Dataset enhancement training of diffraction model-driven neural networks and extension to generate multi-depth computer-generated holograms
INTERNATIONAL CONFERENCE ON OPTICAL AND PHOTONIC ENGINEERING, ICOPEN 2023(2024)
摘要
Learning-based computer-generated holography (CGH) has great potential for real-time, multi-depth holographic displays. However, most existing algorithms only use the amplitude of the target image as a dataset to simplify the algorithmic process. This does not adequately consider the incorporation of the angular spectrum method (ASM) into neural networks that can compute multiplanar attributes. Here, we propose a multi-depth diffraction model-driven neural network (MD-Holo). MD-Holo utilizes pre-trained ResNet34 weights as initial parameters for the encoder phase of a complex amplitude-generating network, aiming to extract a wider range of generalized features and prevent overfitting to some extent. Motion blur, Gaussian filtering, lens blur, and low-pass filtered images are added to accommodate a wider range of images. Compared to the super-resolution DIV2K dataset alone, the use of the enhanced dataset allows both the generation of high-fidelity super-resolution images and the generalization of a wider variety of images. Simulations and optical experiments show that MD-Holo can reconstruct multi-depth images with high quality and fewer artifacts.
更多查看译文
关键词
phase-only hologram,deep learning,multi-depth,Computer-generated Holography
AI 理解论文
溯源树
样例
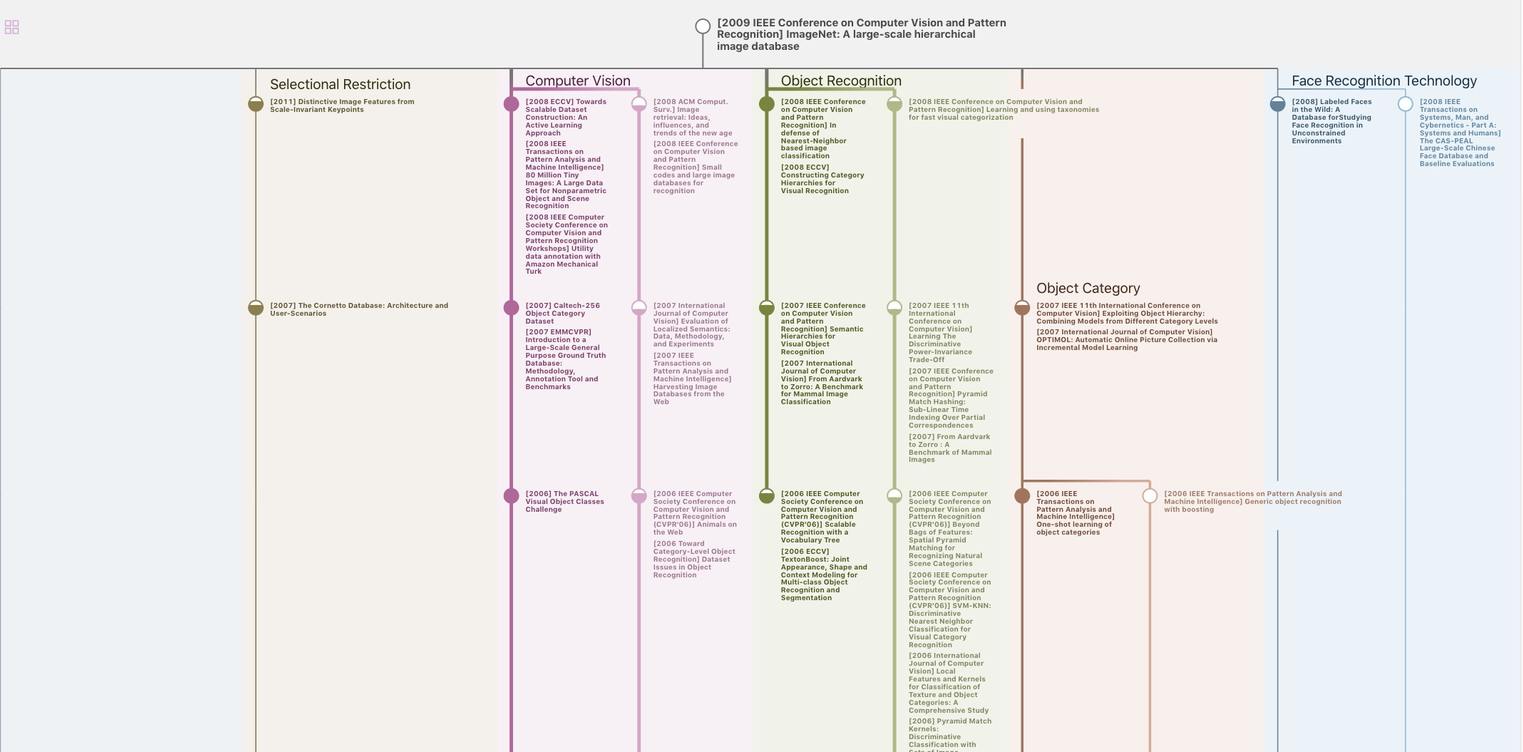
生成溯源树,研究论文发展脉络
Chat Paper
正在生成论文摘要