MCIR-CNN: Multichannel Imaging-Based Nonintrusive Load Identification With Renyi Entropy Window for Improved Sensor Data Processing
IEEE SENSORS JOURNAL(2024)
摘要
Nonintrusive load identification is an important aspect of load monitoring and can be accomplished using sensor data. In this article, we propose multichannel imaging-based methods with REW (MCIR)-convolutional neural network (CNN), a novel load identification method that leverages multichannel imaging of sensor time-series data and CNNs. We introduce a new method based on Renyi entropy for determining the sliding window size when segmenting sensor time series into subsequences, which we refer to as the Renyi entropy window (REW). Our method relies on sensor data to accurately classify loads, providing a nonintrusive solution for energy monitoring. We evaluate our method on publicly available datasets and show that MCIR-CNN outperforms the current state-of-the-art (SOTA) method, demonstrating the potential for sensors to improve household energy efficiency (EE) and reduce energy waste through load monitoring. By leveraging sensor data, our method has significant implications for the development of advanced sensor technologies in energy management.
更多查看译文
关键词
Time series analysis,Sensors,Markov processes,Entropy,Time-frequency analysis,Feature extraction,Energy efficiency,Deep learning,imaging time series,nonintrusive load monitoring (NILM),R\'{e}nyi entropy
AI 理解论文
溯源树
样例
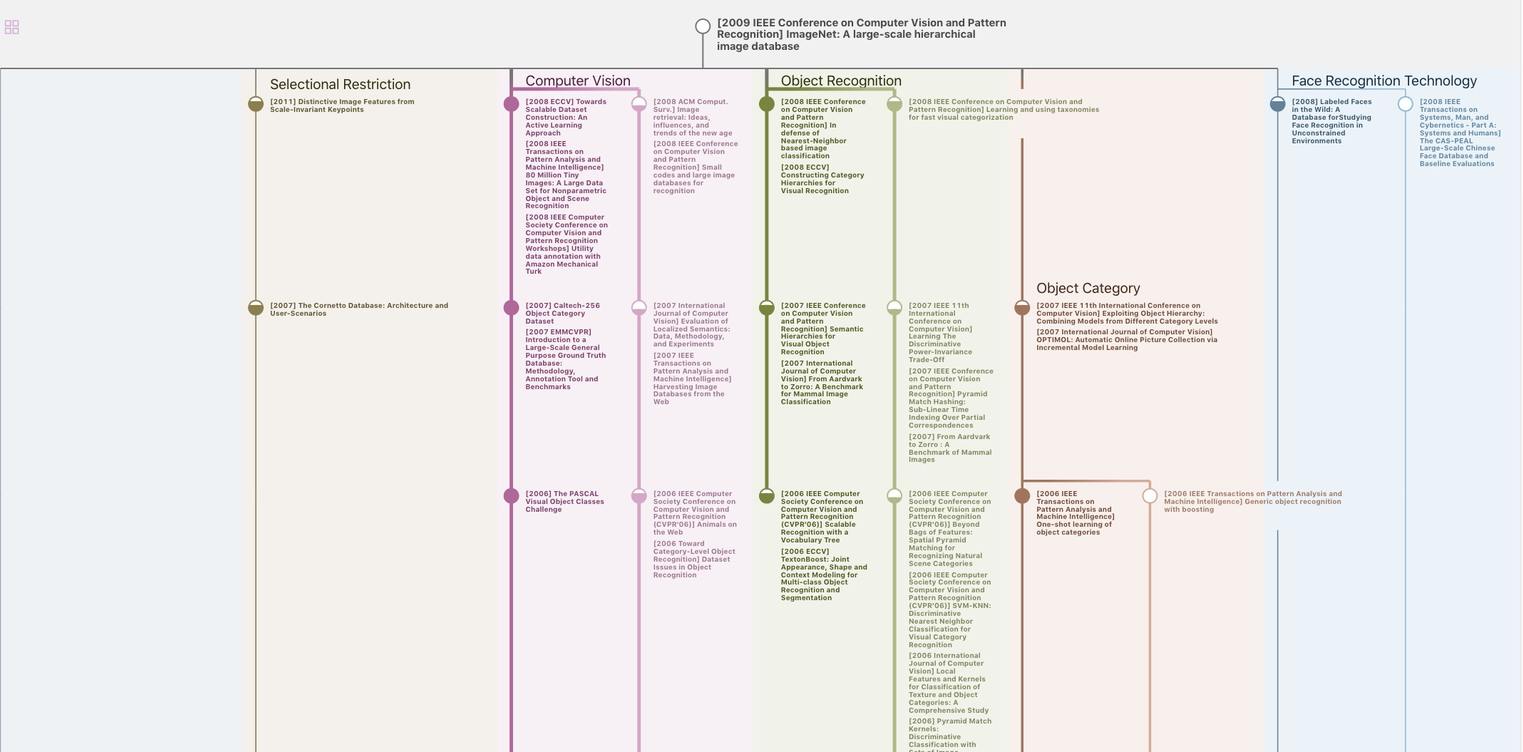
生成溯源树,研究论文发展脉络
Chat Paper
正在生成论文摘要