Learn What You Want to Unlearn: Unlearning Inversion Attacks against Machine Unlearning
CoRR(2024)
摘要
Machine unlearning has become a promising solution for fulfilling the "right
to be forgotten", under which individuals can request the deletion of their
data from machine learning models. However, existing studies of machine
unlearning mainly focus on the efficacy and efficiency of unlearning methods,
while neglecting the investigation of the privacy vulnerability during the
unlearning process. With two versions of a model available to an adversary,
that is, the original model and the unlearned model, machine unlearning opens
up a new attack surface. In this paper, we conduct the first investigation to
understand the extent to which machine unlearning can leak the confidential
content of the unlearned data. Specifically, under the Machine Learning as a
Service setting, we propose unlearning inversion attacks that can reveal the
feature and label information of an unlearned sample by only accessing the
original and unlearned model. The effectiveness of the proposed unlearning
inversion attacks is evaluated through extensive experiments on benchmark
datasets across various model architectures and on both exact and approximate
representative unlearning approaches. The experimental results indicate that
the proposed attack can reveal the sensitive information of the unlearned data.
As such, we identify three possible defenses that help to mitigate the proposed
attacks, while at the cost of reducing the utility of the unlearned model. The
study in this paper uncovers an underexplored gap between machine unlearning
and the privacy of unlearned data, highlighting the need for the careful design
of mechanisms for implementing unlearning without leaking the information of
the unlearned data.
更多查看译文
AI 理解论文
溯源树
样例
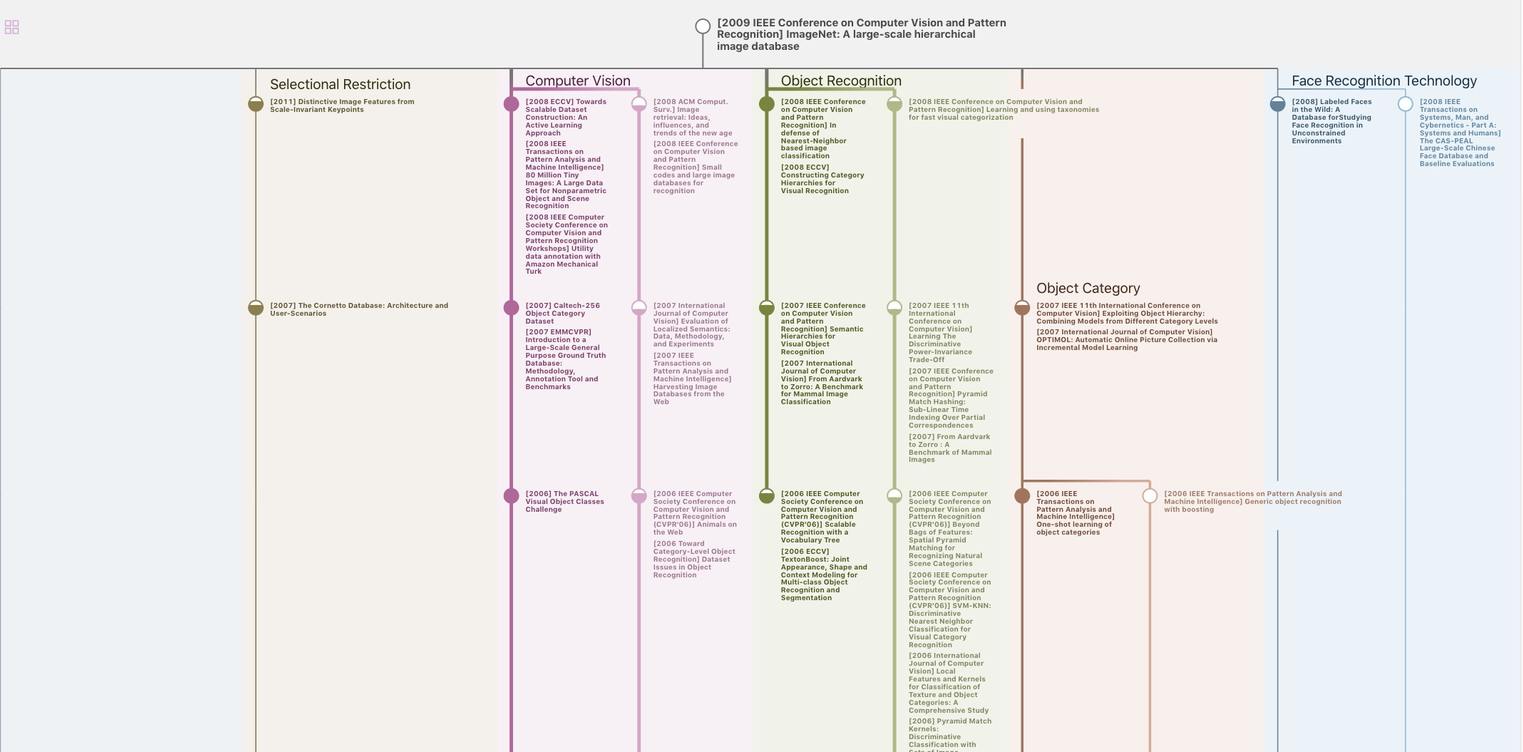
生成溯源树,研究论文发展脉络
Chat Paper
正在生成论文摘要