A tradeoff between false discovery and true positive proportions for sparse high-dimensional logistic regression
ELECTRONIC JOURNAL OF STATISTICS(2024)
摘要
The logistic regression model is a simple and classic approach to binary classification, where in sparse high-dimensional settings, one believes that only a small proportion of the predictive variables are relevant to the response variable with nonnull regression coefficients. We focus on regularized logistic regression models and the analysis is valid for a large group of regularizers, including folded-concave regularizers such as MCP and SCAD. For finite samples, the discrepancy between the estimated and true nonnull coefficients is evaluated by the false discovery and true positive rates. We show that the false discovery rate can be described using a nonlinear tradeoff function of power asymptotically using a system of equations with six parameters. The analysis is conducted in an "average-over-components" fashion for the unknown parameter and follows the conventional assumptions of the literature in the relevant field. More specifically, we assume a linear growth rate n/p -> delta > 0 covering not only the typical high dimensional settings where p >= n but also for n > p. Further, we propose two applications of this tradeoff function that improve the reproducibility of variable selection: (1) a sample size calculation procedure to achieve a certain power under a prespecified level of false discovery rate using the tradeoff; (2) calibration of the false discovery rate for variable selection taking power into consideration. A similar asymptotic analysis for the model-X knockoff, which provides a selection with a controlled false discovery rate, is investigated to show how to compare two selection methods by comparing the tradeoff curves. We illustrate the tradeoff analysis and its corresponding applications using simulated and real data.
更多查看译文
关键词
High-dimensional data,FDR control,knockoff,logistic regression,sparsity,false discovery rate
AI 理解论文
溯源树
样例
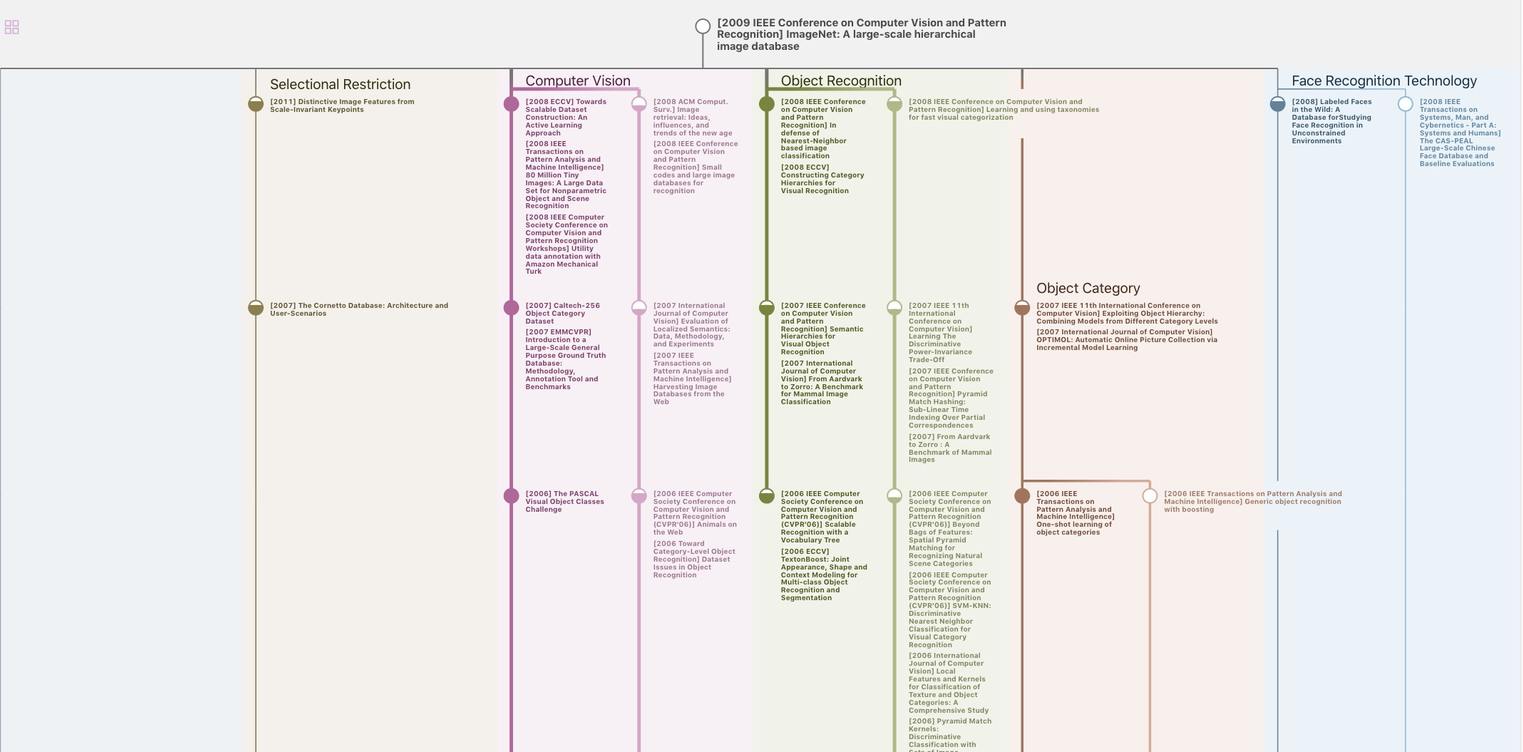
生成溯源树,研究论文发展脉络
Chat Paper
正在生成论文摘要