XGBoost algorithm-assisted prediction of amorphous formability of Fe-based nanocrystalline precursor
INTERMETALLICS(2024)
摘要
The completely amorphous structure in the precursor is a significantly important prerequisite for obtaining the perfect amorphous-nanocrystalline duplex structure and the excellent soft magnetic properties of Fe-based nanocrystalline alloy ribbons (FNR). However, the correlation between the amorphous formability (AFA) and the key parameters of FNR precursors is not clear. Herein, a data-preprocessed dataset was established, consisting of 158 FNR samples with amorphous and crystallized precursors. This dataset includes features such as elements, thermal parameters, and calculated parameters. Pearson correlation analysis was performed to assess the linear correlation between any two features, resulting in the removal of eight features. Then the features importance was ranked by random forest (RF) algorithm, and top five key features (e.g., Delta T, VEC1, VEC, P, and B) were retained. A classification predictive model in that the structure of FNR precursors is amorphous or crystalline was constructed based on XGBoost algorithm. The accuracy of the classification model by confusion matrix analysis is up to 97.9 %. Furthermore, SHAP (SHapley Additive exPlanations) results reveal that crystallization temperature intervals (Delta T), valence electron concentration without Fe, Ni, and Co elements (VEC1), as well as valence electron concentration (VEC) play an important role in affecting AFA of FNR precursors. When Delta T > 142 degrees C, VEC1 > 0.69, and VEC >7.27, the FNR precursors readily obtain a fully amorphous structure. The outstanding classification accuracy and the elucidated correlation between the key features and AFA could guide the discovery of novel fully amorphous FNR precursors.
更多查看译文
关键词
Nanocrystalline alloy,Amorphous formability,Machine learning,XGBoost
AI 理解论文
溯源树
样例
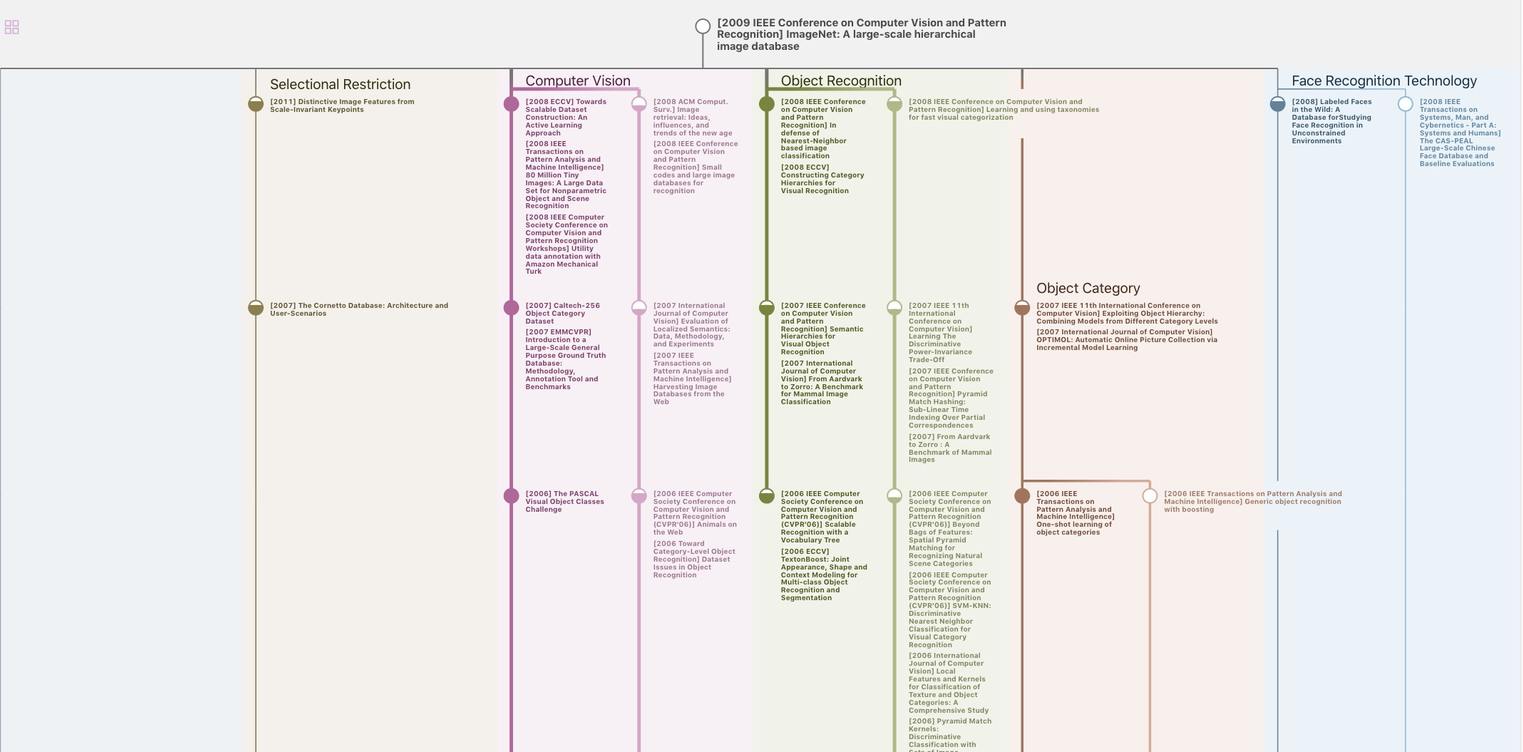
生成溯源树,研究论文发展脉络
Chat Paper
正在生成论文摘要