Development of a deep-learning model for phase-separation structure of diblock copolymer based on self-consistent field analysis
ADVANCED COMPOSITE MATERIALS(2024)
摘要
Self-consistent field (SCF) analysis is an indispensable tool for predicting the microphase separation structures of polymer alloys. However, the computation of the phase-separated structures in the equilibrium state is computationally intensive, leading to high costs. To address this challenge, we propose a novel deep learning approach that leverages a generative adversarial network (GAN), a powerful deep generative model, to accelerate SCF analysis. Specifically, we trained the GAN using comprehensive data obtained from SCF analysis, enabling us to generate various images of feasible structures that are similar to the SCF analysis results. Our results demonstrate that the latent variables in the GAN are linked to the physical parameters and features of the phase-separation structures.
更多查看译文
关键词
Polymer alloy,phase-separation structure,self-consistent field theory,,deep learning
AI 理解论文
溯源树
样例
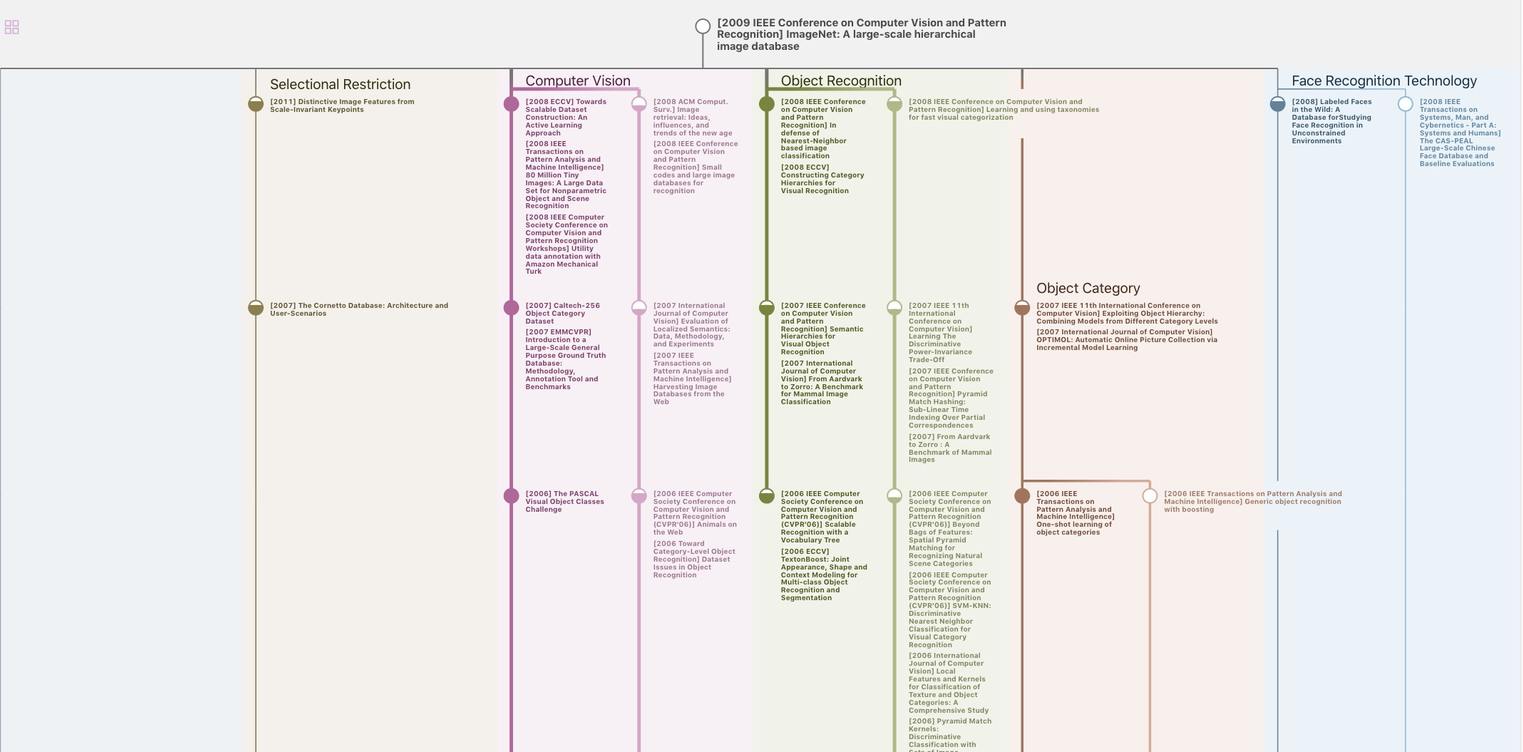
生成溯源树,研究论文发展脉络
Chat Paper
正在生成论文摘要