A Single-sample Pruning and Clustering Method for Neural Network Verification.
Asia-Pacific Software Engineering Conference(2023)
摘要
The verification techniques based on formal methods can provide deterministic guarantees for the robustness of Deep Neural Networks(DNNS). However, the enormous scale of DNNS makes the application of such methods in this field a huge challenge. To address this problem, this study proposes a single-sample sub-network pruning method, which can identify redundant nodes by combining neuron coverage and the symbolic interval propagation method to reduce the network verification scale. In addition, to solve the problem of too many sub-networks to be pruned, according to the similarity of neuron coverage between samples, we propose a corresponding clustering algorithm to establish sub-networks for different categories of samples to improve the verification efficiency. We combine the MIPverify verification tool to validate the above method. Experiments show that the sub-networks can give the same robust validation results and similar robustness bounds as the original network, while greatly reducing the validation time and network size.
更多查看译文
AI 理解论文
溯源树
样例
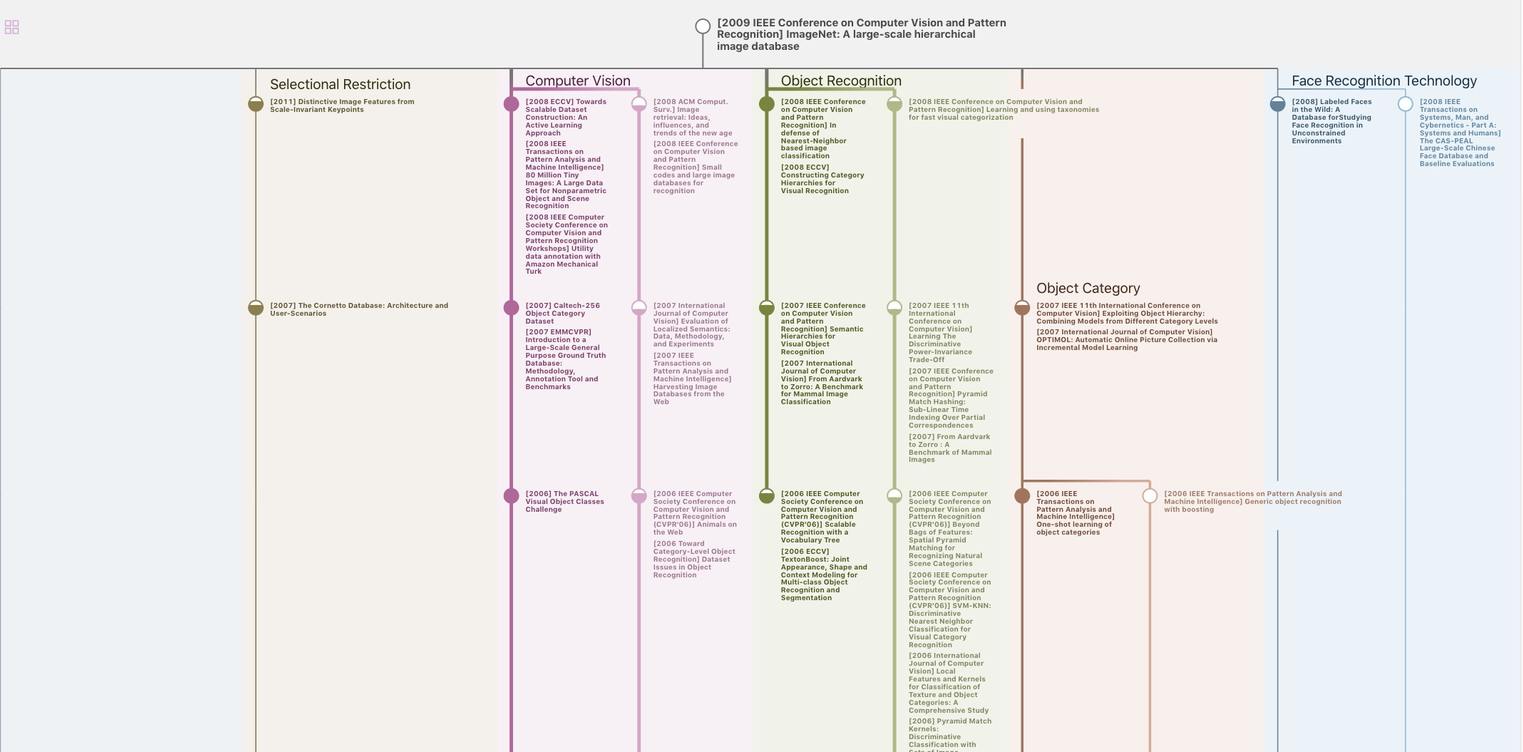
生成溯源树,研究论文发展脉络
Chat Paper
正在生成论文摘要