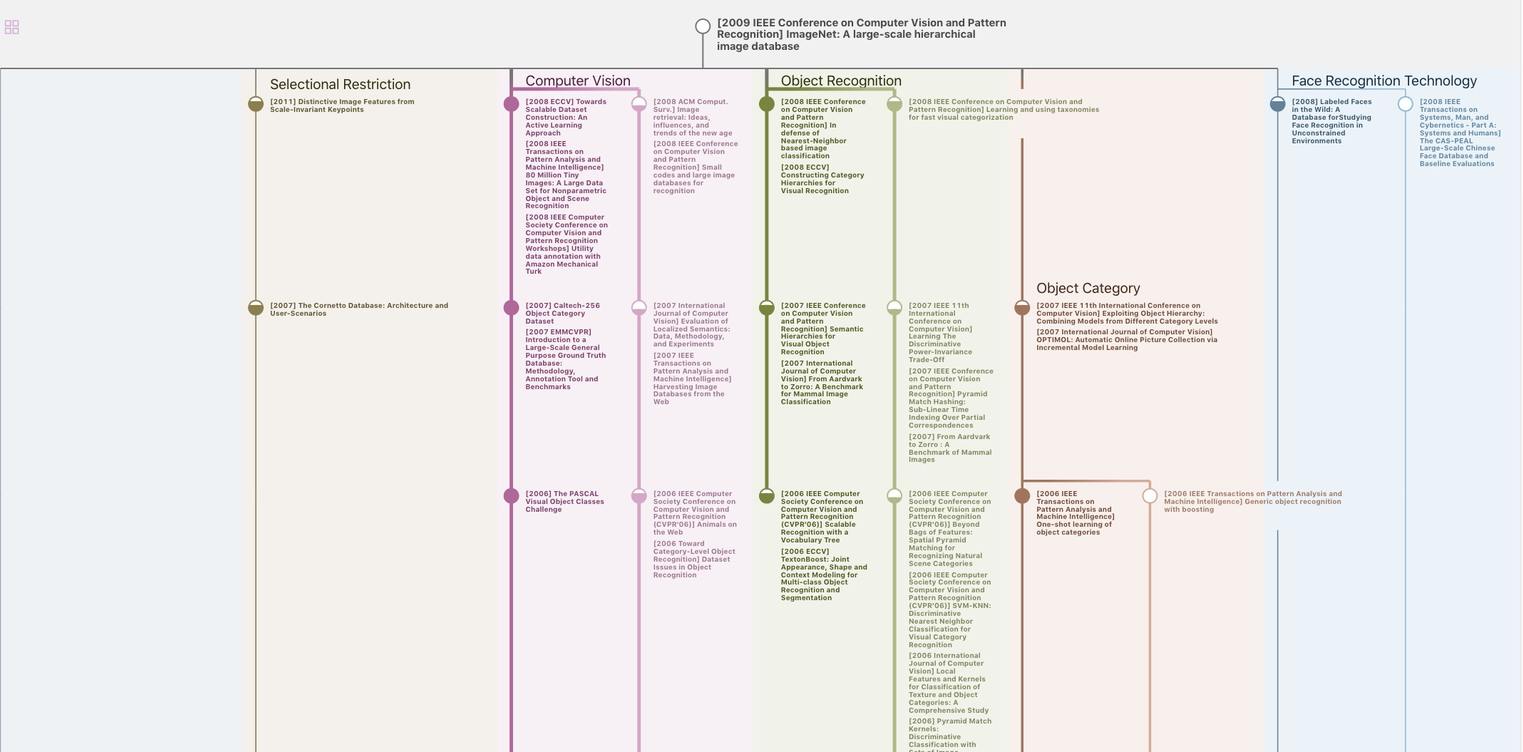
生成溯源树,研究论文发展脉络
Chat Paper
正在生成论文摘要