FM-IGNN: Interaction Graph Neural Network Fine-grained Matching for Session -based Recommendation
23RD IEEE INTERNATIONAL CONFERENCE ON DATA MINING, ICDM 2023(2023)
摘要
Session-based recommendation plays a critical role in a number of scenarios, e.g., c-commerce, which predicts user behavior based on sessions. The primary challenge is to match user interests and candidate items accurately. Existing studies mainly learn the representation of session items and candidate items independently, and aggregate session representations through an attention mechanism. Nevertheless, user interests are usually cross -domain, and the characteristics of the items are also multifactorial. Fixed session and candidate item representations lead to suboptimal matching. To some extent, it limits the representation capability of the model. In this paper, we present an Interaction Graph Neural Network with Fine-grained Matching, named FM-IGNN, for session-based recommendation. It incorporates interactive features into item and session modeling to match candidate items and user interests more accurately. We first propose an Interaction Graph Neural Network (IGNN) to learn candidate -aware session item representation and session aware candidate item representation interactively. We then design a Fine-grained interest Matching (FM) framework to learn user interests related to candidate items, and calculate the matching scores of users and candidate items at multi-levels. Experiments on three benchmark datasets demonstrate that FM-IGNN outperforms the state-of-the-art (SOTA) schemes with a large margin. Specifically, on the Tmall dataset, it achieves relative improvement of up to 20.54%, 16.07%, 13.41%, and 15.52% on the Precision@ 10, MRRC010, Precision(4)20, and MRR@20, respectively.
更多查看译文
关键词
Session-based recommendation,Graph neural network,Fine-grained Matching
AI 理解论文
溯源树
样例
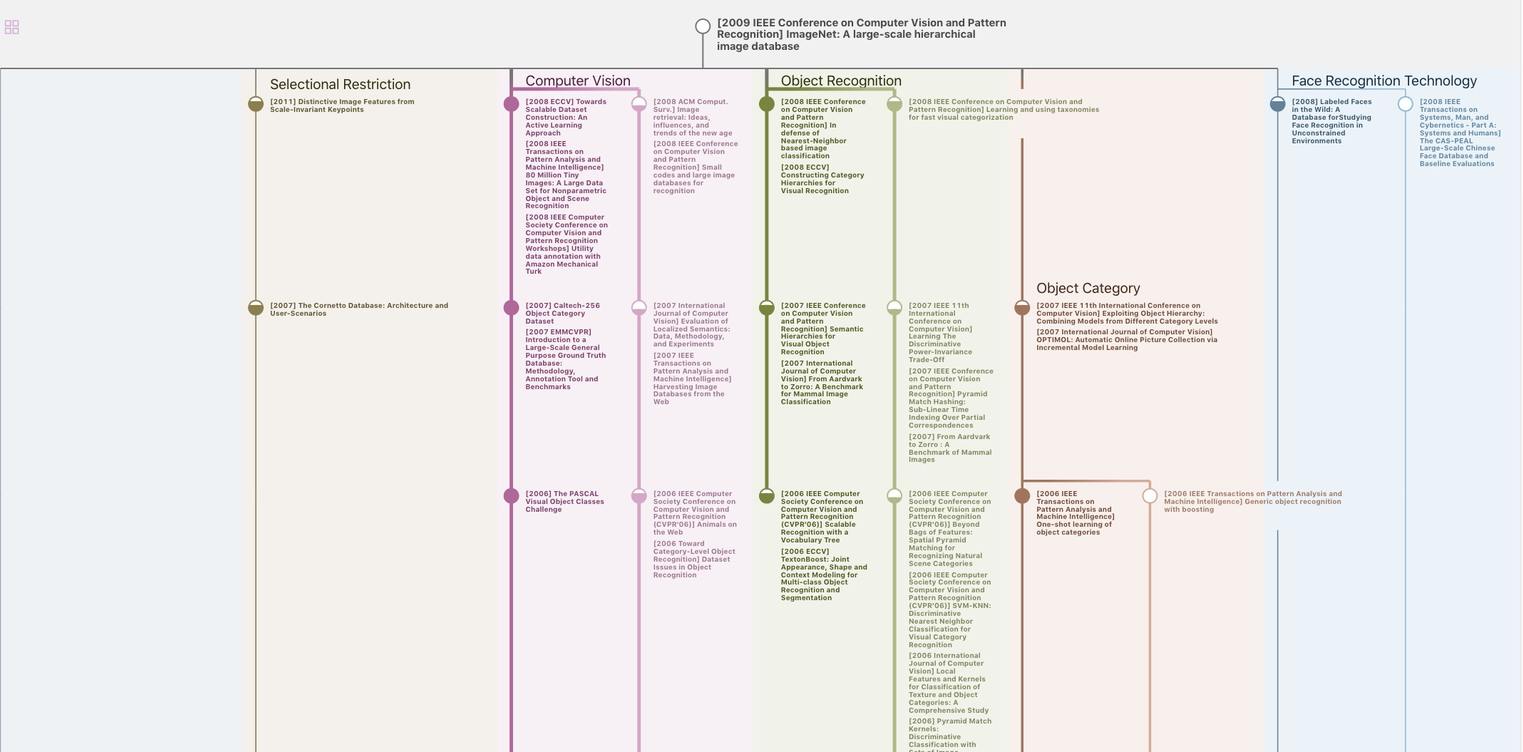
生成溯源树,研究论文发展脉络
Chat Paper
正在生成论文摘要