Cross-domain NER under a Divide-and-Transfer Paradigm
ACM Transactions on Information Systems(2024)
摘要
Cross-domain Named Entity Recognition (NER) transfers knowledge learned from a rich-resource source domain to improve the learning in a low-resource target domain. Most existing works are designed based on the sequence labeling framework, defining entity detection and type prediction as a monolithic process. However, they typically ignore the discrepant transferability of these two sub-tasks: the former locating spans corresponding to entities is largely domain-robust, while the latter owns distinct entity types across domains. Combining them into an entangled learning problem may contribute to the complexity of domain transfer. In this work, we propose the novel divide-and-transfer paradigm in which different sub-tasks are learned using separate functional modules for respective cross-domain transfer. To demonstrate the effectiveness of divide-and-transfer, we concretely implement two NER frameworks by applying this paradigm with different cross-domain transfer strategies. Experimental results on 10 different domain pairs show the notable superiority of our proposed frameworks. Experimental analyses indicate that significant advantages of the divide-and-transfer paradigm over prior monolithic ones originate from its better performance on low-resource data and a much greater transferability. It gives us a new insight into cross-domain NER. Our code is available at our github.
更多查看译文
AI 理解论文
溯源树
样例
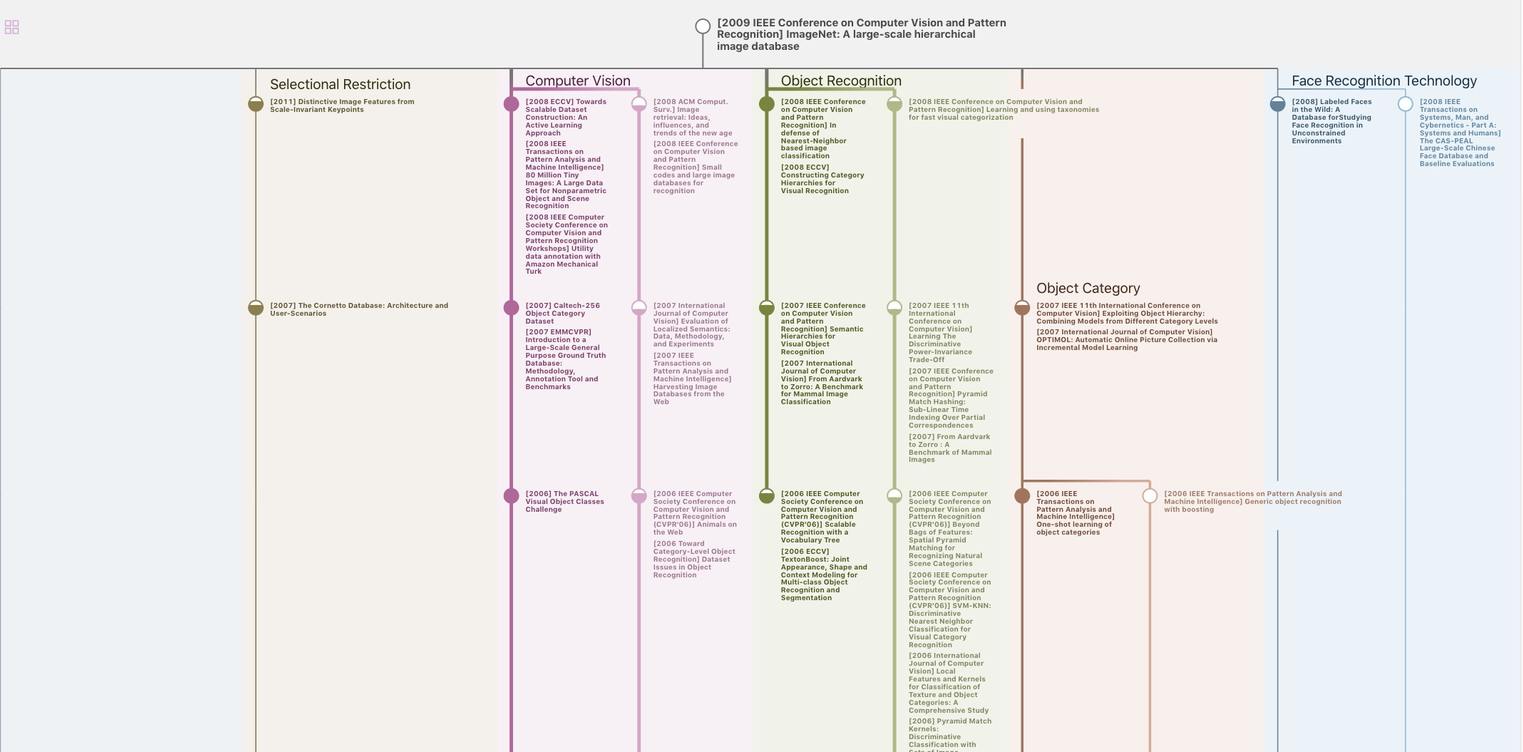
生成溯源树,研究论文发展脉络
Chat Paper
正在生成论文摘要