Blind image quality assessment based on hierarchical dependency learning and quality aggregation
Neurocomputing(2024)
摘要
Image quality assessment (IQA) aims to build a quality prediction model to assess image quality automatically rather than artificially. Due to a lack of reference images, blind image quality assessment (BIQA) has become an attractive yet challenging research topic. Inspired by the hierarchical perception mechanism in the human visual system, some existing BIQA methods aggregate multi-stage features of a convolutional neural network (CNN). However, they are regardless of the latent dependencies. To solve this problem, we propose a novel BIQA method based on hierarchical dependency learning and quality aggregation (HDLaQA). The proposed method includes multi-stage feature extraction, hierarchical dependency learning, and quality aggregation. In multi-stage feature extraction, a CNN is used as the feature extractor and multi-stage features are output for further learning. In hierarchical dependency learning, spatial and channel dependencies among the multi-stage features are modeled. To this end, a dual-head spatial dependency (DSD) module is designed to harvest the spatial dependencies between the adjacent-stage features and deliver these dependencies to the next stage. Moreover, exponential bilinear pooling (EBP) is presented to learn the channel dependencies, which is more stable than commonly used BP. In quality aggregation, multiple quality scores are predicted based on the learned dependencies, and multiple learnable weights are used to measure the importance of the predicted scores for final quality evaluation. Experimental results on seven IQA databases demonstrate the competitiveness of the proposed method on both synthetic and authentic distortions.
更多查看译文
关键词
Blind image quality assessment,Human visual system,Spatial dependencies,Channel dependencies
AI 理解论文
溯源树
样例
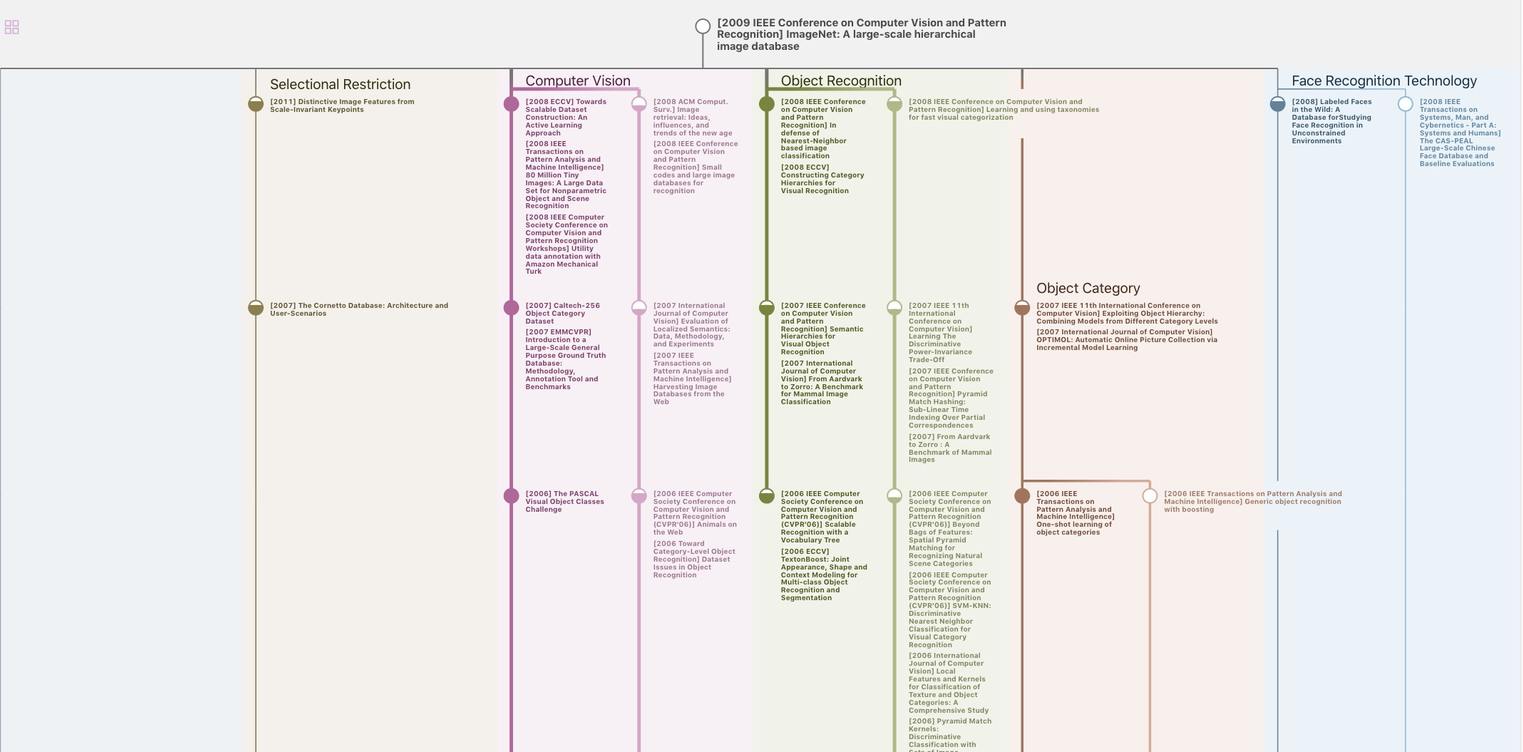
生成溯源树,研究论文发展脉络
Chat Paper
正在生成论文摘要