Exploration, Exploitation, and Engagement in Multi-Armed Bandits with Abandonment
JOURNAL OF MACHINE LEARNING RESEARCH(2024)
摘要
The traditional multi-armed bandit (MAB) model for recommendation systems assumes the user stays in the system for the entire learning horizon. In new online education platforms such as ALEKS or new video recommendation systems such as TikTok, the amount of time a user spends on the app depends on how engaging the recommended contents are. Users may temporarily leave the system if the recommended items cannot engage the users. To understand the exploration, exploitation, and engagement in these systems, we propose a new model, called MAB-A where "A" stands for abandonment and the abandonment probability depends on the current recommended item and the user's past experience (called state). We propose two algorithms, ULCB and KL-ULCB, both of which do more exploration (being optimistic) when the user likes the previous recommended item and less exploration (being pessimistic) when the user does not. We prove that both ULCB and KL-ULCB achieve logarithmic regret, Oplog Kq, where K is the number of visits (or episodes). Furthermore, the regret bound under KL-ULCB is asymptotically sharp. We also extend the proposed algorithms to the general-state setting. Simulation results show that the proposed algorithms have significantly lower regret than the traditional UCB and KL-UCB, and Q-learning-based algorithms.
更多查看译文
关键词
multi-armed bandit,abandonment,exploration,exploitation,regret bound
AI 理解论文
溯源树
样例
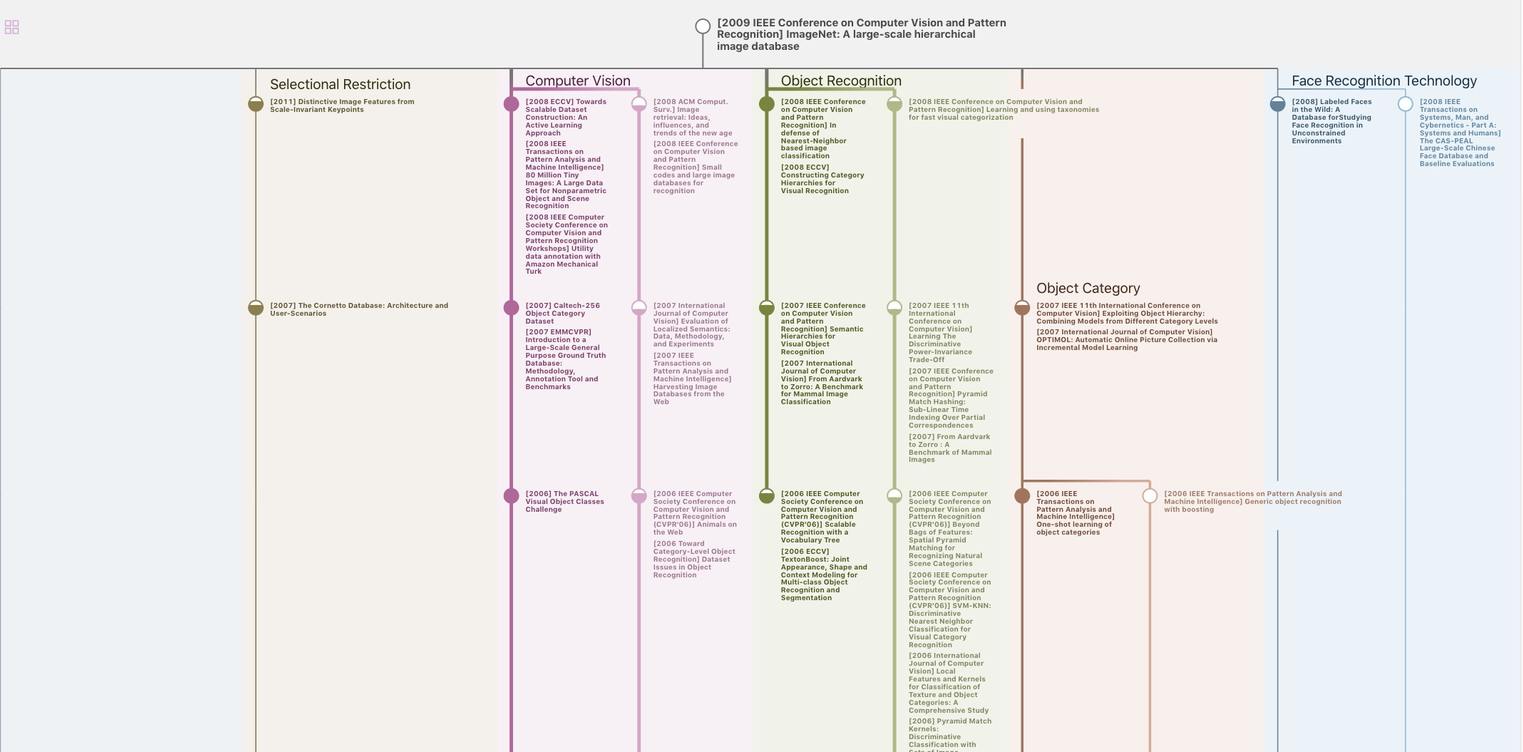
生成溯源树,研究论文发展脉络
Chat Paper
正在生成论文摘要