Standigm ASK?: knowledge graph and artificial intelligence platform applied to target discovery in idiopathic pulmonary fibrosis
BRIEFINGS IN BIOINFORMATICS(2024)
摘要
Standigm ASK (TM) revolutionizes healthcare by addressing the critical challenge of identifying pivotal target genes in disease mechanisms-a fundamental aspect of drug development success. Standigm ASK (TM) integrates a unique combination of a heterogeneous knowledge graph (KG) database and an attention-based neural network model, providing interpretable subgraph evidence. Empowering users through an interactive interface, Standigm ASK (TM) facilitates the exploration of predicted results. Applying Standigm ASK (TM) to idiopathic pulmonary fibrosis (IPF), a complex lung disease, we focused on genes (AMFR, MDFIC and NR5A2) identified through KG evidence. In vitro experiments demonstrated their relevance, as TGF beta treatment induced gene expression changes associated with epithelial-mesenchymal transition characteristics. Gene knockdown reversed these changes, identifying AMFR, MDFIC and NR5A2 as potential therapeutic targets for IPF. In summary, Standigm ASK (TM) emerges as an innovative KG and artificial intelligence platform driving insights in drug target discovery, exemplified by the identification and validation of therapeutic targets for IPF.
更多查看译文
关键词
target identification,knowledge graph,neural network,idiopathic pulmonary fibrosis,epithelial-mesenchymal transition
AI 理解论文
溯源树
样例
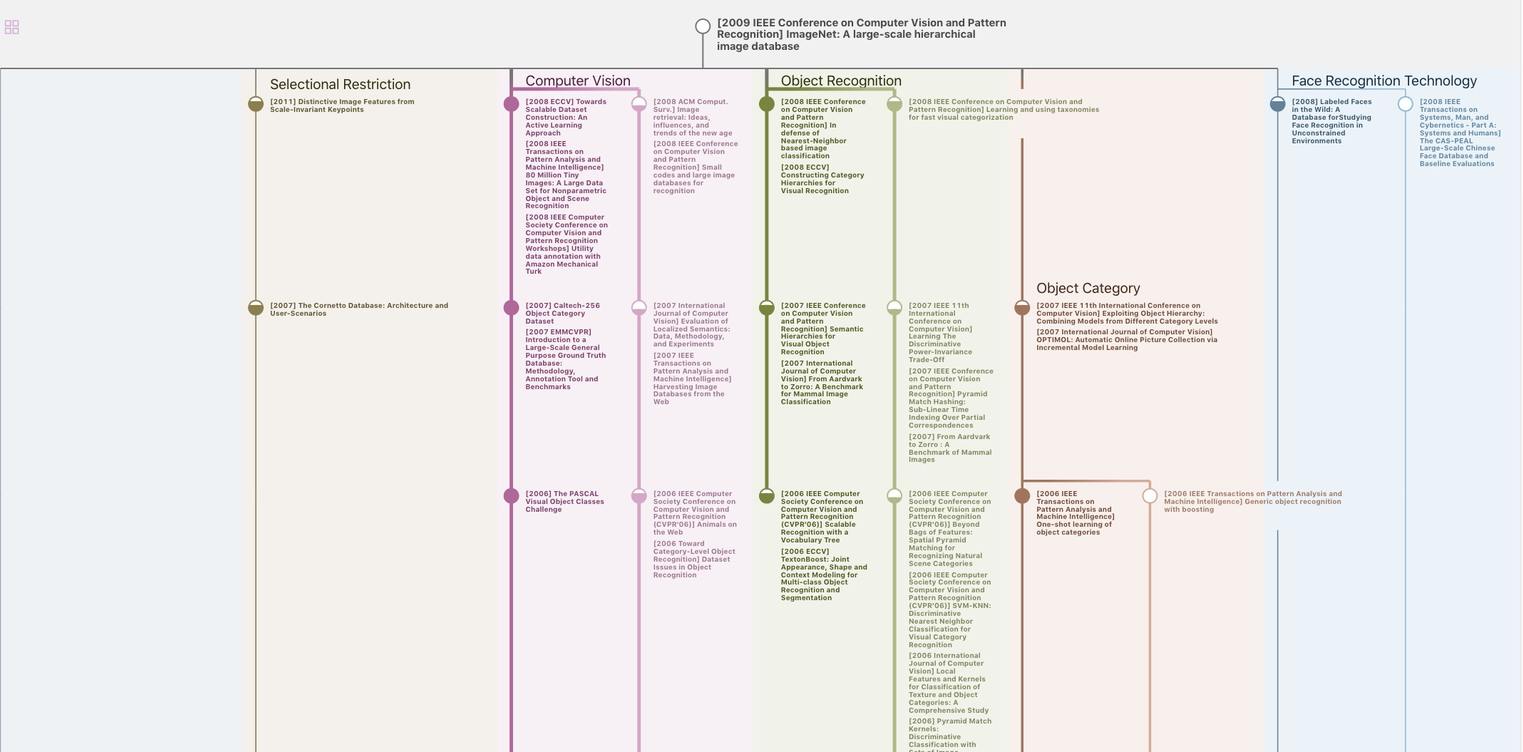
生成溯源树,研究论文发展脉络
Chat Paper
正在生成论文摘要