Exploring the Effectiveness of Different State Spaces and Reward Functions in Reinforcement Learning-based Control of a DC/DC Buck Converter
2023 25TH EUROPEAN CONFERENCE ON POWER ELECTRONICS AND APPLICATIONS, EPE'23 ECCE EUROPE(2023)
摘要
The precise control of DC/DC buck converters is critical for achieving stability, efficiency, and reliability of power systems. Reinforcement learning (RL), a type of machine learning, has shown promising results in solving control problems in various domains. The proposed study aims to explore the potential of using RL techniques, specifically the deep deterministic policy gradient (DDPG) algorithm, to control DC/DC buck converters and to identify the optimal combination of state space and reward functions to achieve the best control performance for DC/DC buck converters. This is done by analyzing the performance of the control system and comparing different sets of state space and reward functions. The findings of this study have the potential to contribute to the development of improved control systems for power electronics. This research could ultimately lead to more efficient, stable, and reliable power systems.
更多查看译文
关键词
"Buck Converter","Machine Learning","DC/DC power converters","Deep Neural Network","Converter control"
AI 理解论文
溯源树
样例
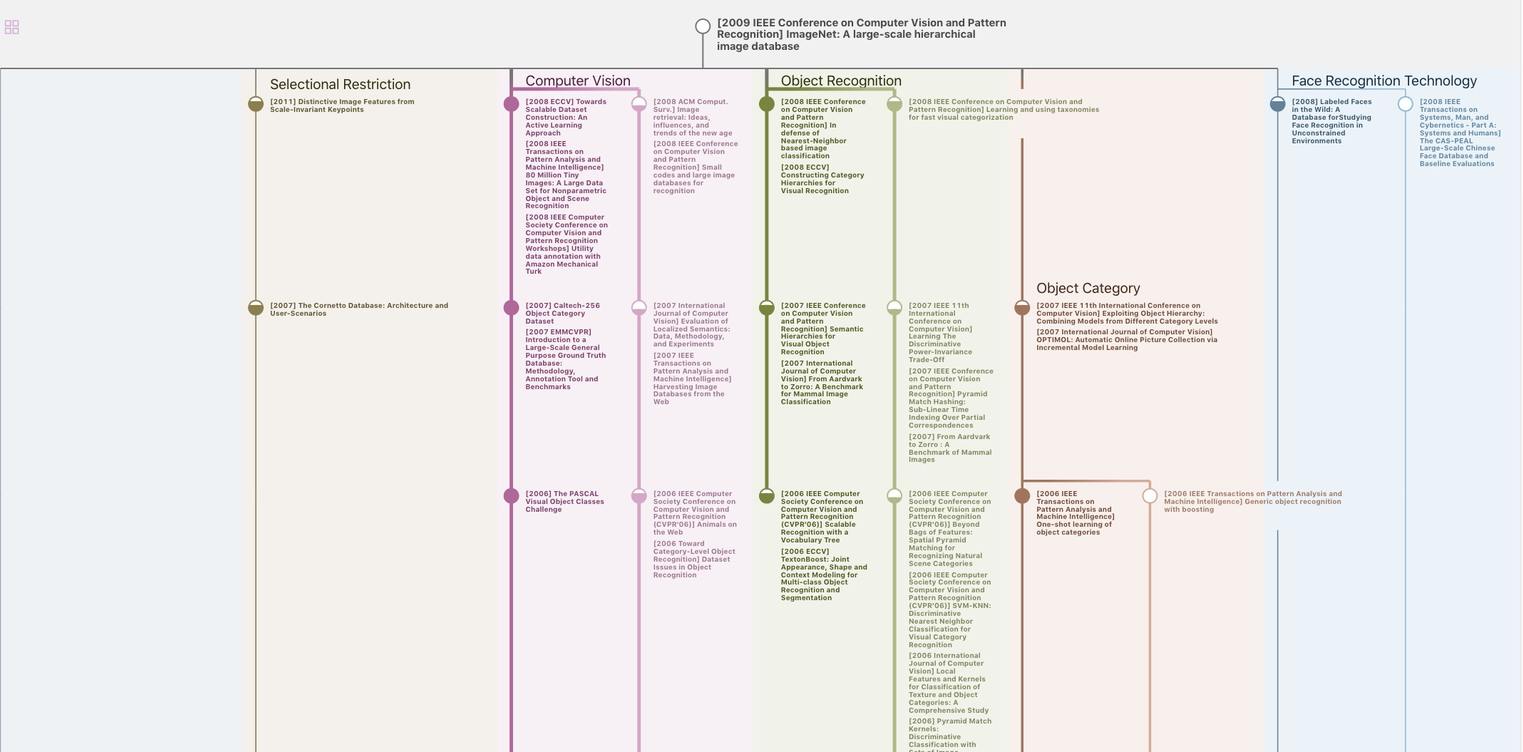
生成溯源树,研究论文发展脉络
Chat Paper
正在生成论文摘要