Forecasting-Aided Joint Topology/State Estimation for Distribution Systems via Bayesian Non-Parametric Modeling
IEEE TRANSACTIONS ON SMART GRID(2024)
摘要
To ensure the validity of distribution system state estimation (DSSE) in case of frequent topology changes, it is important to develop DSSE methods with topology identification capability. This paper proposes a forecasting-aided joint topology/state estimation method for distribution systems considering topology changes. The joint estimation problem is formulated under the Dirichlet-process hidden-Markov-model (DP-HMM), which requires establishing state-space models for a partial set of topologies (i.e., predefined topologies) and constructing probability distribution of the other possible topologies (i.e., unseen topologies). A particle filter (PF)-based method is proposed to resolve this joint estimation problem, which augments the particles to include both topology-related variables and nodal voltages. Its robustness against measurement outliers is enhanced by adopting the anti-zero bias modification. The base distribution for unseen topologies are constructed via probabilistic neural networks (PNNs). To improve the accuracy of PNNs, the synthetic minority oversampling technique is employed to rebalance the training data. Aiming at efficiency enhancement, a rule-based candidate set reduction method is proposed for the unseen topologies to improve topology sampling validity. Test results on the IEEE 33-bus, IEEE 123-bus, and a modified 2516-bus distribution systems show that the proposed method has good state and topology estimation accuracy while achieving good scalability.
更多查看译文
关键词
Active distribution system,Dirichlet-process hidden-Markov-model,forecasting-aided estimation,joint topology/state estimation
AI 理解论文
溯源树
样例
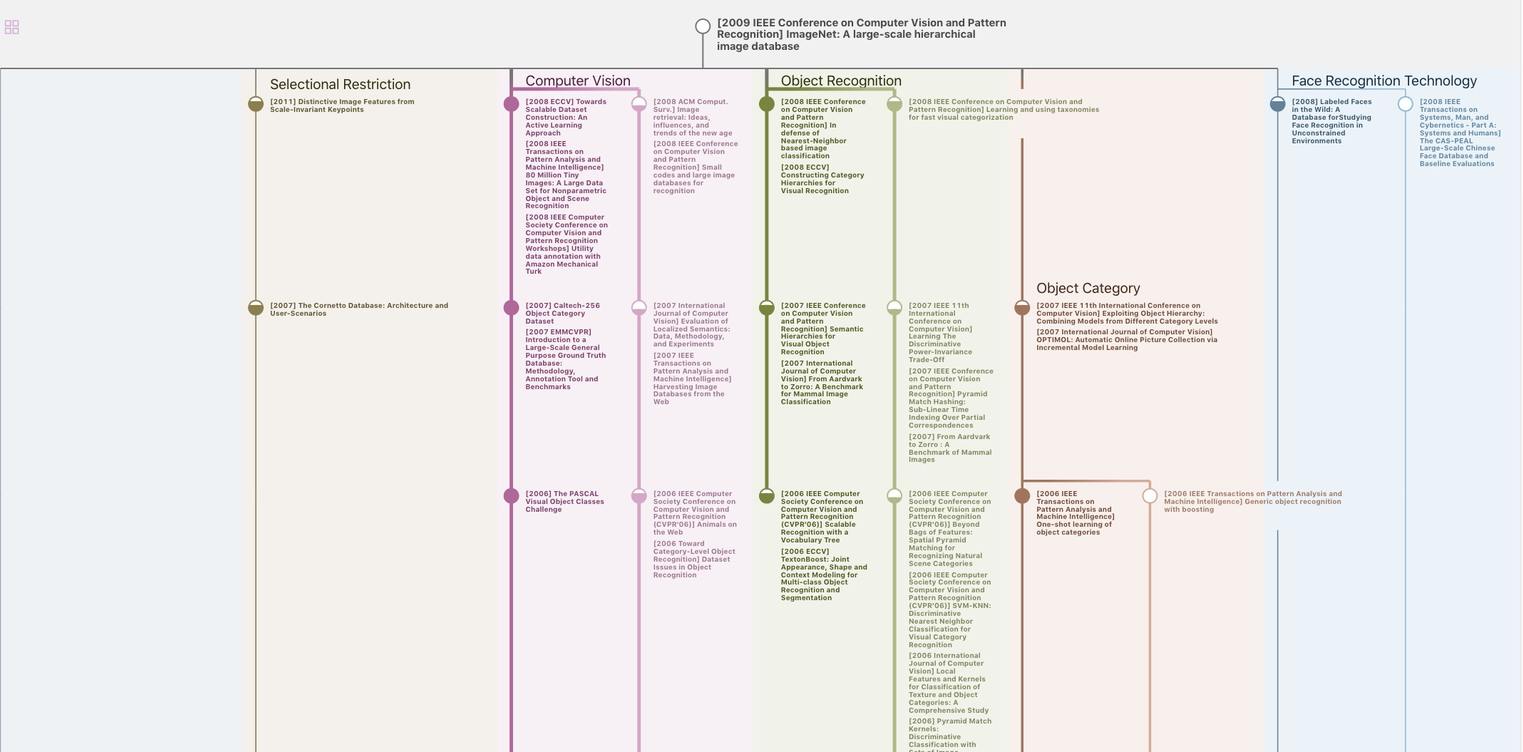
生成溯源树,研究论文发展脉络
Chat Paper
正在生成论文摘要