Fuzzy Deep Learning for Modeling Uncertainty in Character Recognition Using EEG signals
Applied Soft Computing(2024)
摘要
Classification of brain signals for character recognition is a challenging task associated with uncertainties. This study aims to present an Improved Type-2 Fuzzy Deep Learning (IT2FDL) framework for uncertainty management in character recognition using EEG signals by learning rules from each other in the learning layer. The Evolutionary Algorithm (EA) was employed for this study's optimum design of the proposed IT2FDL model. This algorithm was used to determine the T2F set parameters. The model parameters were tuned by Parallel Swarm Optimization (PSO) Algorithm. The present framework optimized the fusion layer of the model. This layer is a multi-layered Recurrent Type2 Fuzzy Neural Network (RT2FNN) with a learning layer composed of RNN cells. For performance evaluation, the models were examined using three public databases: BCI Competition III-Dataset II, BCI Competition II-Dataset IIb, and EEG Dataset. The optimized IT2FDL model outperformed the counterpart models with a significant improvement of test score of 2.5% for Competition III-Dataset II at epoch 15, 2.5% for Competition II-Dataset IIb at epoch 3, and 1% for EEG Dataset at epoch 15. The proposed IT2FDL-PSO (GRU) model showed a mean test score of 96.2% at epoch 15 for all 58 subjects, which outperformed other models with many subjects for character recognition.
更多查看译文
关键词
Brain-Compute Interfaces (BCI),Electroencephalogram (EEG),Spelling,Classification,Type2 Fuzzy System,Recurrent Neural Network (RNN),Optimization Algorithm,PSO
AI 理解论文
溯源树
样例
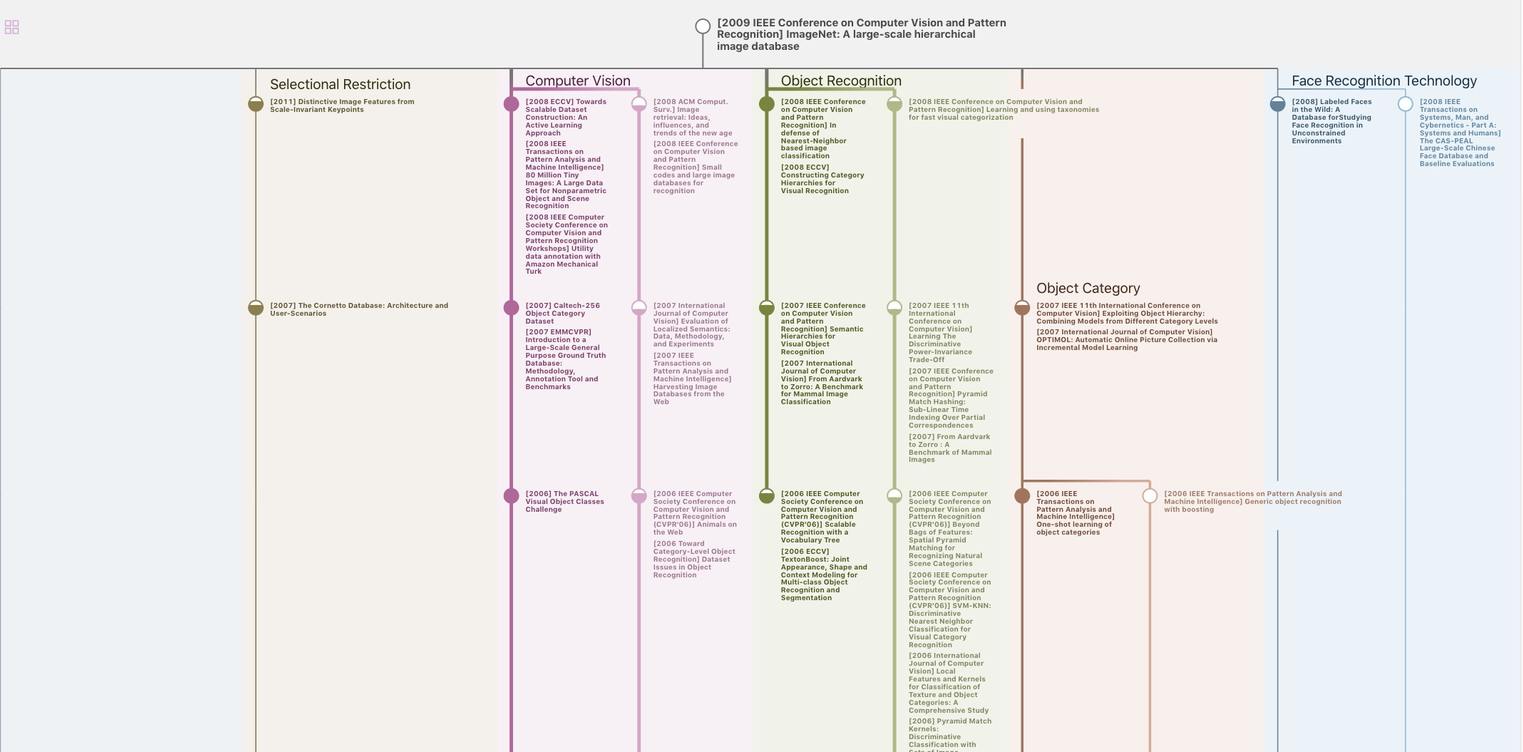
生成溯源树,研究论文发展脉络
Chat Paper
正在生成论文摘要