Computationally Efficient Unsupervised Deep Learning for Robust Joint AP Clustering and Beamforming Design in Cell-Free Systems
arxiv(2024)
摘要
In this paper, we consider robust joint access point (AP) clustering and
beamforming design with imperfect channel state information (CSI) in cell-free
systems. Specifically, we jointly optimize AP clustering and beamforming with
imperfect CSI to simultaneously maximize the worst-case sum rate and minimize
the number of AP clustering under power constraint and the sparsity constraint
of AP clustering. By transformations, the semi-infinite constraints caused by
the imperfect CSI are converted into more tractable forms for facilitating a
computationally efficient unsupervised deep learning algorithm. In addition, to
further reduce the computational complexity, a computationally effective
unsupervised deep learning algorithm is proposed to implement robust joint AP
clustering and beamforming design with imperfect CSI in cell-free systems.
Numerical results demonstrate that the proposed unsupervised deep learning
algorithm achieves a higher worst-case sum rate under a smaller number of AP
clustering with computational efficiency.
更多查看译文
AI 理解论文
溯源树
样例
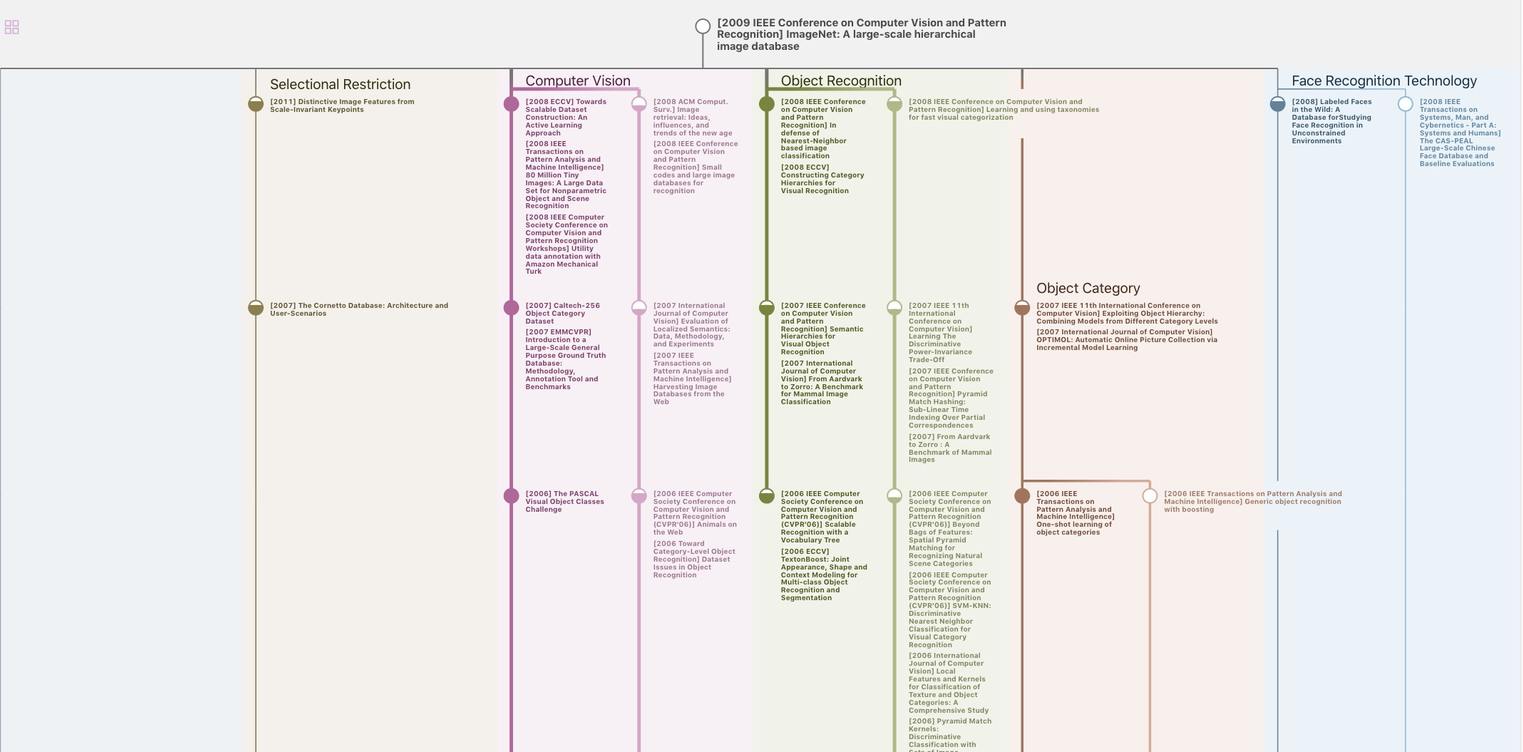
生成溯源树,研究论文发展脉络
Chat Paper
正在生成论文摘要