Sparse Bayesian Inference on Gamma-Distributed Observations Using Shape-Scale Inverse-Gamma Mixtures
BAYESIAN ANALYSIS(2024)
摘要
In various applications, we deal with high-dimensional positive-valued data that often exhibits sparsity. This paper develops a new class of continuous global-local shrinkage priors tailored to analyzing gamma-distributed observations where most of the underlying means are concentrated around a certain value. Unlike existing shrinkage priors, our new prior is a shape-scale mixture of inverse -gamma distributions, which has a desirable interpretation of the form of posterior mean and admits flexible shrinkage. We show that the proposed prior has two desirable theoretical properties; Kullback-Leibler super-efficiency under sparsity and robust shrinkage rules for large observations. We propose an efficient sampling algorithm for posterior inference. The performance of the proposed method is illustrated through simulation and two real data examples, the average length of hospital stay for COVID-19 in South Korea and adaptive variance estimation of gene expression data.
更多查看译文
关键词
gamma distribution,Kullback-Leibler super-efficiency,Markov chain Monte Carlo,tail-robustness
AI 理解论文
溯源树
样例
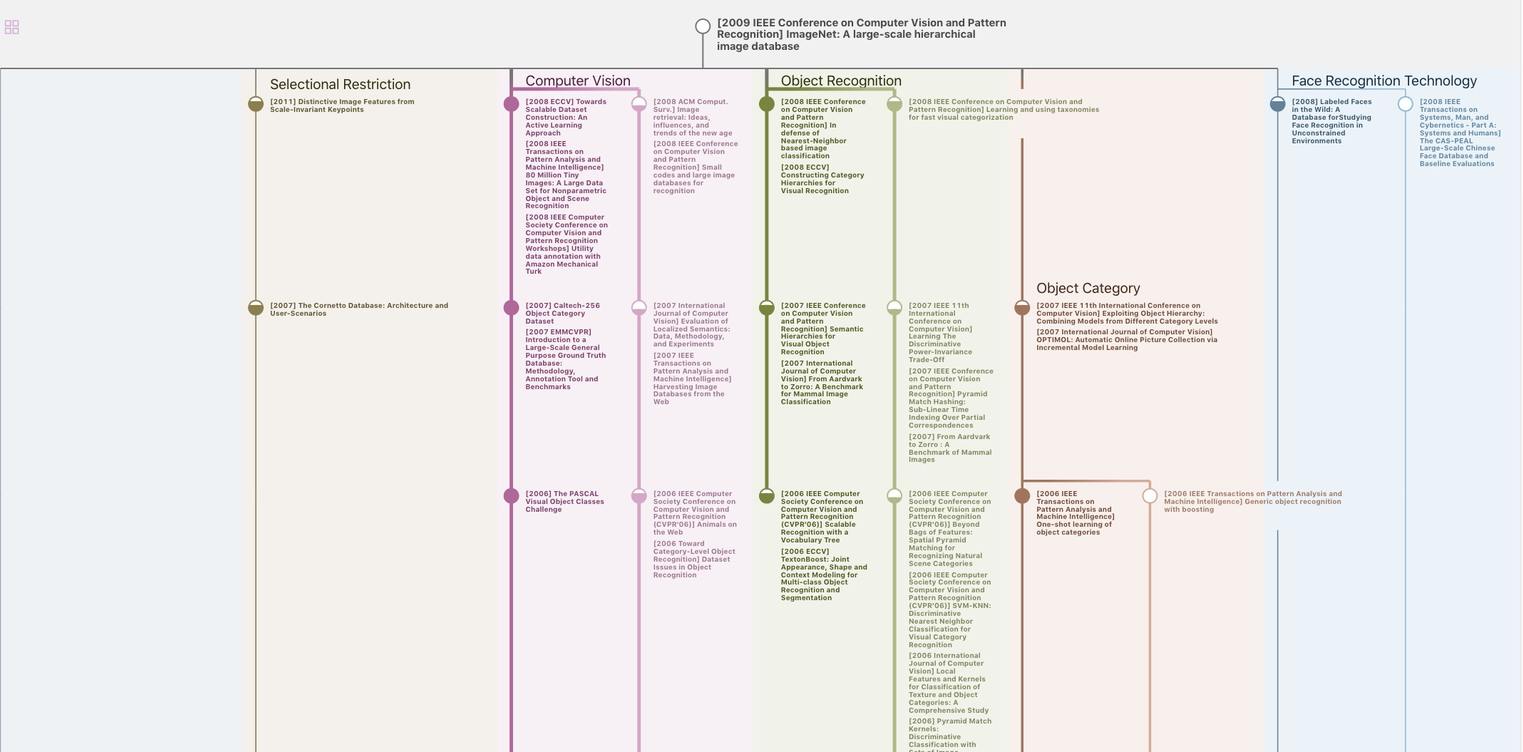
生成溯源树,研究论文发展脉络
Chat Paper
正在生成论文摘要