A simple probabilistic neural network for machine understanding
JOURNAL OF STATISTICAL MECHANICS-THEORY AND EXPERIMENT(2024)
摘要
We discuss the concept of probabilistic neural networks with a fixed internal representation being models for machine understanding. Here, 'understanding' is interpretted as the ability to map data to an already existing representation which encodes an a priori organisation of the feature space. We derive the internal representation by requiring that it satisfies the principles of maximal relevance and of maximal ignorance about how different features are combined. We show that, when hidden units are binary variables, these two principles identify a unique model-the hierarchical feature model-which is fully solvable and provides a natural interpretation in terms of features. We argue that learning machines with this architecture possess a number of interesting properties, such as the continuity of the representation with respect to changes in parameters and data, the possibility of controlling the level of compression and the ability to support functions that go beyond generalisation. We explore the behaviour of the model with extensive numerical experiments and argue that models in which the internal representation is fixed reproduce a learning modality which is qualitatively different from that of traditional models, such as restricted Boltzmann machines.
更多查看译文
关键词
learning theory,machine learning
AI 理解论文
溯源树
样例
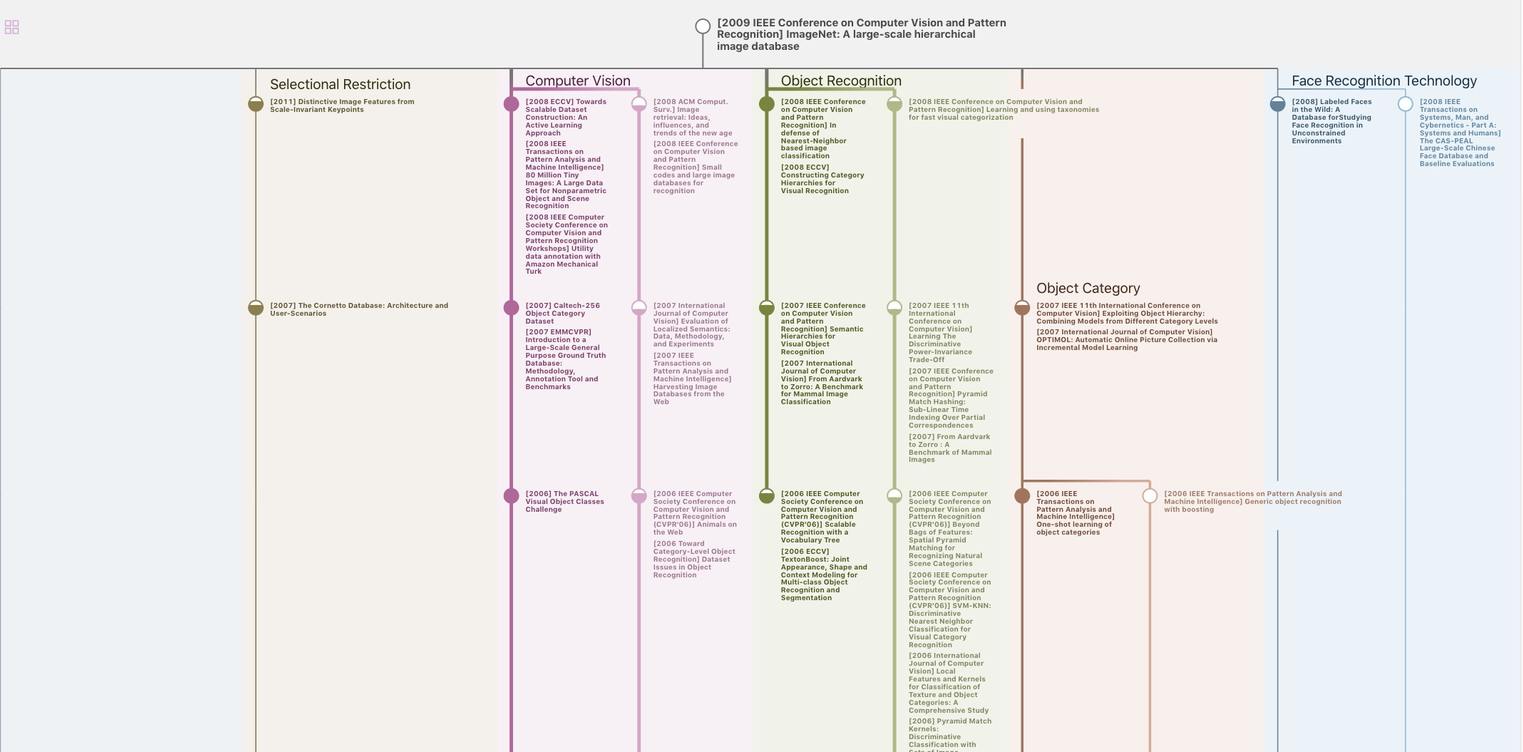
生成溯源树,研究论文发展脉络
Chat Paper
正在生成论文摘要