Modeling thermal conductivity of hydrogen-based binary gaseous mixtures using generalized regression neural network
INTERNATIONAL JOURNAL OF HYDROGEN ENERGY(2024)
摘要
The accurate measurement of the thermal conductivity of hydrogen is crucial in efficiently integrating hydrogen into diverse applications that encompass storage, utilization, and production. This paper introduces strong intelligent algorithms capable of predicting the thermal conductivity of hydrogen-based binary gaseous mixtures consisting of carbon monoxide (CO), methane (CH4), and carbon dioxide (CO2), based on the mole fractions of these components, pressure, and temperature. To this end, four advanced intelligent models, namely Radial Basis Function (RBF), Cascade Forward Neural Network (CFNN), Multilayer Perceptron (MLP), and Generalized Regression Neural Network (GRNN) were developed. After that, an analysis of graphical and statistical errors was conducted to further examine the precision of the proposed models. The findings revealed a strong alignment between the suggested models and experimental values. Additionally, among the developed models, the GRNN technique was identified as the superior forecasting approach with the (RMSE = 0.00129) and the highest value of the coefficient of determination, (R2 = 0.9987). Moreover, the sensitivity analysis of the GRNN model indicates that hydrogen composition has the highest influence on the thermal conductivity of hydrogen-based gaseous mixtures, as evidenced by the relevancy factor value of 0.881. The assessment of group errors also demonstrates that the proposed models exhibit high accuracy when confronted with higher temperatures and pressures. Ultimately, the Leverage analysis was employed to evaluate the dependability of the new paradigm, revealing that more than 97% of the data falls within the applicable range of the paradigm.
更多查看译文
关键词
Thermal conductivity,Binary gaseous mixtures,Hydrogen,Machine learning,Generalized regression neural network
AI 理解论文
溯源树
样例
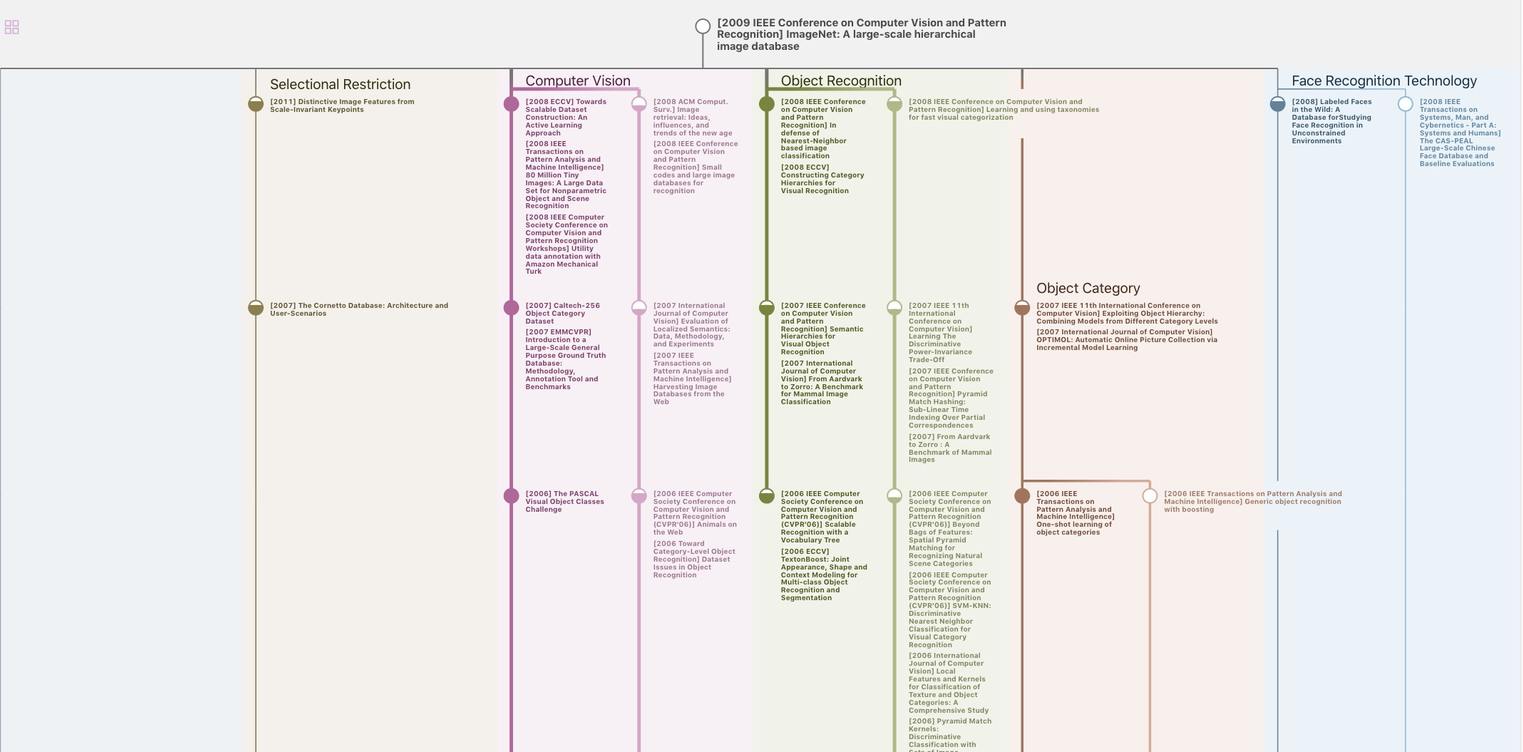
生成溯源树,研究论文发展脉络
Chat Paper
正在生成论文摘要