SCGFormer: Semantic Chebyshev Graph Convolution Transformer for 3D Human Pose Estimation
APPLIED SCIENCES-BASEL(2024)
摘要
With the rapid advancement of deep learning, 3D human pose estimation has largely freed itself from reliance on manually annotated methods. The effective utilization of joint features has become significant. Utilizing 2D human joint information to predict 3D human skeletons is of paramount importance. Effectively leveraging 2D joint data can improve the accuracy of 3D human skeleton prediction. In this paper, we propose the SCGFormer model to reduce the error in predicting human skeletal poses in three-dimensional space. The network architecture of SCGFormer encompasses Transformer and two distinct types of graph convolution, organized into two interconnected modules: SGraAttention and AcChebGconv. SGraAttention extracts global feature information from each 2D human joint, thereby augmenting local feature learning by integrating prior knowledge of human joint relationships. Simultaneously, AcChebGconv broadens the receptive field for graph structure information and constructs implicit joint relationships to aggregate more valuable adjacent features. SCGraFormer is tested on widely recognized benchmark datasets such as Human3.6M and MPI-INF-3DHP and achieves excellent results. In particular, on Human3.6M, our method achieves the best results in 9 actions (out of a total of 15 actions), with an overall average error reduction of about 1.5 points compared to state-of-the-art methods, demonstrating the excellent performance of SCGFormer.
更多查看译文
关键词
human pose estimation,graph convolution,adjacency matrix,transformer
AI 理解论文
溯源树
样例
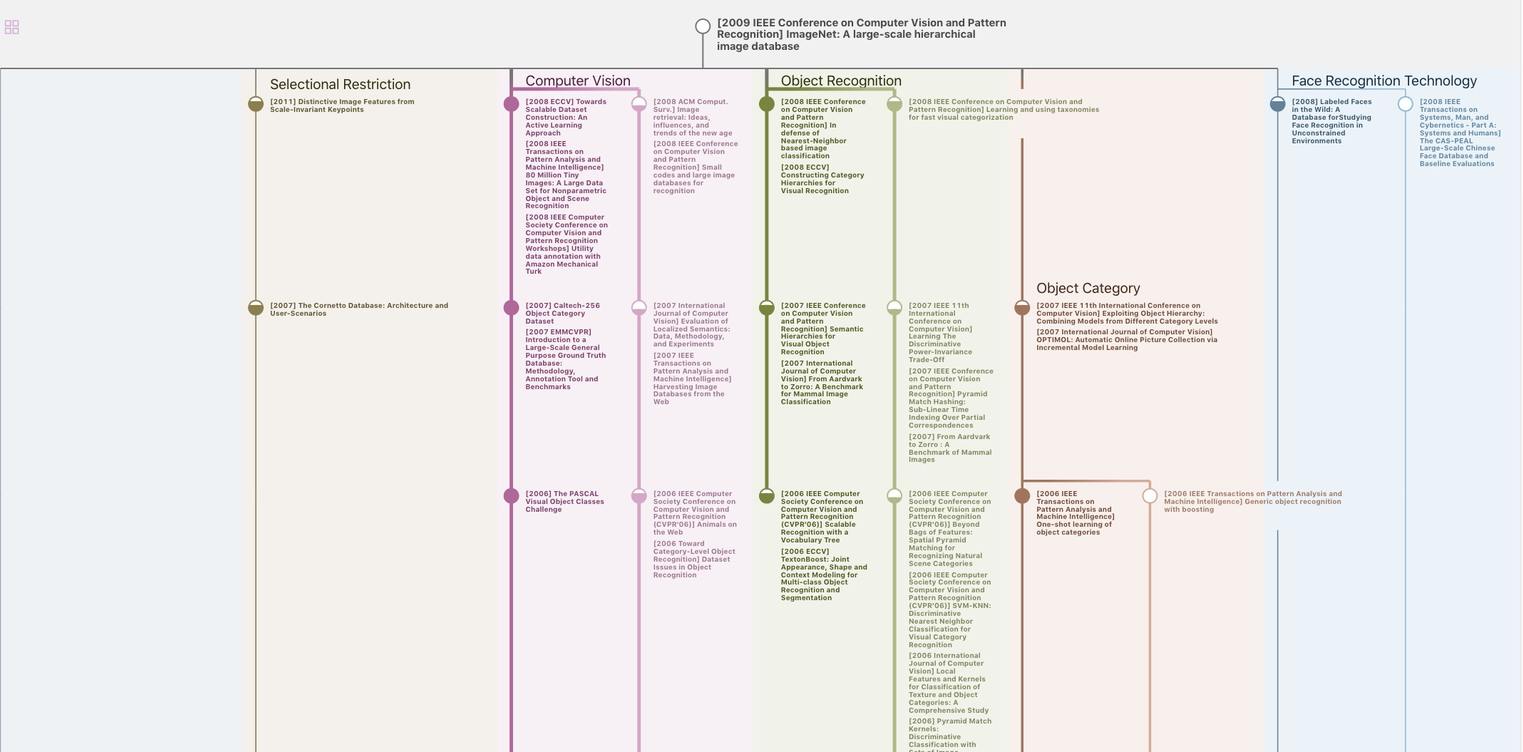
生成溯源树,研究论文发展脉络
Chat Paper
正在生成论文摘要