Edge-guided adaptive multi-feature learning for breast ultrasound image segmentation
BIOMEDICAL SIGNAL PROCESSING AND CONTROL(2024)
摘要
An Edge-guided Adaptive Multi-feature Learning Network (EAML-Net) for lesion segmentation is proposed in this paper to address the issues of blurred edges, high speckle noise, low contrast and serious ultrasound artifacts in breast ultrasound images. EAML-Net consists of an encoder, a decoder and an Edge Guidance Module (EGM). Firstly, in the encoder, an Adaptive Residual Multi-feature Learning (ARML) module and a Mixed Pooling (MP) layer are utilized to mitigate the problems of speckle noise and low contrast. The former is used to adaptively obtain multi-resolution features, while the latter can guarantee the efficient transfer of those features between different layers. In addition, they can improve the segmentation accuracy of small targets. Then, the decoder gradually restores features to the original image size by upsampling. Furthermore, the EGM is explored to alleviate the problem of blurred edges and refine the segmentation results. In EGM, an Information Fusion Block (IFB) is used to extract and fuse those features located in the encoder and then detect them by an edge detection method to guide the network to learn more abundant edge information. Finally, the learning ability of EAML-Net is further accelerated with the supervision of a hybrid loss function so as to obtain superior segmentation performance. Experiment results on two public breast ultrasound datasets demonstrate that EAML-Net achieves 86.82% and 84.58% of the Dice index, respectively. Moreover, comparison and ablation experiment results further demonstrate the effectiveness of the proposed algorithm.
更多查看译文
关键词
Breast ultrasound image segmentation,Adaptive residual learning,Multi-feature learning,Edge guidance
AI 理解论文
溯源树
样例
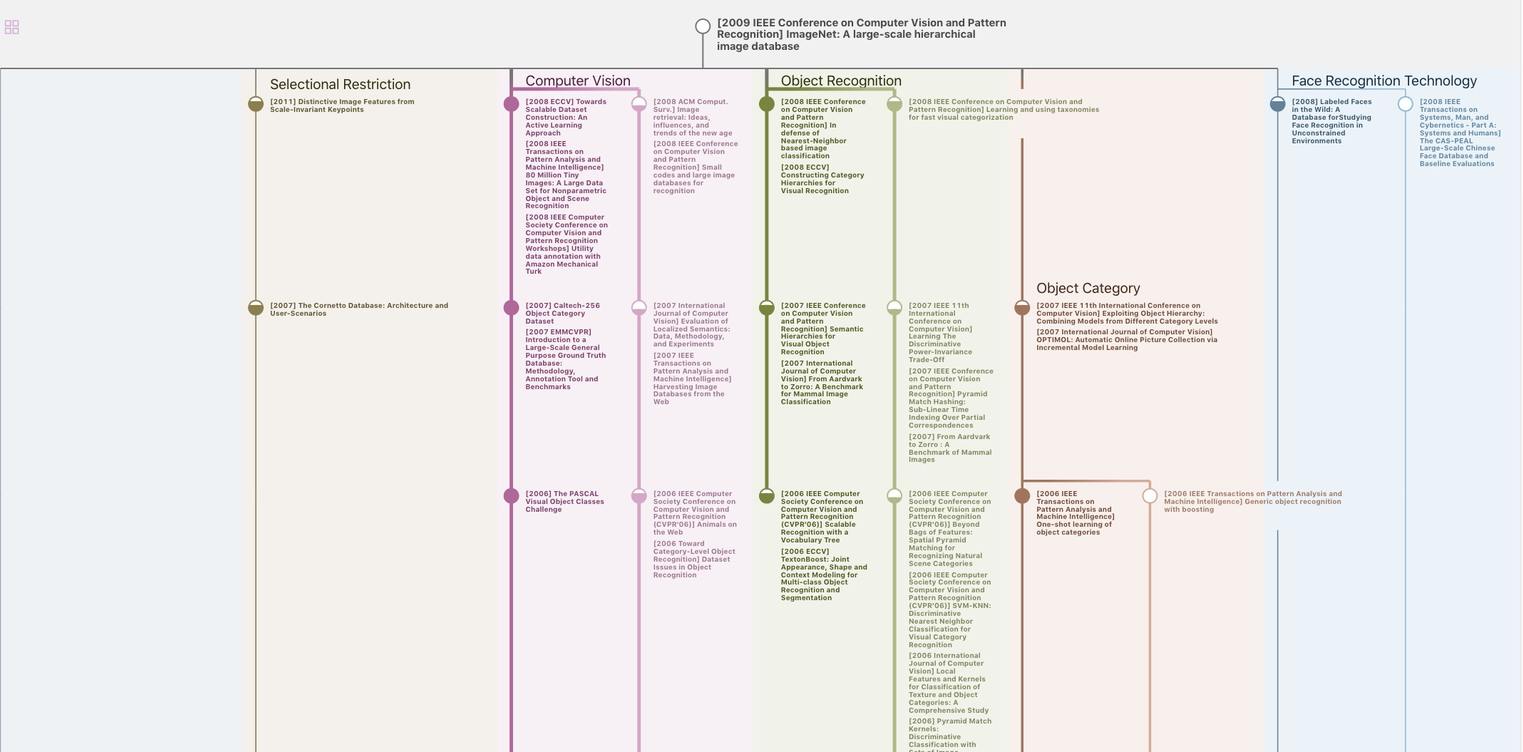
生成溯源树,研究论文发展脉络
Chat Paper
正在生成论文摘要