AD2VNCS: Adversarial Defense and Device Variation-tolerance in Memristive Crossbar-based Neuromorphic Computing Systems
ACM TRANSACTIONS ON DESIGN AUTOMATION OF ELECTRONIC SYSTEMS(2024)
摘要
In recent years, memristive crossbar-based neuromorphic computing systems (NCS) have obtained extremely high performance in neural network acceleration. However, adversarial attacks and conductance variations of memristors bring reliability challenges to NCS design. First, adversarial attacks can fool the neural network and pose a serious threat to security critical applications. However, device variations lead to degradation of the network accuracy. In this article, we propose DFS (Deep neural network Feature importance Sampling) and BFS (Bayesian neural network Feature importance Sampling) training strategies, which consist of Bayesian Neural Network (BNN) prior setting, clustering-based loss function, and feature importance sampling techniques, to simultaneously combat device variation, white-box attack, and black-box attack challenges. Experimental results clearly demonstrate that the proposed training framework can improve the NCS reliability.
更多查看译文
关键词
Neuromorphic,memristor,adversarial defense,variation tolerance
AI 理解论文
溯源树
样例
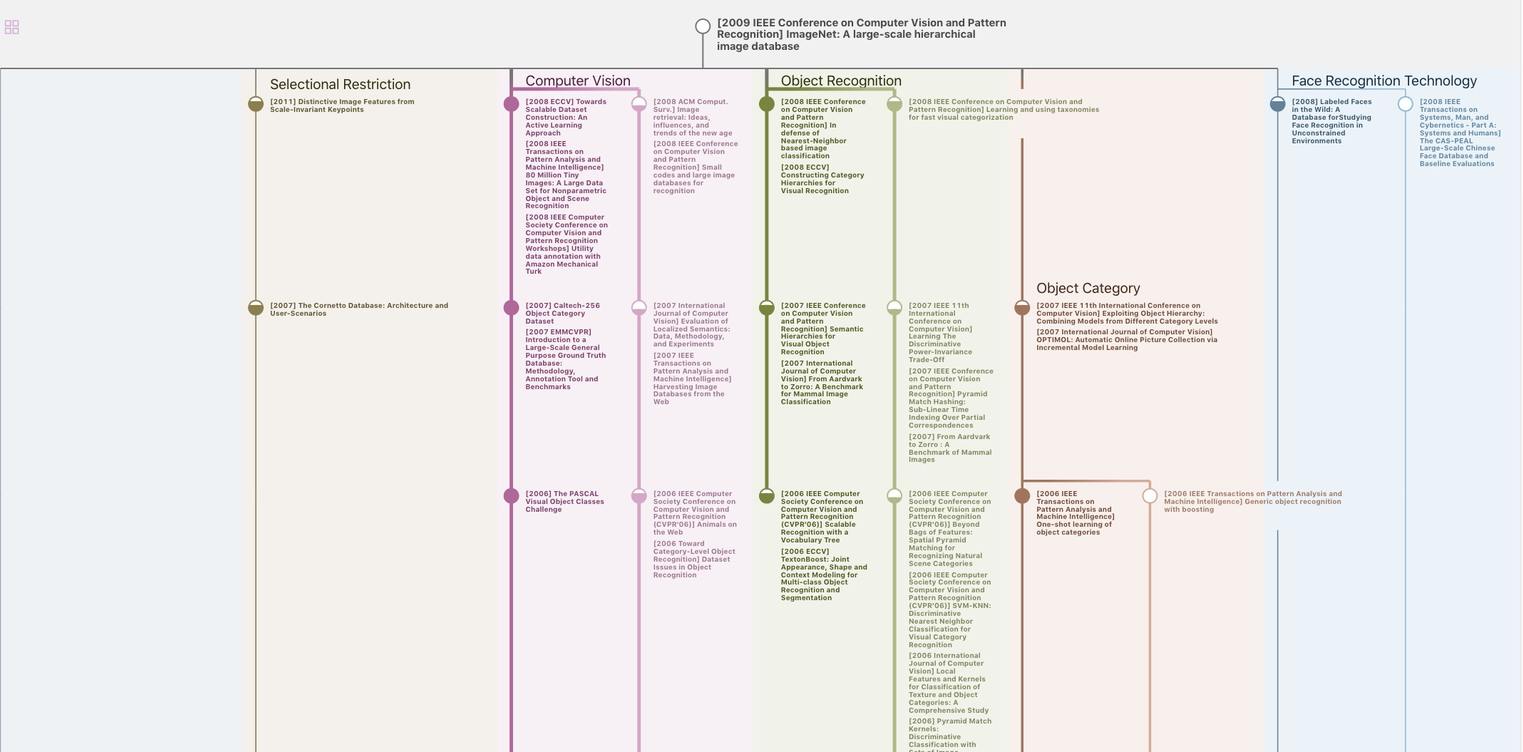
生成溯源树,研究论文发展脉络
Chat Paper
正在生成论文摘要