Effect of variable selection strategy on the predictive models for adverse pregnancy outcomes of pre-eclampsia: A retrospective study
Placenta and Reproductive Medicine(2024)
摘要
Objectives: The improvement of prediction for adverse pregnancy outcomes is quite essential to the women suffering from pre-eclampsia, while the collection of predictive indicators is the prerequisite. The traditional knowledge-based strategy for variable selection confronts challenge referring to dataset with high-dimensional or unfamiliar data. In this study, we employed five different automatic variable selection methods to screen out influential indicators, and evaluated the performance of constructed predictive models. Methods: Seven hundreds and thirty-three Han-Chinese women were enrolled and 56 clinical and laboratory variables were recorded. After grouping based on binary pregnancy outcomes, statistical description and analysis were performed. Then, utilizing forward stepwise logistic regression (FSLR) as the reference method, another four variable selection strategies were included for filtering contributing variables as the predictive subsets, respectively. Finally, the logistic regression prediction models were constructed by the five subsets and evaluated by the receiver operator characteristic curve. Results: The variables confirmed statistical significance between the adverse and satisfactory outcomes groups did not overlap with the variables selected by selection strategies. “Platelet” and “Creatinine clearance rate” were the most influential indicator to predict adverse maternal outcome, while “Birth weight of neonates” was the best indicator for predicting adverse neonatal outcome. In average, the predictive models for neonatal outcomes achieved better performance than models for maternal outcomes. “Mutual information” and “Recursive feature elimination” were the best strategy under current dataset and study design. Conclusions: Variable selection strategies may provide an alternative approach besides picking influential indicators by statistical significance. Future work will focus on applying different variable selection methods to the high-dimensional dataset, which includes novel or unfamiliar variables. This aims to identify the most appropriate collection of predictors that can enhance prediction ability and clinical decision-making.
更多查看译文
AI 理解论文
溯源树
样例
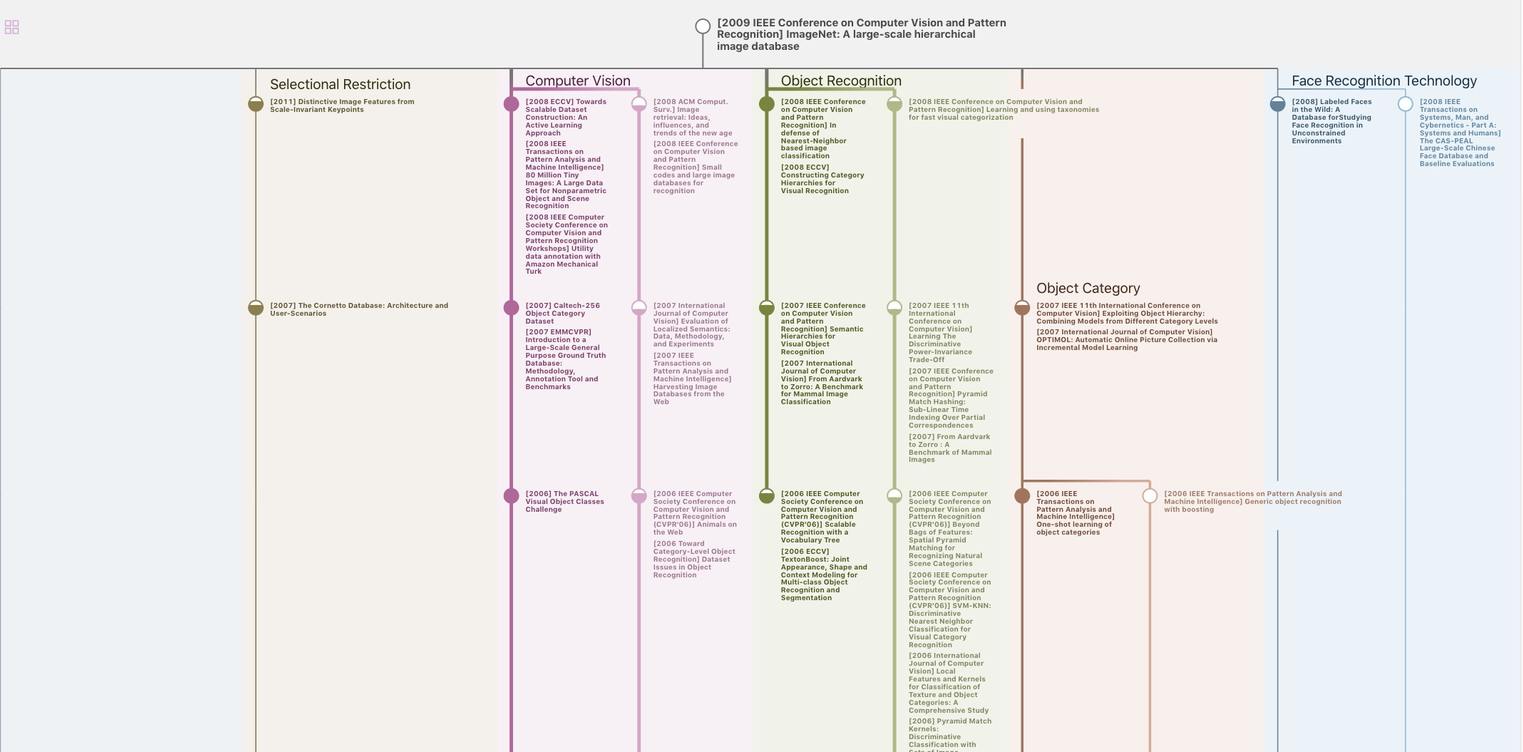
生成溯源树,研究论文发展脉络
Chat Paper
正在生成论文摘要