Mutual Correlation Network for few-shot learning
Neural Networks(2024)
摘要
Most metric-based Few-Shot Learning (FSL) methods focus on learning good embeddings of images. However, these methods either lack the ability to explore the cross-correlation (i.e., correlated information) between image pairs or explore limited consensus among the correlation map constrained by the limited receptive field of CNN. We propose a Mutual Correlation Network (MCNet) to explore global consensus among the correlation map by using the self-attention mechanism which has a global receptive field. Our MCNet contains two core modules: (1) a multi-level embedding module that generates multi-level embeddings for an image pair which capture hierarchical semantics, and (2) a mutual correlation module that refines correlation map of two embeddings and generates more robust relational embeddings. Extensive experiments show that our MCNet achieves competitive results on four widely-used few-shot classification benchmarks miniImageNet, tieredImageNet, CUB-200-2011, and CIFAR-FS. Code is available at https://github.com/DRGreat/MCNet.
更多查看译文
关键词
Few-shot classification,Mutual correlation,Multi-level embedding,Self-attention mechanism
AI 理解论文
溯源树
样例
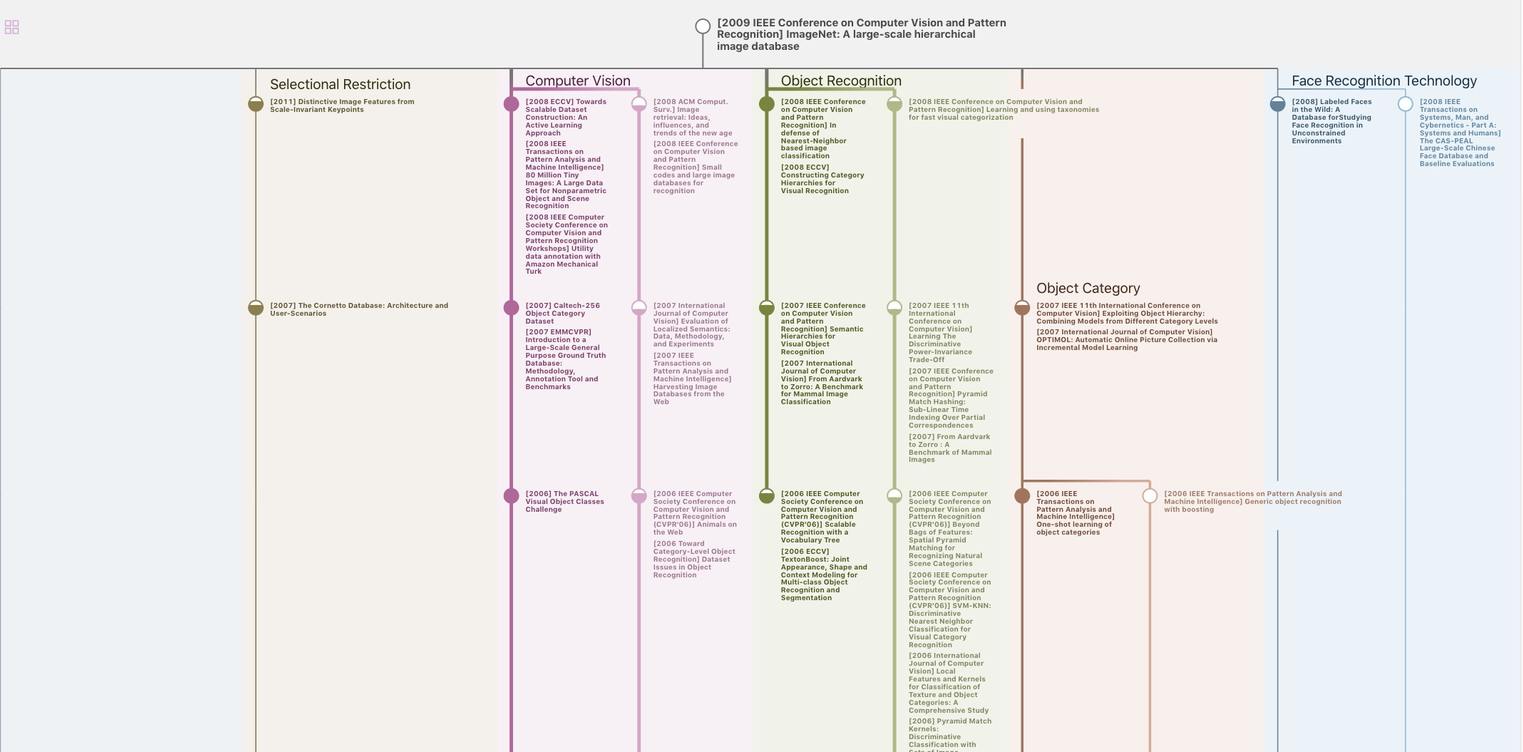
生成溯源树,研究论文发展脉络
Chat Paper
正在生成论文摘要