FCA-based reasoning for privacy
LOGIC JOURNAL OF THE IGPL(2024)
摘要
Notwithstanding the potential danger to security and privacy, sharing and publishing data has become usual in Data Science. To preserve privacy, de-identification methodologies guided by risk estimation have been designed. Two issues associated with classical risk metrics are, on the one hand, the adequacy of the metric and, on the other hand, its static nature. In this paper, we present metrics for estimating risk based on the emerging semantics provided by Formal Concept Analysis. The metrics are designed to estimate the a priori risk of compromised data deletion. Furthermore, by applying specialized variable forgetting methods for association rules, it is shown how to reflect the effect of deleting attributes belonging to potentially dangerous quasi-identifier sets. Additionally, a study of the role of the risk metric in confidence-based reasoning for re-identification is presented.
更多查看译文
关键词
Privacy,re-identification,formal concept analysis,risk metrics,association rules
AI 理解论文
溯源树
样例
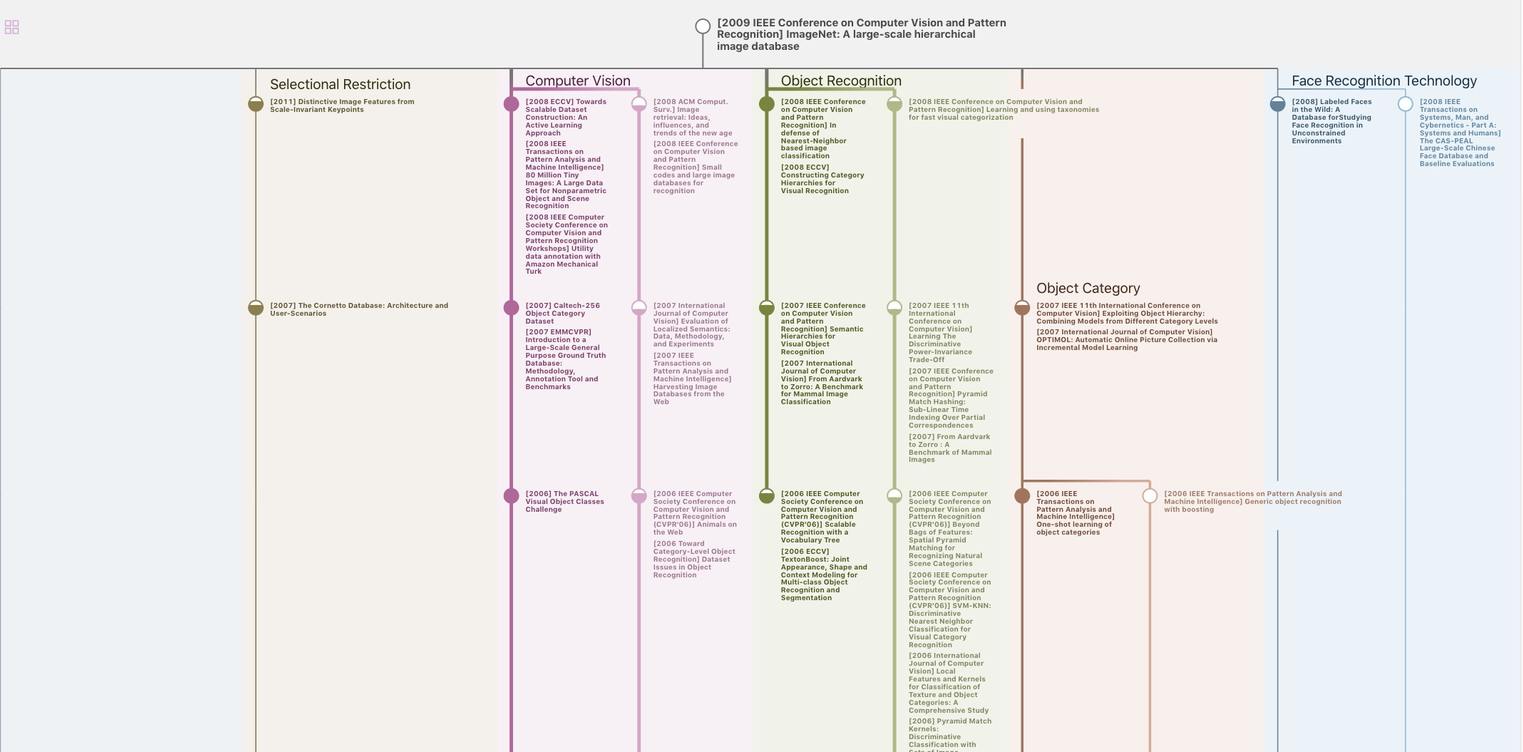
生成溯源树,研究论文发展脉络
Chat Paper
正在生成论文摘要