Machine Learning Models and Dimensionality Reduction for Prediction of Polymer Properties
MACROMOLECULES(2024)
摘要
Accurate prediction of block polymer properties as a function of monomer sequence is necessary for better material development. The number of permutations of chemistry and sequence is nearly infinite, and new methods are needed to predict and engineer properties as a function of molecular structure. In this work, we present a machine learning approach to determine polymer properties where a feed-forward neural network is trained to predict the period length of a diblock lamellar system as a function of block sequence and interaction parameters. These sequenced polymers are similar to experimentally explored polypeptoid systems. Additionally, we report on our efforts to explore dimensionality reduction as a method for gaining physical insights into these polymeric materials.
更多查看译文
AI 理解论文
溯源树
样例
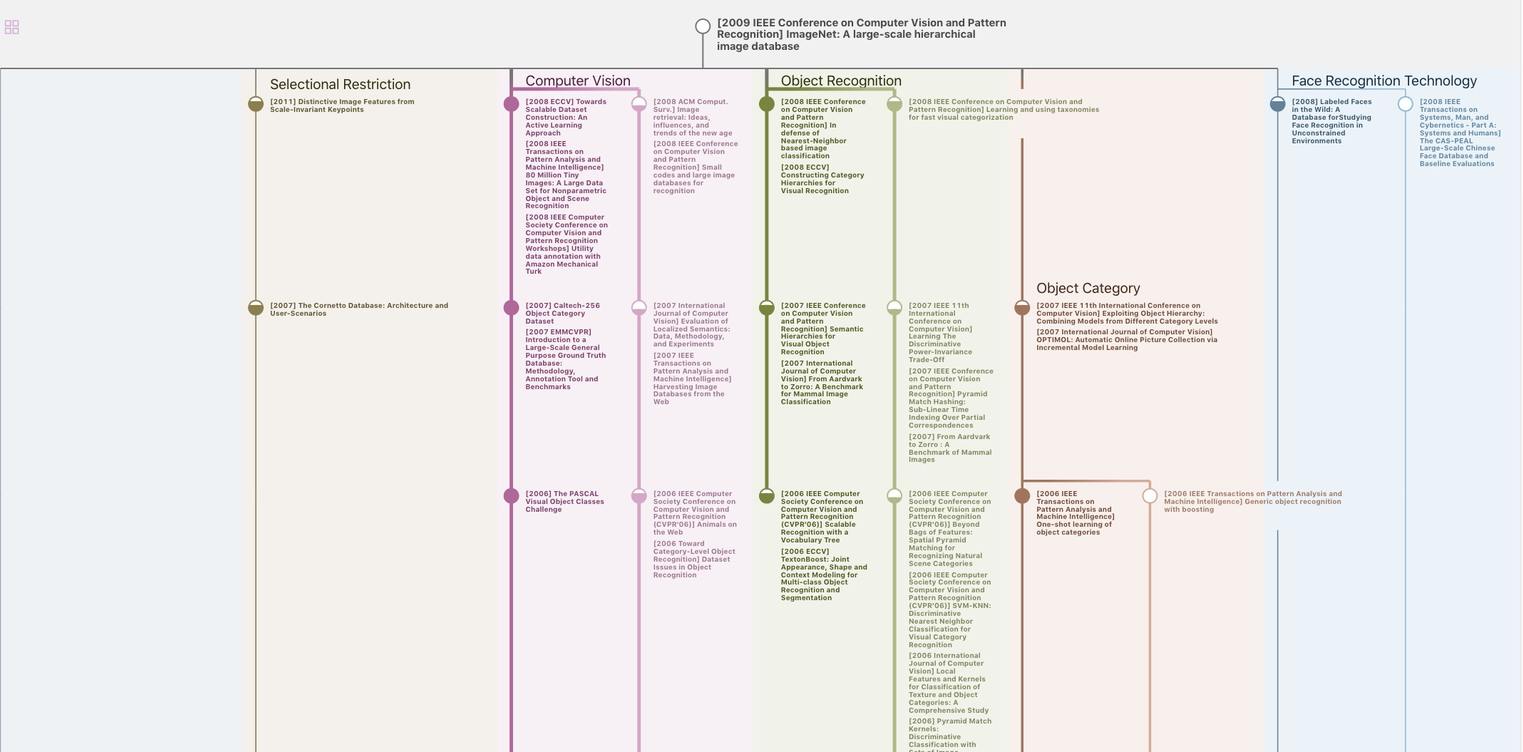
生成溯源树,研究论文发展脉络
Chat Paper
正在生成论文摘要