Private Federated Learning: An Adversarial Sanitizing Perspective
ISECURE-ISC INTERNATIONAL JOURNAL OF INFORMATION SECURITY(2023)
摘要
Large-scale data collection is challenging in alternative centralized learning as privacy concerns or prohibitive policies may rise. As a solution, Federated Learning (FL) is proposed wherein data owners, called participants, can train a common model collaboratively while their privacy is preserved. However, recent attacks, namely Membership Inference Attacks (MIA) or Poisoning Attacks (PA), can threaten the privacy and performance in FL systems. This paper develops an innovative Adversarial-Resilient Privacy-preserving Scheme (ARPS) for FL to cope with preceding threats using differential privacy and cryptography. Our experiments display that ARPS can establish a private model with high accuracy out not sign performing state-of-the-art approaches. To the best of our knowledge, this work is the only scheme providing privacy protection beyond any output models in conjunction with Byzantine resiliency without sacrificing accuracy and efficiency. (c) 2023 ISC. All rights reserved.
更多查看译文
关键词
Byzantine-resilience Differential,Privacy Federated Learning,,Homomorphic Encryption
AI 理解论文
溯源树
样例
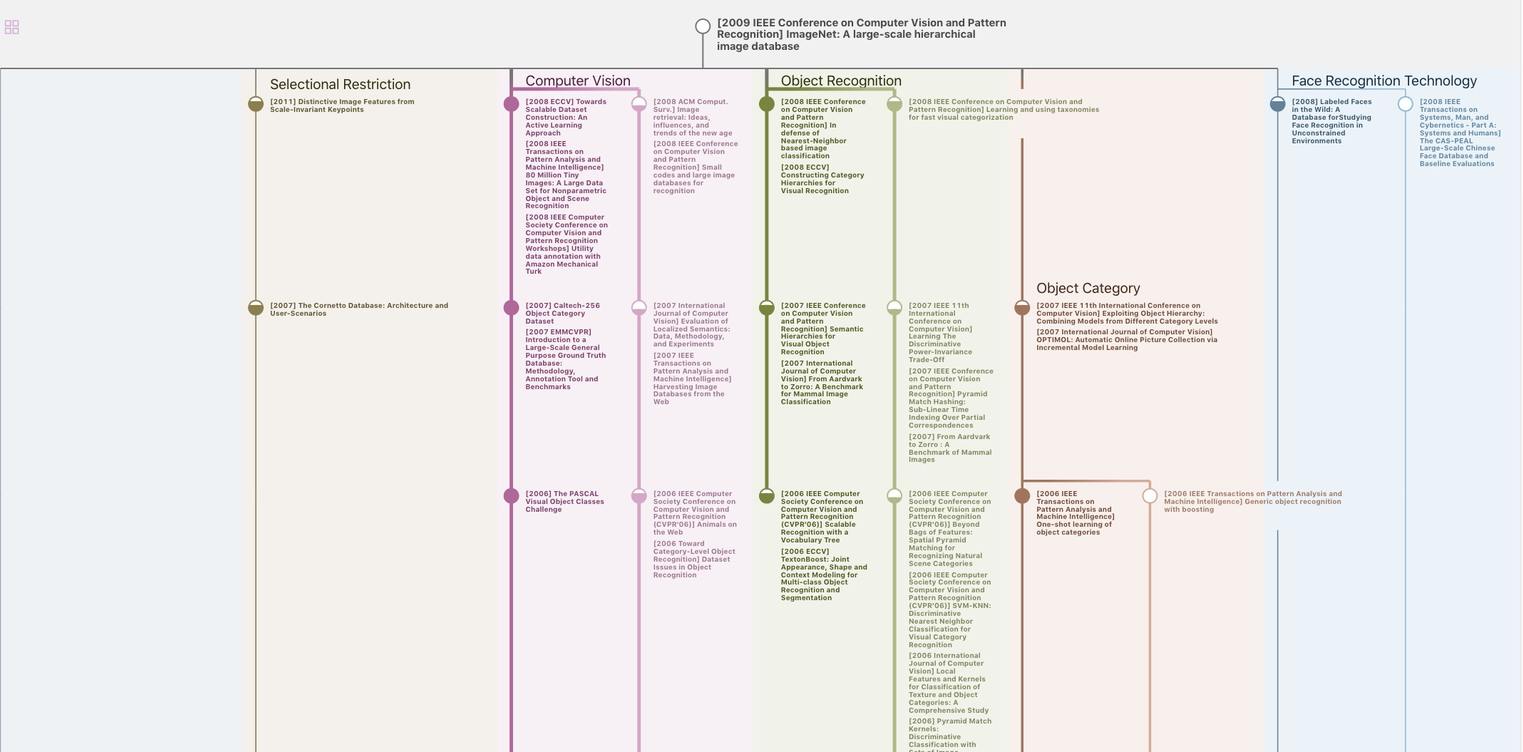
生成溯源树,研究论文发展脉络
Chat Paper
正在生成论文摘要