Federated Texture Classification: Implementing Colorectal Histology Image Analysis using Federated Learning
JOURNAL OF ELECTRICAL SYSTEMS(2023)
摘要
This research explores neural network models' performance and adaptability in the context of the colorectal histology dataset as it pertains to the categorization of textures. Inception, VGG19, and MobileNet, together with their federated variations, are among the models being examined. The study includes a detailed evaluation, parameter analysis, and training information. VGG19 stands out as a particularly noteworthy high performance, with remarkable accuracy, precision, and recall. Due to its lightweight design, MobileNet performs less well, but its potential is enhanced by the addition of federated learning. The accuracy and precision of federated versions of Inception, VGG19, MobileNet, and a Lightweight MobileNet model are competitive, with FL-Lightweight MobileNet achieving outstanding results. The work has important ramifications for the field of medical image analysis since it shows how federated learning may balance the need for data confidentiality and privacy with model performance. This study marks a turning point in the development of medical imaging by opening the door to in-depth investigation into the complex interactions across federated paradigms. Furthermore, these results provide a compelling story in the wider discussion of how cutting-edge technologies and the pressing needs of contemporary healthcare might work together.
更多查看译文
关键词
Neural networks,Texture classification,Colorectal histology,Inception,VGG19,MobileNet,Federated learning,Privacy-preserving,Medical image analysis,Accuracy,Precision,Recall,Model performance,Data security,Distributed learning,Healthcare
AI 理解论文
溯源树
样例
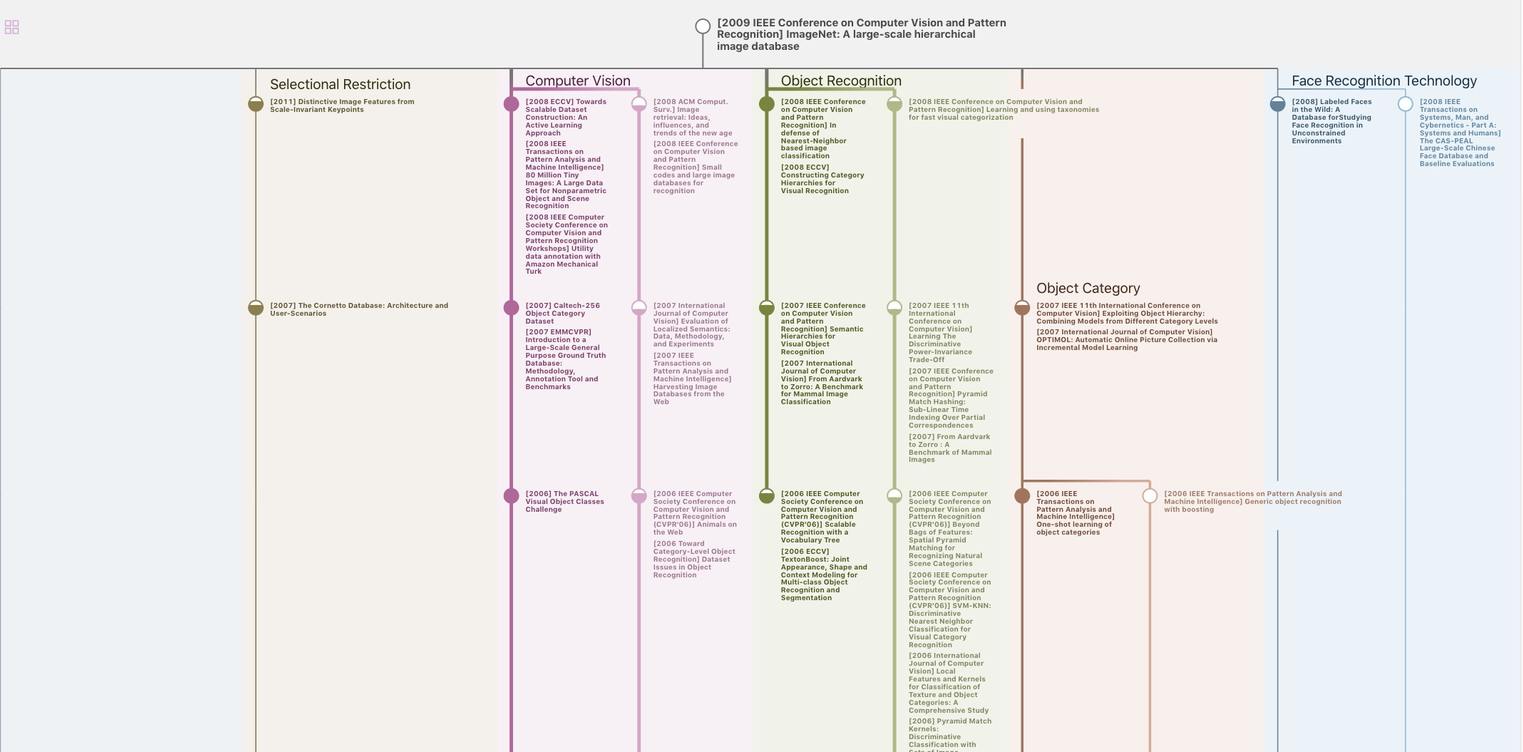
生成溯源树,研究论文发展脉络
Chat Paper
正在生成论文摘要