Time Series EEG Image classification using pretrained Residue Network for Detection of Schizophrenia
2024 International Conference on Automation and Computation (AUTOCOM)(2024)
摘要
Schizophrenia (SCZ) is a persistent psychotic mental condition that has a negative impact on a person's social life. Electroencephalogram (EEG) can be used as a tool to study brain activity patterns associated with SCZ. This study analyzed 19-channel Time Series EEG Image (TSEI) for SCZ detection. The study is conducted on a balanced dataset of 720 samples with 360 samples from each group i.e. SCZ and healthy control (HC). Two popular Residue network (ResdNet) architectures: ResdNet-18 and ResdNet-50 are used in this study. In order to train and test the model 5-fold data division protocol is used. Different performance metric such as selevtivity, F-1 score, positive predictive value (PPV), accuracy etc. are obtained in order to analyse the performance of proposed methodology. With ResdNet-50 highest classification accuracy achieved was 93.6%, whereas with ResdNet-18 it was limited up to 91.2%. Also, in terms of other performance measures ResdNet-50 either outperforms or has equal performance as ResdNet-18. This study concluded that TSEI in combination with ResdNet-50 can be used to provide assistance to clinician for diagnosis of SCZ.
更多查看译文
AI 理解论文
溯源树
样例
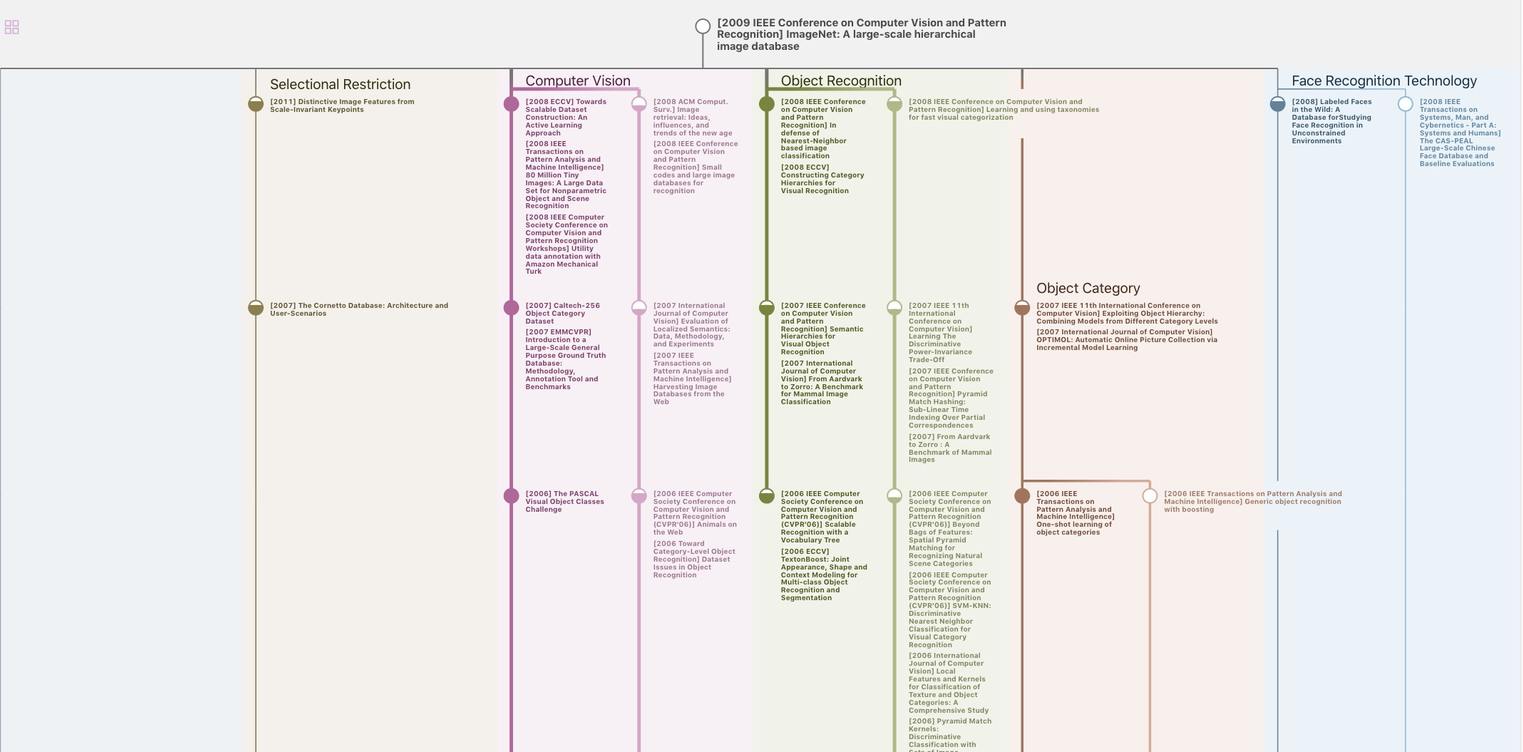
生成溯源树,研究论文发展脉络
Chat Paper
正在生成论文摘要