Neighborhood Attention Transformer Multiple Instance Learning for Whole Slide Image Classification
crossref(2024)
摘要
Abstract Purpose:: : The purpose of the passage is to introduce and describe NATMIL (Neighborhood Attention Transformer Multiple Instance Learning), an innovative deep learning approach aimed at improving the classification of tumor cells and subtypes in whole slide images (WSIs) of tissue section biopsies for diagnosing cancer. Method:: The method involves leveraging dependencies among WSI tiles using the Neighborhood Attention Transformer, which incorporates contextual constraints as prior knowledge into multiple instance learning models. The approach utilizes weakly supervised methods, classifying WSIs using image parts or tiles along with attention ratings, to address the limitations of traditional methods. Result:: NATMIL has demonstrated superior performance compared to other weakly supervised algorithms when subtyping non-small cell lung cancer (NSLC) and lymph node (LN) tumors. The accuracy values achieved on the Camelyon dataset reached 89.6\% and 88.1\% for TCGA-LUSC. The introduction of NATMIL presents an effective and scalable sliding window attention technique for vision, known as Neighborhood Attention, which improves performance and reduces the burden on pathologists while making more data available. Conclusion:: NATMIL's success in improving tumor-level classifications by considering the interdependence of nearby tiles in histopathological pictures highlights its potential to enhance cancer diagnosis. By incorporating contextual constraints and leveraging dependencies among WSI tiles, NATMIL demonstrates promising results in improving accuracy and reducing false positives and negatives, thus advancing the field of cancer diagnosis through deep learning-based approaches.
更多查看译文
AI 理解论文
溯源树
样例
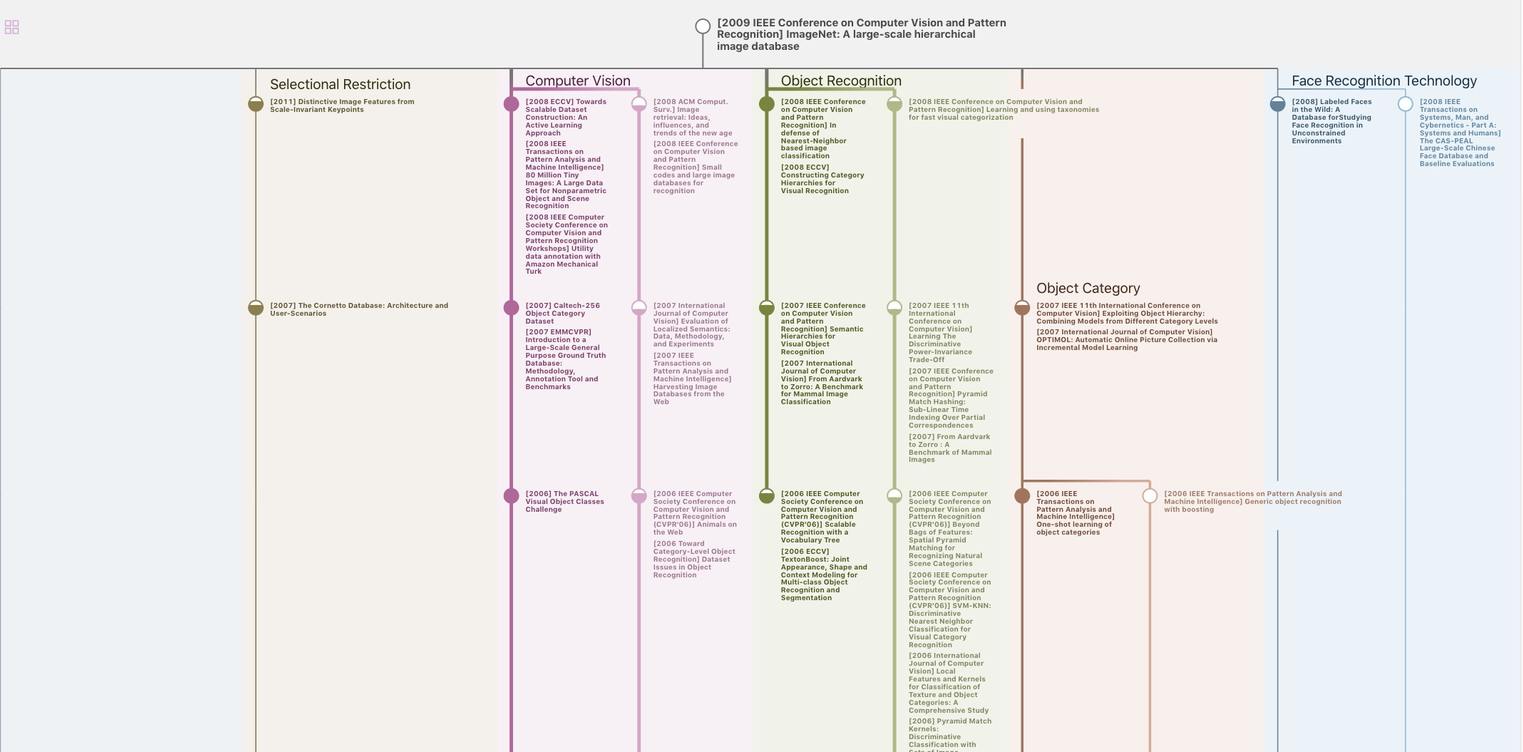
生成溯源树,研究论文发展脉络
Chat Paper
正在生成论文摘要