Practical Considerations for Sandwich Variance Estimation in 2-Stage Regression Settings
AMERICAN JOURNAL OF EPIDEMIOLOGY(2023)
摘要
In this paper, we present a practical approach for computing the sandwich variance estimator in 2-stage regression model settings. As a motivating example for 2-stage regression, we consider regression calibration, a popular approach for addressing covariate measurement error. The sandwich variance approach has rarely been applied in regression calibration, despite its requiring less computation time than popular resampling approaches for variance estimation, specifically the bootstrap. This is probably because it requires specialized statistical coding. Here we first outline the steps needed to compute the sandwich variance estimator. We then develop a convenient method of computation in R for sandwich variance estimation, which leverages standard regression model outputs and existing R functions and can be applied in the case of a simple random sample or complex survey design. We use a simulation study to compare the sandwich estimator to a resampling variance approach for both settings. Finally, we further compare these 2 variance estimation approaches in data examples from the Women's Health Initiative (1993-2005) and the Hispanic Community Health Study/Study of Latinos (2008-2011). In our simulations, the sandwich variance estimator typically had good numerical performance, but simple Wald bootstrap confidence intervals were unstable or overcovered in certain settings, particularly when there was high correlation between covariates or large measurement error.
更多查看译文
关键词
bootstrap method,measurement error,regression calibration,robust variance,sandwich variance estimation,2-stage regression
AI 理解论文
溯源树
样例
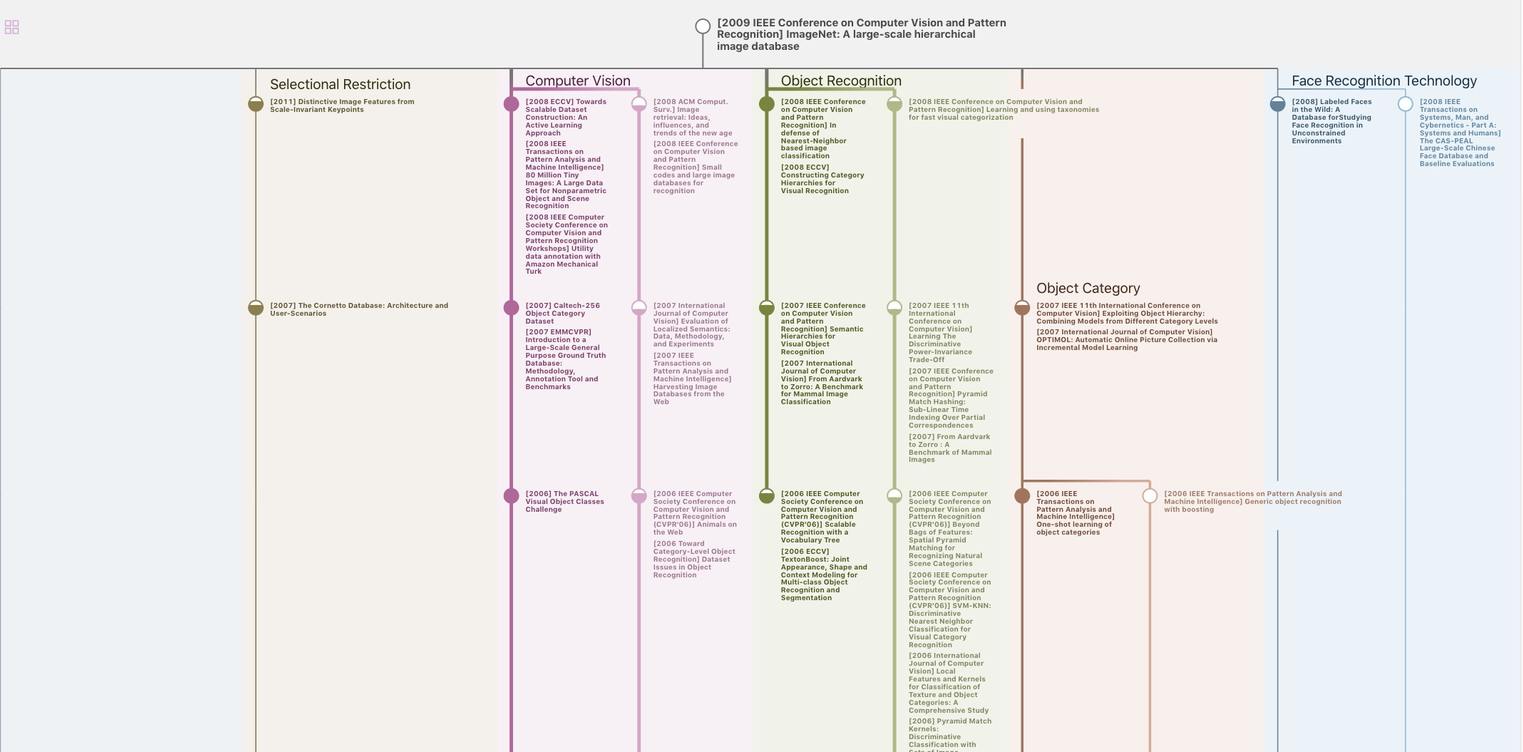
生成溯源树,研究论文发展脉络
Chat Paper
正在生成论文摘要