Disturbance observer-based control for cable-driven parallel robots with external disturbance using deep reinforcement learning
ASIAN JOURNAL OF CONTROL(2024)
摘要
In cable-driven parallel robots (CDPRs) practical applications, external disturbances inevitably influence the actuator performance, leading to low positioning accuracy of end-effector (EE), and even system crashes. This paper addresses a disturbance observer-based control scheme using deep reinforcement learning (RL) to suppress the effect of external disturbances. A controller via the non-singular terminal sliding mode (NTSM) is proposed to enhance the system robustness. The estimation of the disturbance is investigated, and the deep RL is employed to enhance the model-based disturbance observer, namely, the RLDO, and a corresponding controller via NTSM (RLDO-NTSMC) is proposed. Simulations and experimental results validate that the RLDO effectively improves the estimation accuracy, and the RLDO-NTSMC significantly increases the control accuracy of CDPRs. The root mean square errors of the EE's positioning errors controlled by the RLDO-NTSMC decreased by over 93% compared with the classical augmented proportional-derivative (APD) controller.
更多查看译文
关键词
cable-driven parallel robots,disturbance estimation,reinforcement learning
AI 理解论文
溯源树
样例
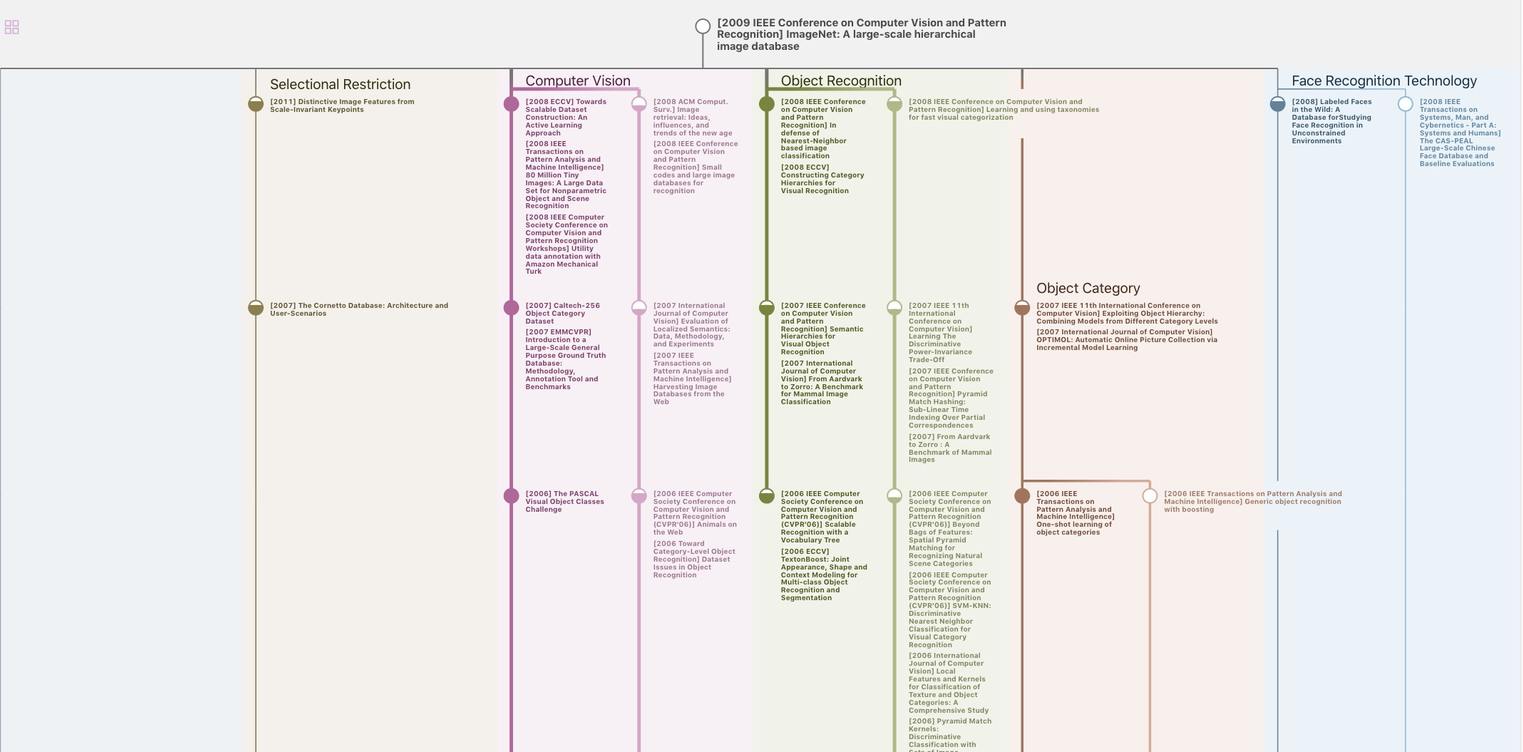
生成溯源树,研究论文发展脉络
Chat Paper
正在生成论文摘要