Control Lyapunov-barrier function-based safe reinforcement learning for nonlinear optimal control
AICHE JOURNAL(2024)
摘要
This article develops a safe reinforcement learning (SRL) algorithm for optimal control of nonlinear systems with input constraints. First, we design a novel performance index function by taking advantage of control Lyapunov-barrier functions (CLBF) with inherent safety and stability properties to ensure closed-loop stability and safety during operation under the optimal control policy. Additionally, since it is challenging to represent the CLBF-based value function as an explicit function of process states, neural networks (NNs) are used to approximate the value function using the process operational data that indicate safe and unsafe operations. Theoretical results on the stability, safety, and optimality of the SRL algorithm are developed, accounting for the approximation error of the NN-based value function. Finally, the efficacy of the proposed safe optimal control scheme is shown using an application to a chemical process example.
更多查看译文
关键词
chemical process control,control Lyapunov-barrier function,machine learning,optimal control,safe reinforcement learning
AI 理解论文
溯源树
样例
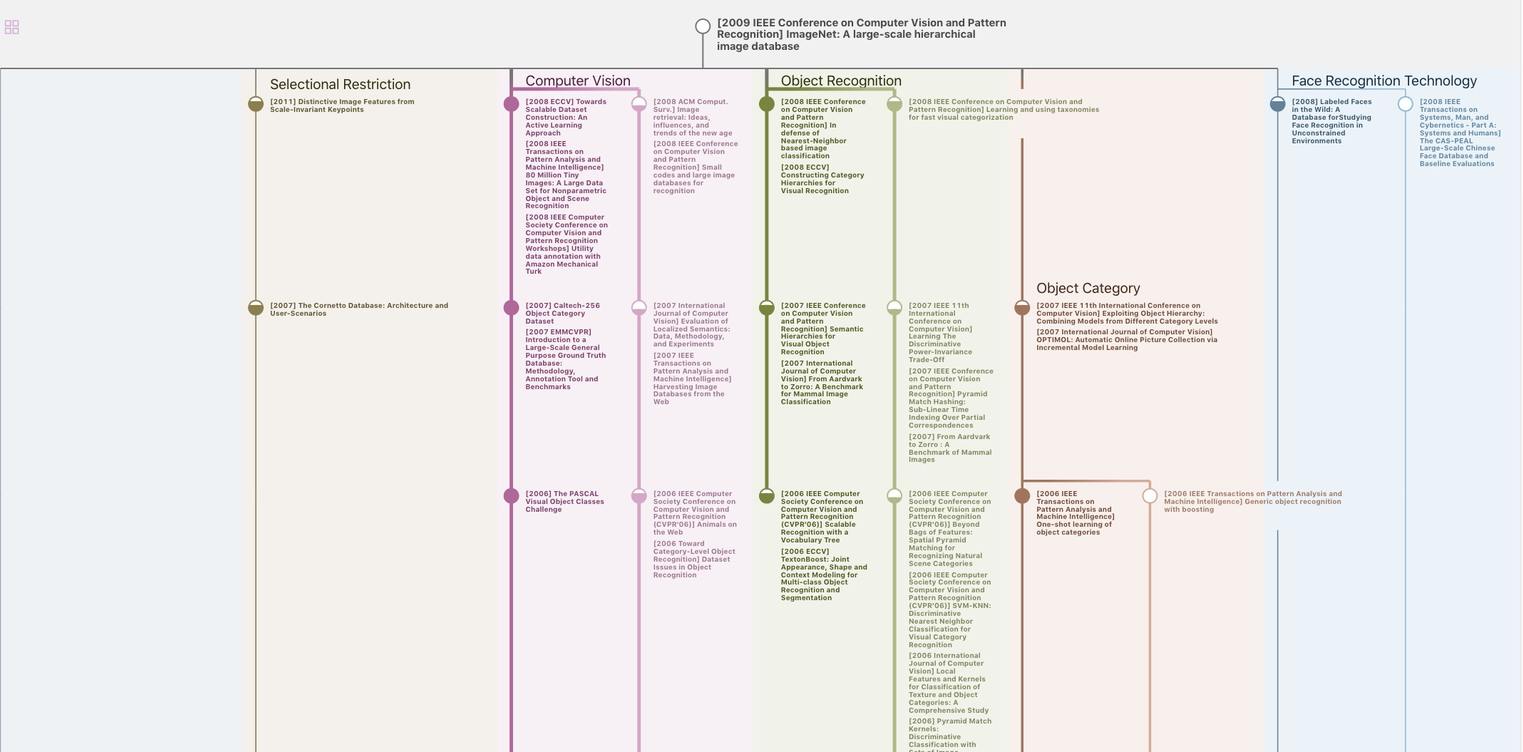
生成溯源树,研究论文发展脉络
Chat Paper
正在生成论文摘要